the Creative Commons Attribution 4.0 License.
the Creative Commons Attribution 4.0 License.
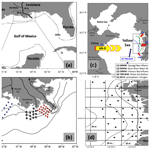
Implications of different nitrogen input sources for potential production and carbon flux estimates in the coastal Gulf of Mexico (GOM) and Korean Peninsula coastal waters
Jongsun Kim
Piers Chapman
Gilbert Rowe
Steven F. DiMarco
Daniel C. O. Thornton
The coastal Gulf of Mexico (GOM) and coastal sea off the Korean Peninsula (CSK) both suffer from human-induced eutrophication. We used a nitrogen (N) mass balance model in two different regions with different nitrogen input sources to estimate organic carbon fluxes and predict future carbon fluxes under different model scenarios. The coastal GOM receives nitrogen predominantly from the Mississippi and Atchafalaya rivers and atmospheric nitrogen deposition is only a minor component in this region. In the CSK, groundwater and atmospheric nitrogen deposition are more important controlling factors. Our model includes the fluxes of nitrogen to the ocean from the atmosphere, groundwater and rivers, based on observational and literature data, and identifies three zones (brown, green and blue waters) in the coastal GOM and CSK with different productivity and carbon fluxes. Based on our model results, the potential primary production rate in the inner (brown water) zone are over 2 gC m−2 d−1 (GOM) and 1.5 gC m−2 d−1 (CSK). In the middle (green water) zone, potential production is from 0.1 to 2 (GOM) and 0.3 to 1.5 gC m−2 d−1 (CSK). In the offshore (blue water) zone, productivity is less than 0.1 (GOM) and 0.3 (CSK) gC m−2 d−1. Through our model scenario results, overall oxygen demand in the GOM will increase approximately 21 % if we fail to reduce riverine N input, likely increasing considerably the area affected by hypoxia. Comparing the results from the USA with those from the Korean Peninsula shows the importance of considering both riverine and atmospheric inputs of nitrogen. This has direct implications for investigating how changes in energy technologies can lead to changes in the production of various atmospheric contaminants that affect air quality, climate and the health of local populations.
- Article
(4581 KB) - Full-text XML
- BibTeX
- EndNote
Industrial expansion and anthropogenic emissions are major factors leading to increased coastal productivity and potential eutrophication (Sigman and Hain, 2012). Coastal primary production is controlled largely by nitrogen (N) and phosphorus (P), and the relative supply of each determines which element limits production (Paerl, 2009); freshwater inputs and the distance from sources such as river mouths are also important (Dodds and Smith, 2016). Changes in nutrient loading from airborne, river-borne and groundwater sources can also affect which element limits coastal productivity (Sigman and Hain, 2012). Most coastal regions are N-limited; however, at certain times conditions can change from N-limited to P-limited (Dodds and Smith, 2016; Howarth and Marino, 2006). Sylvan et al. (2006), for example, suggested that the coastal Gulf of Mexico (GOM), especially near the Mississippi River delta mouth, is P-limited at certain times.
Several studies have shown that increasing atmospheric nitrogen deposition (AN-D) is contributing to ocean production globally, including to eutrophication, and is potentially of future importance in the GOM (Cornell et al., 1995; Doney et al., 2007; Duce et al., 2008; He et al., 2010; Kanakidou et al., 2016; Kim, 2018; T. W. Kim et al., 2011; Lawrence et al., 2000; Paerl et al., 2002). Recently, T. W. Kim et al. (2011), using a model simulation, showed that AN-D controls approximately 52 % of the coastal productivity in the Yellow Sea. Global NOx emissions have increased but appear to be changing differently in the USA and Asia (J. Y. Kim et al., 2010; Luo et al., 2014; Shou et al., 2018; Zhao et al., 2015), and may affect not only coastal productivity but also global total nitrogen budgets. This study uses a box model to define potential carbon fluxes based on different nitrogen input sources in two different regions, the coastal Gulf of Mexico (GOM) and the coastal sea off the Korean Peninsula (CSK). The GOM and CSK were selected in this study because while the major input source to the coastal ocean in both regions is riverine, the AN-D and submarine groundwater discharge (SGD) are considerably more important in the CSK region (Wade and Sweet, 2008; Zhao et al., 2015).
Most previous model studies in the GOM have been used to predict the size of the hypoxic zone (e.g., Fennel et al., 2006, 2011, 2013; Green et al., 2008; Hetland and DiMarco, 2008; Justic et al., 2002; Scavia et al., 2004; Turner et al., 2006, 2008), although Bierman et al. (1994) used a mass balance model to estimate carbon flux and oxygen exchange. The mass balance model is a useful tool to calculate nutrient or carbon fluxes and to estimate production in the coastal ocean (J. S. Kim et al, 2010; G. Kim et al., 2011), and such models have been successfully used in many regions and individual coastal systems to estimate ecosystem metabolism, e.g., in the Patuxent River estuary of the Chesapeake Bay (Hagy et al., 2000; Testa et al., 2008) and in the LOICZ (Land Ocean Interactions in the Coastal Zone) project (e.g., Ramesh et al., 2015). However, there are few such model studies in the GOM and CSK. All previous models for the GOM and the CSK have considered only riverine N as the predominant input source, and AN-D as an input in either region has not been considered.
In this study, we aimed to (1) build a mass balance model considering not only riverine N input but also airborne and groundwater-borne N; (2) use it to calculate potential primary production in the three regions defined by Rowe and Chapman (2002, henceforth RC02, see next section) and their associated coastal productivity; and (3) use the mass balance model to test the RC02 hypothesis. Because RC02 did not quantify their model with nutrient data, and because this model has not been applied to another region, we tested the RC02 hypothesis using data from both the GOM and the CSK that include low salinity samples. We used historical data from the mid-western part of the CSK and evaluated the theoretical model of RC02 in both areas where freshwater with high terrestrial nutrient input mixes into the coastal ocean.
The Louisiana–Texas (LATEX) shelf in the northern Gulf of Mexico is affected by coastal nutrient loading, leading to hypoxia, coming from two major terrestrial sources (the Mississippi and Atchafalaya rivers that together form the Mississippi–Atchafalaya river system, MARS). These two major rivers have different nutrient concentrations. The Gulf of Mexico (GOM) is a semi-enclosed oligotrophic sea and the MARS is the major source of nutrients and freshwater to the northern GOM (Alexander et al., 2008; Rabalais et al., 2002; Robertson and Saad, 2014). The MARS drains 41 % of the contiguous United States (Milliman and Meade, 1983) and discharges approximately 20 000 m3 s−1, or about 60 % of the total freshwater flow, (about 10.6×1011 m3 yr−1 or 3.4×104 m3 s−1) to the northern side of the GOM. The remainder comes from other US rivers, Mexico and Cuba (Nipper et al., 2004).
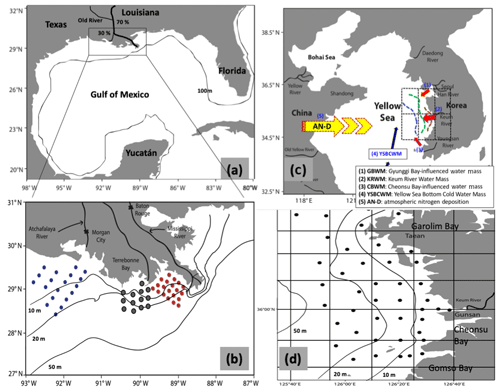
Figure 1Study sites and sampling areas in the Gulf of Mexico and the Korean Peninsula. Panel (a) shows the sampling area within the northern Gulf of Mexico. Flow in the Mississippi–Atchafalaya river system is split 30 % to the Atchafalaya River and 70 % to the Mississippi River. The box is the sampling area. Panel (b) shows station positions from March 2005. Note that MCH project data are widely distributed across the region. Red, grey and blue stations correspond to sub-regions A (near the Mississippi River), B (between the Mississippi and Atchafalaya) and C (near the Atchafalaya), respectively. Panel (c) shows the sampling area off the west coast of the Korean Peninsula. Panel (d) shows all of the station positions.
At the Old River Control Structure on the lower Mississippi River approximately 25 % of the Mississippi River's water is diverted into the Atchafalaya River, where it mixes with the water in the Red River. The flow in the Atchafalaya River totals 30 % of the total MARS flow (Fig. 1a). Several projects have investigated the relationship between nutrients and the marine ecosystem, and how this leads to hypoxia in the GOM (e.g., Bianchi et al., 2010; Diaz and Rosenberg, 1995, 2008; Forrest et al., 2011; Hetland and DiMarco, 2008; Laurent et al., 2012; Quigg et al., 2011; Rabalais and Smith, 1995; Rabalais et al., 2007; Rabalais and Turner, 2001; Rowe and Chapman, 2002). Strong stratification due to the high freshwater discharge from the MARS, local topography (DiMarco et al., 2010), wind direction and high nitrate concentration all affect hypoxia formation, with upwelling-favorable wind facilitating its development (Feng et al., 2012, 2014).
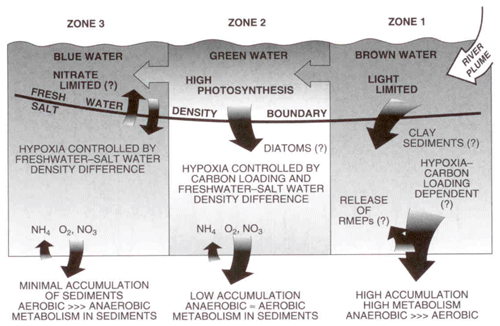
Figure 2The Rowe and Chapman three-zone hypothesis, which describes the physical and biochemical processes that initiate and sustain hypoxia on the Louisiana–Texas shelf (Rowe and Chapman, 2002). RMEPs are reduced metabolic end products. Reprinted with permission of Gulf of Mexico Science.
In the northern GOM, the major factor controlling coastal productivity is riverine N input. Rowe and Chapman (2002) defined three theoretical zones over the LATEX shelf close to the Mississippi and Atchafalaya river mouths to predict the effects of nutrient loading on hypoxia along the river plumes and over the shelf. They named these the brown, green and blue zones (Fig. 2). Nearest the river mouths is a “brown” zone, where the nutrient concentrations are high, but the discharge of sediment from the river reduces light penetration and limits primary productivity within the plume. Further away from the river plume is a stratified “green” zone with available light and nutrients that result in high productivity. In this region, the rapid depletion of nutrients is due to biological uptake processes that depend on the season and river flow (Bode and Dortch, 1996; Dortch and Whitledge, 1992; Lohrenz et al., 1999; Turner and Rabalais, 1994). Still further offshore, and also along the river plume to the west, there is the so-called “blue” zone, defined arbitrarily by nitrate concentrations of 1 µM or less, which is dominated by intense seasonal stratification and a strong pycnocline, so that in the surface layer nutrients are limiting at this distance from the rivers and most primary production is fueled by recycled nutrients (Dortch and Whitledge, 1992). It is important to note that RC02 makes clear that the edges of the zones (geographical regimes) are not static, but change over time depending on season, river flow and biological processes (Fig. 2).
The western CSK forms the eastern side of another semi-enclosed basin (the Yellow Sea) and is affected by freshwater discharge from river plumes in the same way as the coastal GOM, although the freshwater flow is considerably less. The Yellow Sea covers about 380 000 km2 area with an average water depth of 44 m, and numerous islands are located on its eastern side (Liu et al., 2003). Our specific study area is the mid-western coastal region from the Taean Peninsula to Gomso Bay (Fig. 1c and d).
There is a strong tidal front in the coastal area near the Taean Peninsula due to sea floor topography and the coastal configuration (Park, 2017; Park et al., 2017). The region also contains several bays (Garolim Bay, Gomso Bay and Cheonsu Bay), and is affected by discharges from a large artificial lake (Saemangeum Lake) as well as the freshwater discharge from the Keum River plume that contains high concentrations of nutrients (Lim et al., 2008). Conditions in the mid-western CSK near the Taean Peninsula are similar to the coastal GOM, because of mixing of two different water masses from Gyunggi Bay (Han River) and the Keum River (Choi et al., 1998, 1999). The annual mean flow rates within the Keum River were about 70 m3 s−1 (normal period) and 170 m3 s−1 (flood period; Yang and Ahn, 2008). Precipitation within the catchment was 1208 mm yr−1 during 2003 to 2005 (Yang and Ahn, 2008).
Unlike the coastal GOM, the CSK has increased nitrogen inputs from atmospheric nitrogen deposition (AN-D, which is approximately 5 times higher than in the GOM, Table 2; J. Y. Kim et al., 2010; Luo et al., 2014; Shou et al., 2018; Zhao et al., 2015) and nutrient inputs from the groundwater discharge (J. S. Kim et al., 2010; G. Kim et al., 2011). AN-D has increased in the CSK owing to industrial development in China during the last few decades, which has led to increased atmospheric N emission.
3.1 Riverine N data
Hydrographic data from the MCH (Mechanisms Controlling Hypoxia – MCH Atlas) projects in the Gulf of Mexico were collected from the National Oceanographic Data Center (https://www.nodc.noaa.gov, last access: 1 May 2017) covering the period from 2004 through 2007 (Table 1). We excluded cruises MCH M6 and M7 because the threat of hurricanes led to sampling stations in different areas from the other cruises. The study sites and sampling areas are shown in Fig. 1b. Quality control removed inconsistencies and anomalies in the data (e.g., removing outliers, missing data found by linear interpolation). Hydrographic data from the CSK (nutrients, salinity, oxygen) were collected during several cruises (Table 1 and Fig. 1c and d), and the data were put through similar QA/QC routines.
3.2 Atmospheric nitrogen deposition (AN-D) data
AN-D data from around the USA are sparse (Table 2). Most US data have been collected along the east coast of the USA, and the only data in the GOM region were collected near Corpus Christi (∼1 g m−2 yr−1; Wade and Sweet, 2008). Considerable AN-D could be expected, however, from the large number of petrochemical and fertilizer plants in southern Texas, especially near Houston and along the Mississippi. While there are more data from the Yellow Sea (J. Y. Kim et al., 2010; Luo et al., 2014; Shou et al., 2018; Zhao et al., 2015), they are still limited owing to the broad sampling coverage. While AN-D data in the Asian region were up to 14 g m−2 yr−1, data from the eastern side of the USA were under 1 g m−2 yr−1, even lower than in the GOM, suggesting there is currently not a large contribution from AN-D to total N loads in the North Atlantic Ocean. The approximate order of magnitude difference in AN-D concentrations between the GOM and the CSK is due to the continuing industrial development in East Asia and the resulting N emissions (Wang et al., 2016; Zhao et al., 2015). Lamarque et al. (2013) reported model results that cover our study regions, and their model appears to underestimate AN-D at the sampling sites compared with observational data in the GOM (Wade and Sweet, 2008). However, the pattern of AN-D inputs between GOM and CSK from Lamarque et al. (2013) shows around 5 times difference between the two regions, which agrees with our data. Thus, in our model, we used observational data for both regions, as shown in Table 2.
3.3 Methodology: N mass balance model
Our model consists of three sub-regions based on sampling locations during MCH cruises (Fig. 3), each of which contains a series of 0.25∘ square boxes, as followed by Belabbassi (2006). The 0.25∘ boxes in this study were separated into an upper box and a lower box, based on pycnocline depth, as defined by a sharp change in density and which coincides generally with a minimum change in oxygen concentration of 22.33 µM. We assume steady state conditions, and estimate potential production, which we count as an estimate of potential carbon flux (Fig. 3a). Primary production (PP) above the pycnocline is expected to be higher than below it (Anderson, 1969; Sigman and Hain, 2012), which means that the two layers have different production rates. The difference in PP between upper and lower boxes also depends on the freshwater discharge rate, which determines nutrient input to the upper layer, seasonal variability, and transfer processes between the layers. While chlorophyll can be found below the pycnocline (DiMarco and Zimmerle, 2017), the fact that it is typically associated with low oxygen concentrations suggests that the phytoplankton are either inactive or, more likely, producing at a very slow rate.
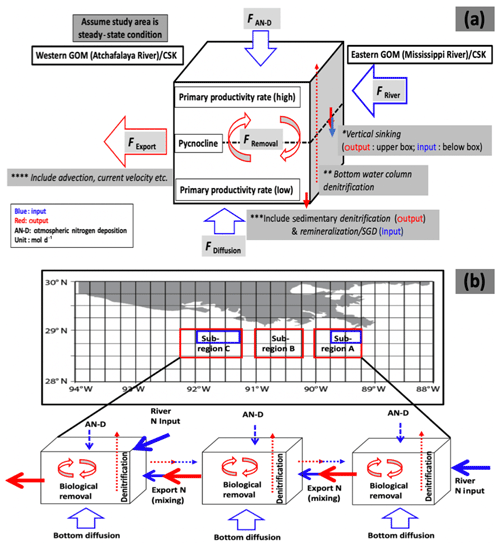
Figure 3(a) Input (blue) and output (red) sources for each 0.25∘ box in the GOM and CSK (see text for details). (b) Area of each sub-region (red) and boxes affected by direct riverine input (blue) in the GOM. Export N (mixing) represents the advective transport term. The processes of biogeochemical and transport processes of both regions are the same and each in- or output factor is the same in the GOM and CSK. Note that transfer between boxes occurs in both directions alongshore and onshore/offshore and is not a one-dimensional process as suggested in the diagram.
The N mass balance box model is modified from previous models to calculate the net removal of dissolved inorganic nitrogen (DIN) inside each box, which represents potential primary production (PPP; De Boer et al., 2010; G. Kim et al., 2011; Eq. 1). In this model, DIN concentration includes ammonium (), nitrate () and nitrite ().
where , an input term, is DIN flux from each river discharge and is calculated with , the DIN concentration in each box; ABott is the bottom area of each 0.25∘ box; and FRiver is the river discharge rate (). As another input term, is the flux from atmospheric nitrogen deposition. , the benthic flux, is an additional input term in the sub-pycnocline layer box. The 0.25∘ blue boxes located closest to the Mississippi and Atchafalaya river mouths were assumed to be the only ones affected by riverine input (Fig. 3b). As an output term, as an advection term was calculated from the current velocity in each region from observations (Nowlin et al., 1998a, b) and from literature data (Jacob et al., 2000; Lim et al., 2008) and the exchange between boxes from the residence time in each box. Note that water and nutrient exchange can take place through all four sides of each box, so the array is two-dimensional. for water mixing was calculated from these factors; is the difference in DIN concentration between adjacent boxes; VS is the water volume of each box; and λMix is the mixing rate of each box . We used a reciprocal of the water residence time that we considered to represent horizontal mixing, i.e., dispersion. Another output term is , the denitrification process from the water column, and is removal by biological production. The details of the model definitions are given below in Table 3 and shown in Fig. 3. Each arrow indicates input (blue) and output (red) terms (Fig. 3). Input/output terms vary based on whether the boxes are above/below the pycnocline, while there are separate inputs from the Mississippi and Atchafalaya rivers in the GOM and Keum and Han rivers in the CSK, respectively.
Table 3Definitions and values used in N mass balance model to calculate DIN removal by biological production.
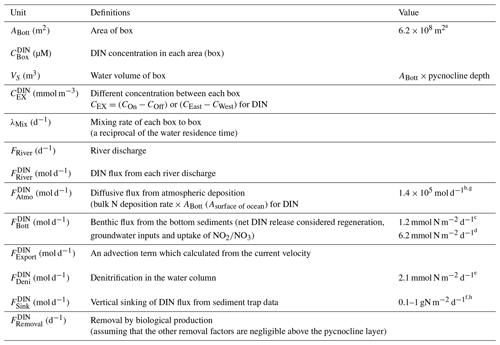
a Each 0.25∘ box. b Wade and Sweet (2008) for GOM region. c McCarthy et al. (2015). d Lee et al. (2012). e McCarthy et al. (2015). f Qureshi (1995). g of CSK region is used as mean values of Asia data in Table 2, which is initially 5 times higher than that of GOM (1.4×105 mol d−1). h The unit of was converted to mol per day from the unit of original data (gN m−2 d−1) with the area of box (0.25 m × 0.25 m) and molar mass of N (14 g mol−1). All units were converted to mol per day multiplied by the area of the box (0.25 m × 0.25 m).
In order to calculate the net removal of DIN in a box above the pycnocline layer, we used our N mass balance model in Eq. (2).
The boxes above the pycnocline layer have two input terms: (1) , riverine N, which affects only a subset of boxes along the edge of each region, and (2) , atmospheric nitrogen deposition (AN-D), which affects every box equally. The mean value of Asian data, as shown in Table 2 (J. Y. Kim et al., 2010; Luo et al., 2014; Shou et al., 2018; Zhao et al., 2015), is used for of the CSK region, which is initially 5 times higher than that of the GOM (1.4×105 mol d−1; Wade and Sweet, 2008). We also considered vertical sinking as an input for the sub-pycnocline layer box and as an output from the upper layer. Other possible input factors might be upwelling/downwelling processes; however, these factors are neglected in the model because both regions are shallow and close to shore (Feng et al., 2014; Lim et al., 2008) and we have no observational data on upwelling/downwelling rates. The output terms are the following: (1) , the exchange rate between each box (obtained from the different N concentrations in each box and the mass transfer between them), and (2) , removal by biological production, including sinking (assuming that any other removal factors are neglected above the pycnocline). We tested the RC02 three-zone hypothesis in the upper box layer, in which we can also examine the horizontal influence (horizontal extent) of the river plume based on production rates.
Below the pycnocline layer we used the revised Eq. (3).
Equation (3) has two separate input terms: (1) the benthic flux term contains all the potential input from the bottom sediment (defined here as net DIN release from the bottom sediment) including nutrient regeneration by bacteria, groundwater nutrient inputs and an uptake of nitrate () and nitrite () mainly by sedimentary denitrification (McCarthy et al., 2015; Nunnally et al., 2014), and (2) term as vertical sinking from the box above the pycnocline layer, for which we used data from Qureshi (1995). The unit of was converted to mol d−1 from the unit of original data (gN m−2 d−1) with area of box (0.25 m × 0.25 m) and molar mass of N (14 g mol−1).
In the GOM, benthic sediments provide excess ammonium to overlying water by regeneration processes such as remineralization (Lehrter et al., 2012; Nunnally et al., 2014; Rowe et al., 2002). Generally, there is an uptake of nitrate and nitrite mainly by sedimentary denitrification (McCarthy et al., 2015) or dissimilatory nitrate reduction to ammonium (DNRA) and assimilation by benthic microalgae (Christensen et al., 2000; Dalsgaard, 2003; Thornton et al., 2007). Due to this, net DIN flux was used as the value of , which shows DIN release from bottom sediments to the overlying water column. For example, in the GOM, the sums of nitrate and nitrite fluxes to bottom sediments (e.g., May: −10.05, July: −61.9, August: −48.42 µmol N m−2 h−1) were similar or smaller than the flux of ammonium from bottom sediments (e.g., May: 203, July: 152, August: 156 µmol N m−2 h−1) off Terrebonne Bay (McCarthy et al., 2015). In the CSK, the sum of the nitrate and nitrite flux to bottom sediments and ammonium flux are 0.5–1.4 mmol N m−2 d−1 and 1.3–9.6 mmol N m−2 d−1, respectively, which indicated that excess ammonium with additional nitrate and nitrite were released from sediments in this region (Lee et al., 2012). The release of nitrate and nitrite in the CSK unlike the GOM can be estimated due to high inputs of nitrogen by groundwater in the CSK (G. Kim et al., 2011) even though there is minor uptake of nitrate and nitrite. Diffusion from groundwater can probably be ignored in the GOM as Rabalais et al. (2002) reported that the groundwater discharge is very low in coastal Louisiana, but is likely important elsewhere and is known to be important in the CSK. Based on this, we averaged and sum the fluxes data of nitrate, nitrite and ammonium from McCarthy et al. (2015) for the GOM and Lee et al. (2012) for the CSK, respectively, and then applied value as 1.2 mmol N m−2 d−1 in the GOM and 6.2 mmol N m−2 d−1 in the CSK. Thus, in Eq. (3), the benthic flux term is calculated from existing literature results after considering all DIN fluxes as above (Lee et al., 2012; McCarthy et al., 2015), and then multiplied by the area of each box.
The output terms are (1) , the exchange rate between each box in the lower layer, and (2) , the denitrification rate from the water column. Due to high stratification at the pycnocline, upward transfer of dissolved material from the lower layer to the upper layer is assumed not to occur in our model. Also, denitrification from the water column below the pycnocline is a significant N removal process, which removes up to a maximum 68 % of total N input from the Mississippi River (MR) in the GOM (McCarthy et al., 2015). As the value of in the GOM, we used a direct measurement of denitrification rates from the McCarthy et al. (2015) in the water column (88 µmol m−2 h−1, which converted to 2.1 mmol N m−2 d−1) where the stations were exactly same as our sub-regions A, B and C. We assumed this applied only below the pycnocline where oxygen concentrations decrease. However, in the CSK, there is no water column denitrification data because the dissolved oxygen concentration has never been below about 4 mg L−1 during our data periods. Based on this, we estimated that there is very little water column denitrification in the CSK, so we did not count this term in the CSK. Thus, we only considered the sedimentary denitrification term for the CSK region.
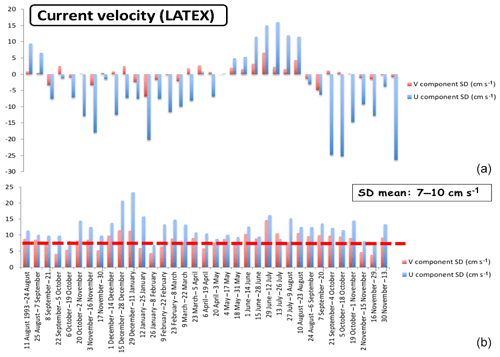
Figure 4Mean ocean current velocities (a) and standard deviations (b) for biweekly periods from August 1993 through December 1994 based on data from LATEX project. Positive values of U show eastward flow; positive values of V show northward flow.
Water transport in the region is generally from the east, i.e., from near the Mississippi River in sub-region A to the west, near the Atchafalaya River in sub-region C during non-summer periods. During summer, the winds change direction from easterly to westerly, blocking the water flow to the west (Cho et al., 1998). We calculated advection from current meter data collected during the LATEX program (Nowlin et al., 1998a, b) from April 1992 to December 1994, from which we determined U (west to east flow) and V (south to north flow) components (cm s−1). Figure 4 shows the mean values of coastal ocean current velocities. The annual range of the currents is 0 to 30 cm s−1 for the longshore component, with standard deviation of about 8 cm s−1, and 0 to 7 cm s−1 for the cross-shelf component, with a similar standard deviation, but these current velocities are not constant and change depending on time and day. The annual current velocities in the CSK are more affected by tidal exchange and the presence of the Yellow Sea Current, but velocities are similar to those in the GOM (Jacob et al., 2000; Lim et al., 2008). The annual range of the currents is around 0 to 28 and 0 to 7 cm s−1 for the cross-shelf component. Thus, we used the mean value of the current velocity for the time of year during each cruise in both the GOM and the CSK for calculating the advective flow in both alongshore and onshore/offshore directions.
To run the box model, we assumed three factors: (1) the study area is in a steady state condition, with equal input sources and outputs, (2) AN-D is evenly distributed across each area and (3) DIN is fully utilized by phytoplankton growth in the layer above the pycnocline, so we can neglect other removal factors. However, in the layer below the pycnocline, as we mentioned above, denitrification, which leads to a main loss of DIN as nitrogen gas, is considered as another output term in Eq. (3). Because we assumed that all DIN removed is fully consumed by primary production above the pycnocline, we can calculate potential carbon fluxes and oxygen consumption using the Redfield ratio (C : N :−O2 : P = 106 : 16 : 138 : 1). The PPP can be compared with 14C measurement data (Lohrenz et al., 1998, 1999; Redalje et al., 1994; Quigg et al., 2011) and dissolved oxygen data from MCH mooring C at 29∘ N, 92∘ W (4 March to 10 July 2005; Bianchi et al., 2010).
4.1 An N mass balance model for the Louisiana–Texas shelf
The existence of the three zones suggested by RC02 has been verified from winter data using nutrient/salinity relationships (Kim, 2018). Figure 5 shows the contour graph based on the mean concentration of DIN at each station during the MCH M4 (March 2005) cruise. For operational and modeling purposes, stations were grouped into three sub-regions – near the Mississippi (A), near the Atchafalaya (C) and an intermediate region (B) between ∼90 and ∼91∘ W. During summer, it is hard to use nutrient/salinity relationships directly because riverine nutrient inputs are lower and phytoplankton growth causes rapid nutrient consumption over the shelf, leading to low overall nutrient surface concentrations. We calculated the mean DIN in each box, and then used the relationship between DIN and salinity to define the edges of the three zones. Near the coast salinity was consistently low, with high turbidity from the river water discharge. This was labeled the brown (river) zone.
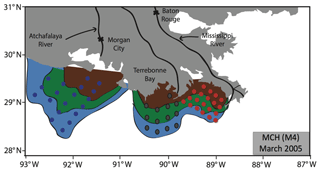
Figure 5Extent of the three zones defined by RC02 based on the mean concentration of nutrient (DIN) at each station during the MCH M4 cruise in March 2005, showing their correspondence with the three sub-regions used in the box model. Red, grey and blue stations correspond to sub-regions A (near the Mississippi River), B (between the Mississippi and Atchafalaya) and C (near the Atchafalaya), respectively.
A range of N input values from various sources were used in the N mass balance model to estimate PPP and carbon fluxes in the coastal GOM. The PPP rates were highest near the river mouth and we set the boundaries of production for each zone based on our N mass balance model results and mean DIN data. We defined the brown zone as having the PPP rate of over 2 gC m−2 d−1 because of the high input of N from the river, AN-D and benthic fluxes, and the rate in the blue zone is less than 0.1 gC m−2 d−1. The PPP rate in the green zone is then between 0.1 and 2 gC m−2 d−1. Basically, these PPP ranges were set based on synthesized measured ranges of coastal GOM primary production, as defined for near, middle, and far fields of the coastal GOM (Dagg and Breed, 2003; Lohrenz et al., 1999). Note that our model results of the PPP might overestimate the actual production because of light limitation, following RC02.
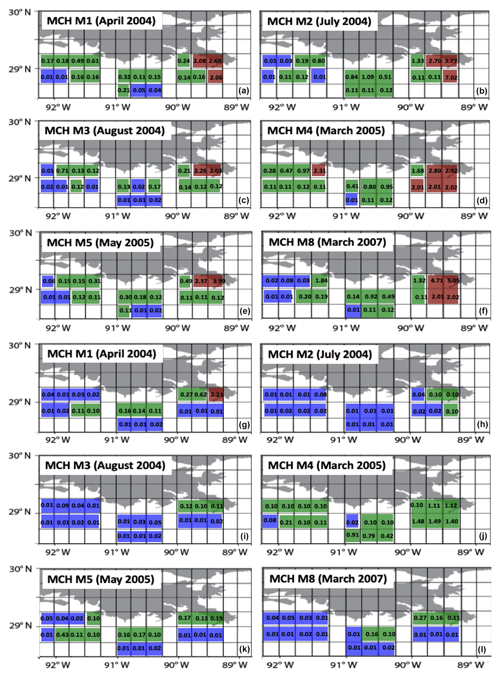
Figure 6Areal distributions of the three zones using data from above the pycnocline (a–f), based on N mass balance model results. Colors and numbers represent boxes found in each of the three zones in terms of potential productivity (unit: gC m−2 d−1). As previously, these distributions use data from below the pycnocline (g–l).
The edges of the three zones above and below the pycnocline layer, based on our N mass balance model results, are shown in Fig. 6a and b. The patterns of the boundaries above and below the pycnocline differ from the edges of the zones. The brown zone was found above the pycnocline on all cruises close to the Mississippi River mouth because of the high nutrient concentrations, but only appeared off the Atchafalaya River in March 2005 (MCH M4). However, below the pycnocline it was found only in April 2004 (MCH M1) in sub-region A. This suggests that vertical transport across the pycnocline rapidly removes the high levels of suspended material that cause light limitation above the pycnocline. In the green zones, the nutrient source is mostly supported directly by the river, with minor additional sources of N from vertical sinking, AN-D and benthic fluxes. We utilized the vertical sinking flux from the sediment trap data from Qureshi (1995) below the pycnocline layer to estimate PPP. This varied between 0.1 and 1.0 gN m−2 d−1 (Table 3). Typically, in the blue zone where biological production is low, vertical sinking followed by local decomposition is assumed to be the major factor that changes the nutrient concentration in the lower layer. The blue zone is always more extensive below the pycnocline than above it, which suggests there is little or no sub-pycnocline production except close to the coast and/or the river mouths, and reinforces the assumption that any chlorophyll below the pycnocline is inactive (Fig. 6b). Thus, we can identify the horizontal influence of the river plume in the layer below the pycnocline and the variation in the boundaries of the three zones, based on the observed nutrient data from a bottom layer and our N mass balance model. The model suggests that regions of moderate potential productivity extend offshore at least as far as 28∘30′ N in sub-region B, both above and below the pycnocline.
4.2 An N mass balance model calibration
The model calibration was done with historic literature data. The literature data suggest that observed PP rates in the green and brown zones of the coastal GOM vary between 0.4 gC m−2 d−1 (winter) and ∼8 gC m−2 d−1 (summer; Dagg et al., 2007; Lohrenz et al., 1998, 1999; Redalje et al., 1994). Recently, Quigg et al. (2011) determined the integrated PP rates with 14C measurements during 2004 in the coastal GOM. The highest integrated PP rates were found near the Mississippi River at 3.5 gC m−2 d−1 (in July), and near the Atchafalaya River at 2.7–5.9 gC m−2 d−1 (in May to July; in the brown and green zones). However, the lowest integrated PP rates were on the outer part of the LATEX shelf (the blue zone) at 0.07 gC m−2 d−1 (in March), 0.04–0.15 gC m−2 d−1 (in May) and 0.33–0.91 gC m−2 d−1 (in July). Additionally, Quigg et al. (2011) pointed out that these higher PP values were affected by high riverine nutrients input from the MR that flows westward during that time period.
The actual PP ranges were similar to our model-based PPP (Fig. 6). However, this was different from RC02's brown zone. This might be due to the differences between methods such as 14C, our N mass balance model and RC02's theoretical model. Typically, RC02 assumed that the brown zone is light-limited due to high sediment turbidity, but our model does not account for this and only considers DIN concentrations. Except for this, our PPP results are similar to direct productivity measurements from the 14C incubations (Quigg et al., 2011). Our model result (PPP) showed the same range of values as 14C incubations (e.g., Dagg et al., 2007; Lohrenz et al., 1998, 1999; Quigg et al., 2011; Redalje et al., 1994) in the three sub-regions.
Note that our model assumed all the biological uptake could be converted directly to production rates, which we considered as PPP. The PPP from cruises MCH M1–M8 for samples from above the pycnocline calculated using our model is reasonable based on comparison with previous PP values (Fig. 6a). The PPP ranges (0.01–5.05 gC m−2 d−1) were similar to previous 14C measurement PP values of between 0.04 and 5.9 gC m−2 d−1.
Based on our model calculation, which assumes all the nutrients are available for production, the PPP showed maxima at all times in sub-region A (near the Mississippi River) and minima in sub-region B (between the Mississippi and Atchafalaya rivers), except for MCH M2 in June 2004, when sub-region C had the lowest PPP (Fig. 6a). The high values in sub-region A are due largely to under-utilization of nutrients in regions of high turbidity. As the water flows west under the influence of the Coriolis effect, PPP is expected to decrease as a result of declining nutrient concentrations because of dilution and nutrient uptake during biological production while the water flows to sub-region B. In sub-region C, MCH M4 (March 2005) had the highest PPP among the all MCH cruises. This probably depended on high nutrient concentrations being present during the winter period, when the region was affected by Atchafalaya River nutrient input.
4.3 Model scenarios in the Gulf of Mexico (GOM)
We tested the sensitivity of the model to changes in input/output parameters such as increasing AN-D and decreasing riverine N input. Assuming the model is robust, we investigated three model scenarios based on the nutrient distributions seen during the MCH1 cruise (note that using data from other cruises gives very similar results). In the first scenario, we cut riverine N input 60 % and increased the AN-D input by a factor of 2 based on increasing N emission predictions (Duce et al., 2008; He et al., 2010; Kanakidou et al., 2016; T. Kim et al., 2011; Lawrence et al., 2000; Paerl et al., 2002). In the second scenario, we doubled the amount of AN-D as in scenario 1 and decreased riverine N input by 30 % based on the hypoxia management plan goal (Gulf Hypoxia Action Plan Report, 2001, 2008; Rabalais et al., 2009). In the third scenario, we increased riverine N input by 20 %, assuming the failure of the hypoxia management plan, while we set the AN-D amount equal with the first and second scenarios. Based on our N mass balance model calculation and model scenarios, we can initially estimate carbon fluxes from our PPP rate, and, using the Redfield carbon-to-oxygen stoichiometry ratio (106:138), the overall oxygen balance within the coastal GOM (Table 4).
Table 4Simulation results for selected model scenarios based on MCH M1 (5–7 April 2004), as described in the text. Biological production is calculated using our N mass balance model, while oxygen demand is calculated by the Redfield stoichiometry ratio (C: ; unit: gC m−2 d−1).
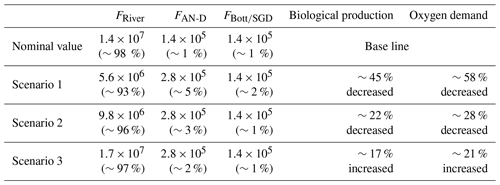
As can be seen in the scenario results for MCH M1 data (Table 4), the riverine N input source is still the major controlling factor in the coastal GOM region even when its contribution is greatly reduced and the AN-D source is doubled. For instance, if we fail to reduce riverine N input in the future (scenario 3), the potential carbon fluxes will increase by 17 % relative to current conditions. In contrast, the AN-D input source only increased to a maximum of 5 % of the total input term; this indicates that AN-D input is still a minor factor in the GOM. If the production is increased, overall oxygen demand will also be increased. The MCH M1 scenario result indicated that the overall oxygen demand would increase approximately 21 % if we fail to reduce riverine N input, likely increasing considerably the area of the hypoxia.
4.4 An N mass balance model in the CSK
As we have done in the GOM, we used our N mass balance model to estimate the PPP in the CSK and define the three different zones (Fig. 7). Similar to the GOM region, the PPP rates were highest near the river mouth, and we set the boundaries of each zone based on our N mass balance model results. Based on nutrient data, as was done for the GOM, we defined the brown zone as having a PPP rate above 1.5 gC m−2 d−1 because of the increased N sources from the river, AN-D, and the sediment flux. We defined the green zone as having PPP rates between 0.3 and 1.5 gC m−2 d−1 and the blue zone as having rates of less than 0.3 gC m−2 d−1.
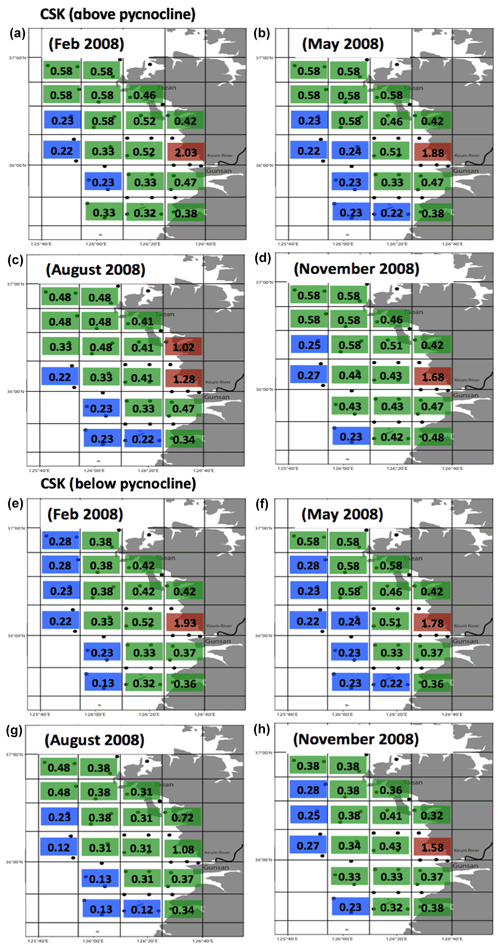
Figure 7The distribution of the three zones of the mid-western CSK above the pycnocline (a–d) based on the RC02 hypothesis applied to the N mass balance model. Colors and numbers represent boxes found in each of the three zones in terms of potential productivity (unit: gC m−2 d−1). As for above, using data from below the pycnocline (e–h).
The seasonal results shown in Fig. 7a and b show that the boundaries of the three zones above and below the pycnocline layer were roughly consistent with the main change coming in summer (August), which is the wet season and sees the highest river discharge. The large size of the green zone in all seasons suggests that AN-D is consistently adding extra nitrogen to the surface ocean along with the riverine N input. This is supported by the fact that the PPP in the blue zone is an order of magnitude higher than for the GOM. Around 90 % of the grid cells in the CSK are in the same zones above and below the pycnocline (Fig. 7a and b) during all four cruises; however, in the GOM (Fig. 6a and b) this was found for fewer than half of the grid cells. This is probably due to the difference in freshwater discharge rate in the two regions, which leads to the much larger stratified area in the GOM than in the CSK.
One question that has not been investigated is the temperature dependence of primary productivity in the two areas. While the GOM is temperate throughout the year, winter temperatures in the CSK fall to ∼5 ∘C. However, according to the ocean color remote sensing images from near the CSK river mouth reported by Son et al. (2005), primary production in the CSK does not appear to be strongly affected by temperature. The PPP results of our model (0.2 to 2.2 gC m−2 d−1) agreed with their ocean color remote sensing results (0.4 to 1.6 gC m−2 d−1) in the CSK. Also, during all seasons, the Keum River consistently supplies high amounts of DIN (average: < 60 µM; Lim et al., 2008) to the coastal zone (especially close to the Keum mouth). We believe, therefore, that the higher value of PPP in winter near the Keum mouth (brown zone in Fig. 7a), is reasonable.
The AN-D input source comes mainly from the Chinese side of the East China Sea (ECS) and this affects the boundaries of the green and blue zones above the pycnocline as it is deposited uniformly across the region. There is also nutrient input from offshore, as the Yellow Sea Bottom Cold Water Mass can up-well during the mixing process and is assumed to supply additional nutrients to the outer shelf (Lim et al., 2008).
4.5 Model scenarios in mid-western CSK
AN-D is currently considerably more important (by approximately an order of magnitude) in the CSK than in the GOM, and it is anticipated that AN-D will likely be a major controlling factor here in the future (Duce et al., 2008; He et al., 2010; T. Kim et al., 2011; Lawrence et al., 2000; Paerl et al., 2002). Because of the lack of research on potential hypoxia scenarios in the Korean Peninsula, we used the same three scenarios in the CSK as were used for the GOM. Similar to GOM results, riverine N input remains the major controlling factor; however, in this area, the AN-D source is more critical than in the GOM region (Table 5). The AN-D input source increased from 20 % to 47 % of the total input under scenario 1, while based on our scenario 3 results, increases in the AN-D input source and riverine N input together will affect biological production by increasing carbon fluxes up to 25 % and oxygen demand up to 32 % if we fail to reduce N input in future (Table 5).
Most previous model studies in the GOM were focused on predicting the hypoxia area (Bierman et al., 1994; Fennel et al., 2011, 2013; Justic et al., 1996, 2002, 2003; Scavia et al., 2004). For example, Justic et al. (1996, 2003) used a two-layer model incorporating vertical oxygen data, from one station (LUMCON station C6; 28.867∘ N, 90.483∘ W), to predict the size of the hypoxia area. Similarly, Fennel et al. (2011, 2013) used their more complex simulation model, which included oxygen concentration as well as a plankton model from Fasham et al. (1990), to predict the size of the hypoxia region in the GOM. Our N mass balance model, in contrast, uses historical data from the LATEX shelf to estimate potential carbon fluxes in the GOM, and calculate the overall oxygen demand from those carbon fluxes. While this affects the total area subject to hypoxia it does not estimate the size of the hypoxic zone.
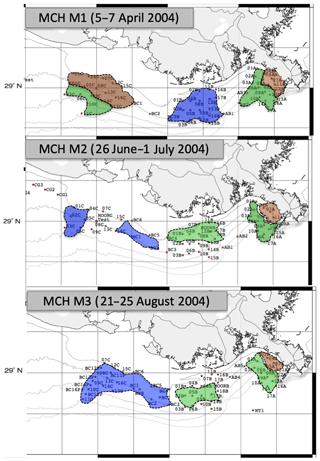
Figure 8Distribution of the three zones during cruises MCH M1–M3 based on salinity data (Lahiry, 2007). Areas identified as brown, green or blue zones are shaded accordingly.
In contrast to our model, traditional predictive models have also ignored different nitrogen input sources such as AN-D and SGD. While this is probably reasonable on the Louisiana–Texas shelf, where riverine inputs dominate, it may not apply in other coastal regions. As a result, model studies in this region have concluded that reducing riverine N input is the only solution to decrease the size of the hypoxia area in the GOM (Gulf Hypoxia Action Plan Report, 2001, 2008; Rabalais et al., 2009; Scavia et al., 2013). According to our model results, AN-D is still a minor controlling factor in the GOM; however, in the CSK, the AN-D contributed more to the total nitrogen budget and may be a major controlling factor in the future. This indicates that AN-D should be considered as another input term for nutrient managements, especially in Asia or in other regions where high concentrations are expected. Similarly, nitrogen input from either sediment fluxes or groundwater also need to be considered.
Our zonal boundaries can be compared with the results of Lahiry (2007), who used salinity to define the edges of each zone for the three cruises MCH M1, M2 and M3 (Fig. 8) and defined the edges of the RC02 zones in the coastal GOM based solely on salinity. Lahiry's limited simulation results indicated similar patterns to our model based on DIN concentration near the Mississippi River mouth (e.g., during MCH M1, M2 and M3). Mixing was more conservative in this region than further west because the low salinity water with high nutrient concentrations was less diluted with offshore water.
Away from the MR in sub-regions B and C, however, Lahiry's results gave very different boundaries for the three zones compared with our results (Fig. 8). In particular, the results near the Atchafalaya River were very different (compare Figs. 6 and 8). For example, our data showed only green and blue zones off Atchafalaya Bay during MCH M1, with no brown zone. Similarly, the extent of the blue zones in sub-region C during MCH M2 and M3 is also very different. We believe that our N-model-based classification can cover more complex biological processes than the Lahiry (2007) method, which considers only advection and mixing, and that our N-model is a more sensible way to look at biological processes in the GOM.
Our results also agree with previous studies that demonstrated that both the GOM and CSK regions are N-limited for most of the year (Lim et al., 2008; Turner and Rabalais, 2013). This compares with the results of Sylvan et al. (2007), who reported that the coastal GOM could be P-limited in the MR delta mouth area where our brown zone is located, while RC02 suggested light limitation rather than N- or P-limitation. However, these P-limited conditions appear to occur when N concentrations are very high. In particular, the N∕P ratios in the both the GOM and CSK during our sampling were less than 16, indicating that both regions were N-limited, although a few stations in the brown zone near the MR area had ratios of between 16 and 18 (Fig. 9). These higher N-to-P ratios may result from the high sediment turbidity causing light-limited conditions in this zone near the river mouth (Rowe and Chapman, 2002).
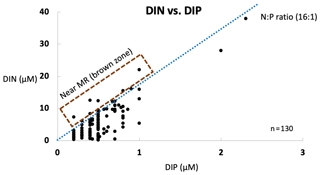
Figure 9Dissolved inorganic nitrogen (DIN) against dissolved inorganic phosphorus (DIP) during sampling periods in GOM and mid-western CSK. Nearly all samples had an N:P ratio of < 16, which indicated potential N-limited conditions. At a few points near the brown zone the ratio was between 16 and 18; this is where light limitation is expected according to RC02.
It should be remembered, however, that the arithmetic N:P value per se is unimportant in determining nutrient limitation. As long as both nutrients can be measured, it is theoretically possible for phytoplankton to continue to grow. The MARS has generally such an excess of N relative to P that N:P ratios ≫16 can be expected as P concentrations fall, but this does not necessarily mean that productivity is limited, and we never found P concentrations of zero in any of our sub-regions; the lowest P concentration measured during all cruises in the GOM and CSK was 0.2 µM.
Both the GOM and CSK regions receive nitrogen inputs from AN-D, rivers and benthic fluxes. These different nitrogen input sources control coastal productivity, and this may reflect the different nitrogen cycling in the two regions. In the GOM, the riverine N input source consistently dominates coastal productivity and eutrophication, while, in the CSK, AN-D is also becoming a critical controlling factor. In the CSK, however, there is strong tidal mixing of freshwater from the Keum River and/or Gyunggi Bay with nearby coastal water, which results in a tidal front along the offshore region and off the Taean Peninsula during spring and summer. It is this physical mixing that mostly controls the spatial distribution patterns of nutrients and salinity here, particularly below the pycnocline (Lim et al., 2008). The brown zone in the upper layer in the CSK (August 2008) changed to a green zone region below the pycnocline layer as a result of the strong coastal tidal mixing.
RC02 considered their model to be theoretical. In the brown zone, close to the river mouth, they assumed turbidity leads to light-limited conditions. Their results agree well with measured 14C PP numbers from Quigg et al. (2011), who found the lowest integrated PP is near the MR delta mouth. However, our N mass balance model did not consider light limitation and therefore PPP in the brown zone is high. Such good agreement suggests that our model can be applied to a wide region, while 14C measurements are typically conducted at a few specific points, as long as such limitations are taken into account.
In the CSK, most previous production studies focused on inshore areas such as estuaries or rivers. Our research focused for the first time on the coastal ocean off the Korean Peninsula. Our results suggest that diverse nitrogen sources need to be recognized as potential issues for future nutrient management concerned with hypoxia, eutrophication and other environmental issues. The agreement between our results and the pattern of production based on satellite-sensing in the CSK (Son et al., 2005) suggests that our model is reasonable.
The results of our changing scenarios represent how the biological processes in these coastal regions may vary as individual nutrient sources change. While our model cannot predict the area of the hypoxic zone, we can investigate the effects of potential flux changes of each factor, such as AN-D, riverine input or benthic fluxes, and calculate the effects of changes in each on PPP and on the overall oxygen balance for the region. We have only considered different input terms of our N mass balance model; output terms such as water mixing rates and the residence time for each box need more detailed study in future work to calculate more realistic production changes in each box.
The model suggests that the three-zone theory of RC02 can be applied not only in the northern GOM but also in the CSK region and that three zones can be distinguished based on their nutrient concentration. As a result, we believe that using our N mass balance model to separate different zones based on RC02 may be appropriate not only for large-scale regions like the GOM and CSK but also at small scales such as river or estuary systems. The model also estimates potential primary production and carbon flux based on the inclusion of AN-D data that have not been considered previously (e.g., Bierman et al., 1994; T. Kim et al., 2011). Our results agree well with previous 14C measurements in the GOM (Quigg et al., 2011) and ocean color remote sensing in the CSK (Son et al., 2005).
Based on CSK cruise data results, we can initially determine where the three different zones are in the CSK. We evaluated our model and tested its sensitivity based on three different scenarios. Through our scenario results, we assume that the AN-D is a considerable factor in the CSK as well as the riverine N input from the Keum River. Reducing nutrient input from the river is critical for hypoxia management policy (Gulf Hypoxia Action Plan Report, 2001, 2008; Rabalais et al., 2009). In addition, these model scenarios will be helpful for future coastal nutrient management and hypoxia management studies in the CSK, especially as AN-D sources become more important.
Hydrographic and dissolved nutrients data used in this study from the Louisiana–Texas shelf from the years 2004–2009 are available from NOAA NCEI (accession-ID 0088164).
PC and SFD were responsible for data collection. JK wrote the paper; all co-authors discussed results and assisted with writing.
The authors declare that they have no conflict of interest.
The authors would like to thank to the captains and crew of the R/V Gyre, R/V Pelican, and R/V Manta along with the many marine technicians and students who participated in the cruises.
This research was made possible by grant SA 12-09/GoMRI-006 to the Gulf Integrated Spill Consortium from the Gulf of Mexico Research Initiative and by grants NA03NOS4780039, NA06NOS4780198 and NA09NOS4780208 from NOAA.
This paper was edited by Mario Hoppema and reviewed by three anonymous referees.
Anderson, G. C.: Subsurface chlorophyll maximum in the northeast Pacific Ocean, Limnol. Oceanogr., 14, 386–391, 1969.
Alexander, R. B., Smith, R. A., Schwartz, G. E., Preston, S. D., Brakebill, J. W., Srinivasan, R., and Pacheco, A. P.: Atmospheric nitrogen flux from the watersheds of major estuaries of the United States: An application of the SPARROW watershed model, in: Coastal and Estuarine Studies-Nitrogen Loading in Coastal Water Bodies: An Atmospheric Perspective, edited by: Valigura, R. A., Alexander, R. B., Castro, M. S., Meyers, T. P., Paerl, H. W., Stacey, P. E., and Turner, R. E., American Geophysical Union, Washington, D.C., USA, 119–170, 2001.
Alexander, R. B., Smith, R. A., Schwarz, G. E., Boyer, E. W., Nolan, J. V., and Brakebill, J. W.: Differences in phosphorus and nitrogen delivery to the Gulf of Mexico from the Mississippi river basin, Environ. Sci. Technol., 42, 822–830, 2008.
Belabbassi, L.: Examination of the relationship of river water to occurrences of bottom water with reduced oxygen concentrations in the northern Gulf of Mexico, Texas A & M University, PhD dissertation., 2006.
Bianchi, T. S., DiMarco, S. F., Cowan Jr., J. H., Hetland, R. D., Chapman, P., Day, J. W., and Allison, M. A.: The Science of hypoxia in the Northern Gulf of Mexico: A review, Sci. Total Environ., 408, 1471–1484, 2010.
Bierman, V. J., Hinz, S. C., Wiseman Jr., W. J., Rabalais, N. N., and Turner, R. E.: A Preliminary Mass Balance Model of Primary Productivity and Dissolved Oxygen in the Mississippi River Plume/Inner Gulf Shelf Region, Estuaries, 17, 886–899, 1994.
Bode, A. and Dortch, Q.: Uptake and regeneration of inorganic nitrogen in coastal waters influenced by the Mississippi River: spatial and seasonal variations, J. Plankton Res., 18, 2251–2258, 1996.
Castro, M. S., Driscoll, C. T., Jordan, T. W., Reay, W. G., Boynton, W. R., Seitzinger, S. P., Styles, R. V., and Cable, J. E.: Contribution of atmospheric deposition to the total nitrogen loads to thirty-four estuaries on the Atlantic and Gulf coasts of the United States, in: Coastal and Estuarine Studies-Nitrogen Loading in Coastal Water Bodies: An Atmospheric Perspective, edited by: Valigura, R. A., Alexander, R. B., Castro, M. S., Meyers, T. P., Paerl, H. W., Stacey, P. E., Turner, R. E., American Geophysical Union, Washington, D.C., USA, 77–106, 2001.
Castro, M. S. and Driscoll, C. T.: Atmospheric nitrogen deposition to estuaries in the mid-Atlantic and northeastern United States, Environ. Sci. Technol., 36, 3242–3249, 2002.
Cho, K. R., Reid, O., and Nowlin Jr, W. D.: Objectively mapped stream function fields on the Texas-Louisana shelf based on 32 months of moored current meter data, J. Geophys. Res., 103, 10377–10390, 1998.
Choi, H. Y., Lee, S. H., and Oh, I. S.: Quantitative Analysis of the Thermal Front in the Mid-Eastern Coastal Area of the Yellow Sea, J. Korean Soc. Oceanogr., 3, 1–8, 1998.
Choi, H. Y., Lee, S. H., and Yoo, K. Y.: Salinity Distribution in the Mid-eastern Yellow Sea during the High Discharge from the Keum River Weir, J. Korean Soc. Oceanogr., 4, 1–9, 1999.
Christensen, P. B., Rysgaard, S., Sloth, N. P., Dalsgaard, T., and Schwaerter, S.: Sediment mineralization, nutrient fluxes, denitrification and dissimilatory nitrate reduction to ammonium in an estuarine fjord with sea cage trout farms, Aquat.Microbial Ecol., 21, 73–84, 2000.
Cornell, S., Rendell, A., and Jickells, T.: Atmospheric inputs of dissolved organic nitrogen to the oceans, Nature, 376, 243–246, 1995.
Dagg, M. J. and Breed, G. A.: Biological effects of Mississippi River nitrogen on the northern Gulf of Mexico-a review and synthesis, J. Mar. Syst., 43, 133–152, 2003.
Dagg, M. J., Ammerman, J. W., AMON, R. M. W., Gardner, W. S., Green, R. E., and Lohrenz, S. E.: A review of water column processes influencing hypoxia in the northern Gulf of Mexico, Estuar. Coasts, 30, 735–752, 2007.
Dalsgaard, T.: Benthic primary production and nutrient cycling in sediments with benthic microalgae and transient accumulation of macroalgae, Limnol. Oceanogr., 48, 2138–2150, 2003.
de Boer, A. M., Watson, A. J., Edwards, N. R., and Oliver, K. I. C.: A multi-variable box model approach to the soft tissue carbon pump, Clim. Past, 6, 827–841, https://doi.org/10.5194/cp-6-827-2010, 2010.
Diaz, R. J. and Rosenberg, R.: Marine benthic hypoxia: A review of its ecological effects and the behavioural responses of benthic macrofauna, Oceanogr. Mar. Biol. Ann. Rev., 33, 245–303, 1995.
Diaz, R. J. and Rosenberg, R.: Spreading dead zones and consequences for marine ecosystems, Science, 321, 926–929, 2008.
DiMarco, S. F. and Zimmerle, H. M.: MCH Atlas: Oceanographic Observations of the Mechanisms Controlling Hypoxia Project, Texas A&M University, Texas Sea Grant, College Station, TX, Publication TAMU-SG- 17-601, 350, 2017.
DiMarco, S. F., Chapman, P., Walker, N., and Hetland, R. D.: Does local topography control hypoxia of the Texas-Louisiana Shelf?, J. Mar. Syst., 80, 25–35, 2010.
Dodds, W. K. and Smith, V. H.: Nitrogen, phosphorus, and eutrophication in streams, Inland Waters., 6, 155–164, 2016.
Doney, S. C., Mahowald, N., Lima, L., Feely, R. A., Mackenzie, F. T., Lamarque, J. F., and Rasch, P. J.: Impact of anthropogenic atmospheric nitrogen and sulfur deposition on ocean acidification and the inorganic carbon system, P. Natl. Acad. Sci. USA, 104, 14580–14585, 2007.
Dortch, Q. and Whitledge, T. E.: Does nitrogen or silicon limit phytoplankton in the Mississippi River plume and nearby regions?, Cont. Shelf Res., 12, 1293–1309, 1992.
Duce, R. A., LaRoche, J., Altierl, K., Arrigo, K. R., Baker, A. R., Capone, D. G., Cornell, S., Dentener, F., Galloway, J., Ganeshram, R. S., Geider, R. J., Jickells, T., Kuypers, M. M., Langlois, R., Liss, P. S., Liu, S. M., Middelburg, J. J., Moore, C. M., Nickovic, S., Oschlies, A., Pedersen, T., Prospero, J., Schlitzer, R., Seitzinger, S., Sorensen, L. L., Uematsu, M., Ulloa, O., Voss, M., Ward, B., and Zamora, L.: Impacts of Atmospheric Anthropogenic Nitrogen on the Open Ocean, Science, 320, 893–897, 2008.
Fasham, M. J. R., Ducklow, H. W., and Mckelvie, S. M.: A nitrogen-based model of plankton dynamics in the oceanic mixed layer, J. Mar. Res., 48, 591–639, 1990.
Feng, Y., DiMarco, S. F., and Jackson, G. A.: Relative role of wind forcing and riverine nutrient input on the extent of hypoxia in the northern Gulf of Mexico, Geophys. Res. Lett., 39, L09601, https://doi.org/10.1029/2012GL051192, 2012.
Feng, Y., Fennel, K., Jackson, G. A., DiMarco, S. F., and Hetland, R. D.: A model study of the response of hypoxia to upwelling-favorable wind on the northern Gulf of Mexico shelf, J. Mar. Syst., 131, 63–73, 2014.
Fennel, K., Wilkin, J., Levin, J., Moisan, J., O'Reilly, J., and Haidvogel, D.: Nitrogen cycling in the Middle Atlantic Bight: Results from a three-dimensional model and implications for the North Atlantic nitrogen budget, Global Biogeochem. Cy., 20, GB3007, https://doi.org/10.1029/2005GB002456, 2006.
Fennel, K., Hetland, R., Feng, Y., and DiMarco, S.: A coupled physical-biological model of the Northern Gulf of Mexico shelf: model description, validation and analysis of phytoplankton variability, Biogeosciences, 8, 1881–1899, https://doi.org/10.5194/bg-8-1881-2011, 2011.
Fennel, K., Hu, J., Laurent, A., Marta-Almeida, M., and Hetland, D. R.: Sensitivity of hypoxia predictions for the northern Gulf of Mexico to sediment oxygen consumption and model nesting, J. Geophys. Res.-Oceans, 118, 990–1002, 2013.
Forrest, D. R., Hetlandl R. D., and DiMarco, S. F.: Multivariable statistical regression models of the areal extent of hypoxia over the Texas–Louisiana continental shelf, Environ. Res. Lett., 6, 045002, https://doi.org/10.1088/1748-9326/7/1/019501, 2011.
Goolsby, D. A.: Mississippi basin nitrogen flux believed to cause Gulf hypoxia, EOS Transactions, 2000, 29–321, 2000.
Green, R. E., Gould Jr., R. W., and Ko, D. S.: Statistical models for sediment/detritus and dissolved absorption coefficients in coastal waters of the northern Gulf of Mexico, Cont. Shelf Res., 28, 1273–1285, 2008.
Hagy, J. D., Sanford, L. P., and Boynton, W. R.: Estimation of net physical transport and hydraulic residence times for a coastal plain estuary using box models, Estuaries, 23, 328–340, https://doi.org/10.2307/1353325, 2000.
He, C. H., Wang, X., Liu, X., Fangmeler, A., Christie, P., and Zhang, F.: Nitrogen deposition and its contribution to nutrient inputs to intensively managed agricultural ecosystems, Ecol. Appl., 20, 80–90, 2010.
Hetland, R. D. and DiMarco, S. F.: How does the character of oxygen demand control the structure of hypoxia on the Texas-Louisiana continental shelf?, J. Mar. Syst., 70, 49–62, 2008.
Howarth, R. W. and Marino, R.: Nitrogen as the limiting nutrient for eutrophication in coastal marine ecosystems: Evolving views over three decades, Limnol. Oceanogr., 51, 364–376, 2006.
Jacob, G. A., Hur, H. B., and Riedlinger, S. K.: Yellow and East China Seas response to winds and currents, J. Geophys. Res., 105, 947–968, 2000.
Justic, D., Rabalais, N. N., and Turner, R. E.: Effects of climate change on hypoxia in coastal waters; A doubled CO2 scenario for the northern Gulf of Mexico, Limnol. Oceanogr., 41, 992–1003, 1996.
Justic, D., Rabalais, N. N., and Turner, R. E.: Modeling the impacts of decadal changes in riverine nutrient fluxes on coastal eutrophication near the Mississippi River Delta, Ecol. Model., 152, 33–46, 2002.
Justic, D., Rabalais, N. N., and Turner, R. E.: Simulated responses of the Gulf of Mexico hypoxia to variations in climate and anthropogenic nutrient loading, J. Mar. Syst., 42, 115–126, 2003.
Kanakidou, M., Myriokefalitakis, S., Daskalakis, N., and Fanourgakis, G.: Past, Present, and Future Atmospheric Nitrogen Deposition, American Meteorological Society, J. Atmos. Sci., 73, 2039–2047, 2016.
Kim, G., Kim, J. S., and Hwang, D. W.: Submarine groundwater discharge from oceanic islands standing in oligotrophic oceans: Implications for global production and organic carbon fluxes, Limnol. Oceanogr., 56, 673–682, 2011.
Kim, J. S.: Implications of different nitrogen input sources for primary production and carbon flux estimates in coastal waters, Texas A&M University, PhD dissertation, 2018.
Kim, J. S., Lee, M. J., Kim, J., and Kim, G.: Measurement of Temporal and Horizontal Variations in 222Rn Activity in Estuarine Waters for Tracing Groundwater Inputs, Ocean Sci. J., 45, 197–202, 2010.
Kim, J. Y., Ghim, Y. S., Lee, S. B., Moon, K. C., Shim, S. G., Bae, G. N., and Yoon, S. C.: Atmospheric Deposition of Nitrogen and Sulfur in the Yellow Sea Region: Significance of Long-Range Transport in East Asia, Water Air Soil Pollut., 205, 259–272, 2010.
Kim, T. W., Lee, K., Najjar, R. G., Jeong, H. D., and Jeong, H. J.: Increasing N abundance in the northwestern Pacific Ocean due to atmospheric nitrogen deposition, Science, 334, 505–509, 2011.
Lahiry, S.: Relationships between nutrients and dissolved oxygen concentrations on the Texas-Louisiana shelf during spring-summer of 2004, Texas A & M University, MS Thesis, 2007.
Lamarque, J.-F., Dentener, F., McConnell, J., Ro, C.-U., Shaw, M., Vet, R., Bergmann, D., Cameron-Smith, P., Dalsoren, S., Doherty, R., Faluvegi, G., Ghan, S. J., Josse, B., Lee, Y. H., MacKenzie, I. A., Plummer, D., Shindell, D. T., Skeie, R. B., Stevenson, D. S., Strode, S., Zeng, G., Curran, M., Dahl-Jensen, D., Das, S., Fritzsche, D., and Nolan, M.: Multi-model mean nitrogen and sulfur deposition from the Atmospheric Chemistry and Climate Model Intercomparison Project (ACCMIP): evaluation of historical and projected future changes, Atmos. Chem. Phys., 13, 7997–8018, https://doi.org/10.5194/acp-13-7997-2013, 2013.
Laurent, A., Fennel, K., Hu, J., and Hetland, R.: Simulating the effects of phosphorus limitation in the Mississippi and Atchafalaya River plumes, Biogeosciences, 9, 4707–4723, https://doi.org/10.5194/bg-9-4707-2012, 2012.
Lawrence, G. B., Goolsby, D. A., Battaglin, W. A., and Stensland, G. J.: Atmospheric nitrogen in the Mississippi River Basin-emissions, deposition and transport, Sci. Total Environ., 248, 87–100, 2000.
Lee, J. S., Kim, K. H., Shim, J. H., Han, J. H., Choi, Y. H., and Khang, B. J.: Massive sedimentation of fine sediment with organic matter and enhanced benthic-pelagic coupling by an artificial dyke in semi-enclosed Chonsu Bay, Korea, Mar. Pollut. Bull., 64, 153–163, 2012.
Lehrter, J. C., Beddick Jr., D. L., Devereux, R., Yates, D. F., and Murrell. M. C.: Sediment-water fluxes of dissolved inorganic carbon, O2, nutrients, and N2 from the hypoxic region of the Louisiana continental shelf, Biogeochemistry, 109, 233–252, 2012.
Lim, D., Kang, M. R., Jang, P. G., Kim, S. Y., Jung, H. S., Kang, Y. S., and Kang, U. S.: Water Quality Characteristics Along Mid-Western Coastal Area of Korea, Ocean Polar Res., 30, 379–399, 2008.
Liu, S. M., Zhang, J., Chen, S. Z., Chen, H. T., Hong, G. H., Wei, H., and Wu, Q. M.: Inventory of nutrient compounds in the Yellow Sea, Cont. Shelf Res., 23, 1161–1174, 2003.
Lohrenz, S. E., Wiesenburg, D. A., Arnone, R. A., and Chen, X. G.: What controls primary production in the Gulf of Mexico? in: The Gulf of Mexico Large Marine Ecosystem: Assessment, edited by: Sherman, K., Kumpf, H., and Steidinger, K., sustainability and management, Blackwell Science, Malden, MA, 151–170, 1998.
Lohrenz, S. E., Fahnenstiel, G. L., Redalje, D. G., Lang, G. A., Dagg, M. J., Whitledge, T. E., and Dortch, Q.: Nutrients, irradiance, and mixing as factors regulating primary production in coastal waters impacted by the Mississippi River plume, Cont. Shelf Res., 19, 1113–1141, 1999.
Luo, X. S., Tang, A. H., Shi, K., Wu, L. H., Li, W. Q., Shi, W. Q., Shi, X. K., Erisman, J. W., Zhang, F. S., and Liu, X. J.: Chinese coastal seas are facing heavy atmospheric nitrogen deposition, Environ. Res. Lett., 9, 1–10, 2014.
McCarthy, M. J., Newell, S. E., Carini, S. A., and Cardner, W. S.: Denitrification dominates sediment nitrogen removal and is enhanced by bottom-water hypoxia in the Northern Gulf of Mexico, Estuaries and Coasts, 38, 2279–2294, 2015.
Milliman, J. D. and Meade, R. H.: World-wide delivery of river sediment to the oceans, J. Geol., 91, 1–21, 1983.
Mississippi River/Gulf of Mexico Watershed Nutrient Task Force.: Action Plan for Reducing, Mitigating, and Controlling Hypoxia in the Northern Gulf of Mexico, Washington, D.C., 33 pp., 2001.
Mississippi River/Gulf of Mexico Watershed Nutrient Task Force.: Gulf Hypoxia Action Plan 2008 for Reducing, Mitigating, and Controlling Hypoxia in the Northern Gulf of Mexico and Improving Water Quality in the Mississippi River Basin, Washington, D.C., 61 pp., 2008.
Nipper, M., Sanchez Chavez, J. A., Tunnell Jr., J. W.: GulfBase: Resource Database for Gulf of Mexico Research: Corpus Christi, Texas A&M University, available at: http://www.gulfbase.org (last access: 1 May 2017), 2004.
Nowlin Jr., W. D., Jochens, A. E., Reid, R. O., and DiMarco, S. F.: Texas-Louisiana Shelf Circulation and Transport processes Study: Synthesis Report, Volume I: Technical Report, OCS Study MMS 98-0035, U.S. Dept. of the Interior, Minerals Mgmt Service, Gulf of Mexico OCS Region, New Orleans, LA, 502, 1998a.
Nowlin Jr., W. D., Jochens, A. E., Reid, R. O., and DiMarco, S. F.: Texas-Louisiana Shelf Circulation and Transport processes Study: Synthesis Report, Volume II: Appendices. OCS Study MMS 98-0036. U.S. Dept. of the Interior, Minerals Mgmt Service, Gulf of Mexico OCS Region, New Orleans, LA, 288, 1998b.
Nunnally, C., Quigg, A., DiMarco, S. F., Chapman, P., and Rowe, G. T.: Benthic-Pelagic Coupling in the Gulf of Mexico Hypoxic Area: Sedimentary enhancement of hypoxic conditions and near bottom primary production, Cont. Shelf Res., 85, 143–152, 2014.
Paerl, H. W.: Controlling Eutrophication along the Freshwater-Marine Continuum: Dual Nutrient (N and P) Reductions are Essential, Estuar. Coasts, 32, 593–601, 2009.
Paerl, H. W., Dennis, R. L., and Whitall, D. R.: Atmospheric Deposition of Nitrogen: Implications for Nutrient Over-Enrichment of Coastal Waters, Estuaries, 25, 677–693, 2002.
Park, M. J., Savenije, H. H. G., Cai, H., Jee, E. K., and Kim, N. H.: Progressive change of tidal wave characteristics from the eastern Yellow Sea to the Asan Bay, a strongly convergent bay in the west coast of Korea, Ocean Dynam., 67, 1137–1150, 2017.
Park, Y. H.: Analysis of characteristics of Dynamic Tidal Power on the west coast of Korea, Renew. Sustain. Energy Rev., 68, 461–474, 2017.
Quigg, A., Sylvan, J., Gustafson, A., Fisher, T., Oliver, R., Tozzi, S., and Ammerman, J.: Going west: nutrient limitation of primary production in the northern Gulf of Mexico and the importance of the Atchafalaya River, Aquat. Geochem., 17, 519–544, 2011.
Qureshi, N. A.: The role of fecal pellets in the flux of carbon to the sea floor on a river-influenced continental shelf subject to hypoxia, Louisiana State University, PhD dissertation, 1995.
Rabalais, N. N. and Smith, L. E.: The effects of bottom water hypoxia on benthic communities of the southeastern Louisiana continental shelf, New Orleans, Louisiana, U.S. Minerals Management Service, Gulf of Mexico OCS Region, 105, 1995.
Rabalais, N. N. and Turner, R. E.: Hypoxia in the Northern Gulf of Mexico: Description, causes and change, pp. 1–36, in: Coastal Hypoxia: Consequences for Living Resources and Ecosystems, edited by: Rabalais, N. N. and Turner, R. E., Coastal and Estuarine Studies, 58, 2001.
Rabalais, N. N., Turner, R. E., and Scavia, D.: Beyond science into policy: Gulf of Mexico hypoxia and the Mississippi river, Bioscience, 52, 129–142, 2002.
Rabalais, N. N., Turner, R. E., Sen Gupta, B. K., Boesch, D. F., Chapman, P., and Murrell, M. C.: Hypoxia in the northern Gulf of Mexico: Does the science support the plan to reduce, mitigate, and control hypoxia?, Estuaries Coastal, 30, 753–772, 2007.
Rabalais, N. N., Turner, R. E., Justic, D., and Diaz, R. J.: Global change and eutrophication of coastal waters, ICES, J. Mar. Sci., 66, 1528–1537, 2009.
Ramesh, R., Chen, Z., Cummins, V., Day, J., D'Elia, C., Dennison, B., Forbes, D. L., Glaeser, B., Claser, M., Clavovic, B., Kremer, H., Lange, M., Larsen, J. N., Tissier, M. L., Newton, A., Pelling, M., Purvaja, R., and Wolanski, E.: Land-ocean interactions in the coastal zone: past, present & future, Anthtropocene, 12, 85–98, 2015.
Redalje, D. G., Lohrenz, S. E., and Fahnenstiel, G. L.: The relationship between primary production and the vertical export of particulate organic matter in a river impacted coastal ecosystem, Estuaries, 17, 829–838, 1994.
Robertson, D. M. and Saad, D. A.: SPARROW Models Used to Understand Nutrient Sources in the Mississippi/Atchafalaya River Basin, J. Environ. Qual., 42, 1422–1440, 2014.
Rowe, G. T. and Chapman, P.: Continental Shelf Hypoxia: some nagging questions, Gulf Mexico Science, 20, 153–160, 2002.
Rowe, G. T., Kaegi, M. E. C., Morse, J. W., Boland, G. S., and Briones, E. G. E.: Sediment community metabolism associated with continental shelf hypoxia, northern Gulf of Mexico, Estuaries, 25, 1097–1106, 2002.
Scavia, D., Justic, D., and Bierman, V. J.: Reducing Hypoxia in the Gulf of Mexico: Advice from Three Models, Estuaries, 27, 419–425, 2004.
Scavia, D., Evans, M. A., and Obenour, D. R.: A scenario and forecast model for Gulf of Mexico hypoxic area and volume, Environ. Sci. Technol., 47, 10423–10428, 2013.
Shou, W., Zong, H., Ding, P., and Hou, L.: A modelling approach to assess the effects of atmospheric nitrogen deposition on the marine ecosystem in the Bohai Sea, China, Estuar. Coast. Shelf Sci., 208, 36–48, 2018.
Sigman, D. M. and Hain, M. P.: The Biological Productivity of the Ocean, Nat. Educat., 3, 1–16, 2012.
Son, S. H., Campbell, J. W., Dowell, M., Yoo, S. J., and Noh, J.: Primary production in the Yellow Sea determined by ocean color remote sensing, Mar. Ecol. Prog. Ser., 303, 91–103, 2005.
Sylvan, J. B., Dortch, Q., Nelson, D. M., Maier Brown, A. F., Morrison, W., and Ammerman, J. W.: Phosphorus limits phytoplankton growth on the Louisiana shelf during the period of hypoxia formation, Environ. Sci. Technol., 40, 7548–7553, 2006.
Testa, J. M., Kemp, W. M., Boynton, W. R., and Hagy, J. D.: Long-term changes in water quality and productivity in the Patuxent river estuary: 1985 to 2003, Estuar. Coasts, 31, 1021–1037, 2008.
Thornton, D. C. O., Dong, L. F., Underwood, G. J. C., and Nedwell, D. B.: Sediment-water inorganic nutrient exchange and nitrogen budgets in the Colne Estuary, UK, Mar. Ecol. Prog. Ser., 337, 63–77, 2007.
Turner, R. E. and Rabalais, N. N.: Changes in the Mississippi River nutrient supply and offshore silicate-based phytoplankton community responses, in: Changes in Fluxes in Estuaries: Implications from Science to management Proceedings of ECSA22/ERF Symposium, International Symposium Series, Olsen, Gredensborg, Denmark, 147–150, 1994.
Turner, R. E. and Rabalais, N. N.: Nitrogen and phosphorus phytoplankton growth limitation in the northern Gulf of Mexico, Aquat. Microbial Ecol., 68, 159–169, 2013.
Turner, R. E., Rabalais, N. N., and Justic, D.: Predicting summer hypoxia in the northern Gulf of Mexico: riverine N, P and Si loading, Mar. Pollut. Bull., 51, 139–148, 2006.
Turner, R. E., Rabalais, N. N., and Justic, D.: Gulf of Mexico Hypoxia: Alternate States and a Legacy, Environ. Sci. Technol., 42, 2323–2327, 2008.
Wade, T. L. and Sweet, S. T.: Final Report Coastal Bend Bays and Estuaries Program (CBBEP): Atmospheric Deposition Study, Coastal Bend Bays & Estuaries Program, Corpus Christi, Texas, USA, 48 pp., 2008.
Wang, H., Dai, M., Liu, J., Kao, S. J., Zhang, C., Cai, W. J., Wang, G., Qian, W., Zhao, M., and Sun, Z.: Eutrophication-Driven Hypoxia in the East China Sea off the Changjiang Estuary, Environ. Sci. Technol., 50, 2255–2263, 2016.
Yang, J. S. and Ahn, T. Y.: The analysis of the correlation between groundwater level and the moving average of precipitation in Kuem river watershed, J. Engineer. Geol., 18, 1–6, 2008.
Zhao, Y., Zhang, L., Pan, Y., Wang, Y., Paulot, F., and Henze, D. K.: Atmospheric nitrogen deposition to the northwestern Pacific: seasonal variation and source attribution, Atmos. Chem. Phys., 15, 10905–10924, https://doi.org/10.5194/acp-15-10905-2015, 2015.