the Creative Commons Attribution 4.0 License.
the Creative Commons Attribution 4.0 License.
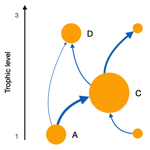
New insights into the Weddell Sea ecosystem applying a quantitative network approach
Leonardo A. Saravia
Susanne Kortsch
Network approaches can shed light on the structure and stability of complex marine communities. In recent years, such approaches have been successfully applied to study polar ecosystems, improving our knowledge on how they might respond to ongoing environmental changes. The Weddell Sea is one of the most studied marine ecosystems outside the Antarctic Peninsula in the Southern Ocean. Yet, few studies consider the known complexity of the Weddell Sea food web, which in its current form comprises 490 species and 16 041 predator–prey interactions. Here we analysed the Weddell Sea food web, focusing on the species and trophic interactions that underpin ecosystem structure and stability. We estimated the strength for each interaction in the food web, characterised species position in the food web using unweighted and weighted food web properties, and analysed species' roles with respect to the stability of the food web. We found that the distribution of the interaction strength (IS) at the food web level is asymmetric, with many weak interactions and few strong ones. We detected a positive relationship between species median IS and two unweighted properties (i.e. trophic level and the total number of interactions). We also found that only a few species possess key positions in terms of food web stability. These species are characterised by high median IS, a middle to high trophic level, a relatively high number of interactions, and middle to low trophic similarity. In this study, we integrated unweighted and weighted food web information, enabling a more complete assessment of the ecosystem structure and function of the Weddell Sea food web. Our results provide new insights, which are important for the development of effective policies and management strategies, particularly given the ongoing initiative to implement a marine protected area (MPA) in the Weddell Sea.
- Article
(3288 KB) - Full-text XML
-
Supplement
(1080 KB) - BibTeX
- EndNote
Food web analysis constitutes an important framework for understanding ecological community structure and for conserving biodiversity through ecosystem management (Thompson et al., 2012). Although topological food web analysis, which considers only the presence and absence of predator–prey interactions, provides important insights into the structure and functioning of ecological communities (e.g. Pascual et al., 2006; Kortsch et al., 2015; Marina et al., 2018; Cordone et al., 2020; Rodriguez et al., 2022), more information on the nature of the trophic interactions is needed to effectively characterise ecosystem dynamics and stability (e.g. Kortsch et al., 2021; Pecuchet et al., 2022). This is a fundamental step for providing assessments on ecosystem vulnerability to environmental pressures and for prioritising management actions. In this regard, quantifying the strength of trophic interactions and species' roles within the network are of paramount importance (Carrara et al., 2015; Allesina et al., 2015; Nilsson and McCann, 2016; Cirtwill et al., 2018).
Estimating interaction strength (IS) in food webs allows the importance of species interactions to be differentiated. On the contrary, unweighted food web representations give equal importance to all interactions despite some species interactions being stronger than others and hence playing a different role in ecosystem functioning and stability. Both empirical and theoretical studies show that interactions' strength distributions in food webs are asymmetric (Paine, 1992; McCann et al., 1998; Emmerson and Raffaelli, 2004; Wootton and Emmerson, 2005; Kortsch et al., 2021), containing a few strong and many weak interactions. This asymmetric patterning of weak and strong links is crucial to food web stability (Paine, 1992; McCann et al., 1998; Neutel et al., 2002). In a recent paper on an aquatic food web it was further highlighted that temporal changes in ecosystem functioning could only be predicted using a weighted food web structure (Kortsch et al., 2021). Hence, in order to assess the stability and functioning of a food web, it is important to first determine link weights.
Several methodologies have been applied to estimate IS in food webs, where the quantity and quality of the data mostly determine which approach is the most convenient (Berlow et al., 2004). Approaches include experimental methods combined with dynamic modelling (Emmerson and Raffaelli, 2004; Carrara et al., 2015), measurements of species abundances through time (Fahimipour and Hein, 2014; Chang et al., 2021), and estimation of metabolic rates and biomass of all species in the community (Neutel and Thorne, 2014). However, these types of methods require large experimental set-ups and parameterisations restricting the analyses to smaller networks (e.g. approximately 10 species or fewer). Other methods based on allometric scaling relationships and biomass information (Kortsch et al., 2021; Gauzens et al., 2019) can be applied to larger networks with fewer data requirements, but this comes at the expense of precision in the predictions. For even larger food webs composed of nearly 1000 species and more than 10 000 interactions, only methods with even fewer data requirements are feasible. One of these methods, proposed by Pawar et al. (2012), combines data on consumer and resource body masses and consumer search space (interaction dimensionality) to obtain IS estimates for each pairwise predator–prey interaction. An advantage of this method is that it can be applied without information on species biomass.
Diverse food webs (e.g. terrestrial, lake, marine) from various geographic locations have been studied worldwide, including marine food webs in polar regions (Carscallen and Romanuk, 2012; de Santana et al., 2013; Kortsch et al., 2019; Pecuchet et al., 2022). Studies from the Arctic show that marine food web properties (e.g. connectance and modularity) are constrained by sea ice and seawater temperature (Kortsch et al., 2019; Pecuchet et al., 2022). Likewise for the Southern Ocean it was shown that sea ice has impacts on local (Ross Sea) food web structure (Rossi et al., 2019). For example, in the Ross Sea, sea-ice breakup introduced a surge of sympagic food, which led to simpler food webs with reduced intraguild predation, potential disturbance propagation, and increased vulnerability to biodiversity loss (Rossi et al., 2019).
The Weddell Sea is expected to be one of the last regions of the Southern Ocean to experience the consequences of climate change due to its extensive sea-ice cover and ocean currents (Teschke et al., 2021) resulting in less sea surface warming compared to other areas of the Southern Ocean. Generally, the Southern Ocean plays an important role in driving global thermohaline circulation and ventilating the global abyssal ocean because it generates a considerable part of the Antarctic Bottom Water (Fahrbach et al., 2009). Because of these environmental characteristics, the Weddell Sea may serve as a refuge for Antarctic species which depend on sea ice (e.g. krill, emperor penguin, Weddell seal) or have low heat tolerance (e.g. most notothenioid fishes) due to their adaptations to freezing temperatures (Griffiths et al., 2017). While essential large-scale hydrodynamic relationships are relatively well-known for this region (de Steur et al., 2019), information on the current distribution, abundance, and sensitivity to climate change is only partially known for a few species (e.g. emperor penguin) (Houstin et al., 2022).
The Weddell Sea food web is highly complex, comprising 488 species and 16 200 predator–prey interactions (Jacob et al., 2011). To better understand species roles related to food web stability, Jacob et al. (2011) performed secondary extinctions and found that the removal of small- to medium-sized, and not large, organisms caused a cascade of secondary extinctions. These findings highlighted the relative importance of predators, rather than prey, for the architecture, functioning, and stability of the Weddell Sea food web, which coincides with findings from recent meta-analyses in empirical complex food webs (Brose et al., 2019; Perkins et al., 2022). Brose et al. (2019) considered this food web in a meta-analysis context, showing that high predator–prey body-mass ratios are found for predator groups with specific trait combinations, including small vertebrates and large swimming or flying predators. These trait combinations generate weak interactions that stabilise communities against perturbations maintaining ecosystem functioning.
In this study, we aim to go beyond a purely topological (presence/absence) assessment of who eats whom in the Weddell Sea ecosystem by providing a quantitative analysis of the trophic network structure. We aim to analyse the species' role in the structure and stability of the food web. To achieve this, we (1) estimated the strength for each interaction, (2) characterised species' roles considering both weighted and unweighted properties, and (3) analysed species' roles related to the stability of the food web.
2.1 Study area
The high Antarctic Weddell Sea shelf, our study area, is situated between 74 and 78∘ S, stretching approximately 450 km from east to west (Fig. 1). Water depth varies between 200 and 500 m, and shallower areas are covered by continental ice, which forms the coastline along the eastern and southern parts of the Weddell Sea. The shelf area contains a complex three-dimensional benthic habitat with large benthic biomasses, intermediate to high diversity in comparison to benthic boreal communities, and a spatially patchy distribution of organisms (Dayton, 1990; Teixidó et al., 2002).
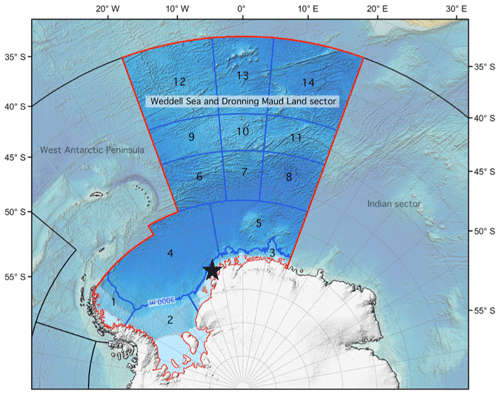
Figure 1Map of the Weddell Sea and Dronning Maud Land sector indicating the approximate location of the study area (black star, 74–78∘ S) within the high Antarctic shelf. Modified from https://www.soos.aq (last access: 10 November 2023).
2.2 Weddell Sea food web dataset
The Weddell Sea food web was retrieved from the GlobAL daTabasE of traits and food Web Architecture (GATEWAy; version 1.0) of the German Centre for Integrative Biodiversity Research (iDiv) Halle-Jena-Leipzig (Brose, 2018). In addition to predator–prey interactions, the database contains information on other biological data: mean body mass and length and movement and metabolic type for each species in the food web. Furthermore, it incorporates information about the interaction itself: type (predacious, herbivorous and detritivorous) and dimension (two or three dimensions according to the predator search space). In its current form, the Weddell Sea food web comprises 490 species and 16 041 predator–prey interactions and thus constitutes one of the most resolved food webs constructed to date (Jacob et al., 2011) (Fig. A1 in Appendix A).
Complex empirical food webs are compilations of species and their potential feeding interactions within a given area and time period. The species occurrence data to construct the Weddell Sea food web were sampled between 1983 and 2005, and hence the Weddell Sea food web is representative of this time period. The diet composition for each species was observed between 2001 and 2004 through a combination of field observations and stomach content analyses (further details can be found in Jacob, 2005). Some species, such as benthic grazers and suspension feeders, had poor taxonomic resolution of their prey; therefore laboratory information about their size, behaviour, and stable isotope signatures was used to infer their feeding habits (Jacob et al., 2011). As the data used to construct the Weddell Sea food web are sampled during the summer season due to the inaccessibility of ecological field sampling in Antarctica during the winter, the Weddell Sea food web is representative of the summer season. Moreover, the species in the food web are representative of adult specimens and were not categorised into ontogenetic life stages (i.e. larvae, juveniles, and adults). As a result, ontogenetic diet shifts cannot be addressed with this food web. The average body masses of species were either directly measured (Jacob, 2005) or taken from published accounts for marine mammals and seabirds (Brose et al., 2006). Due to the static nature of the Weddell Sea food web and its low temporal resolution, it is not possible to assess phenological mismatches, nor species temporal turnover. Because of these methodological limitations, the nature of the Weddell Sea food web data may likely lead to overestimations of some trophic interactions and network properties (connectance), compared to realised networks at any given point in time and space. The spatial extent of the food web is described in the “Study area” section (Sect. 2.1; see also Fig. 1).
2.3 Dataset analyses
2.3.1 Interaction strength estimation and distribution
To estimate the strength of each pairwise interaction in the food web, we followed an approach proposed by Pawar et al. (2012). The minimum data requirements are body mass of the consumer (predator) and resource (prey) and the interaction dimensionality (ID) classified as two or three dimensions. The ID is defined as the search space dimensions of the predator, which is also equivalent to the movement space of the prey. Thus, the ID is classified as 2D when both predator and prey move in 2D (e.g. both are benthic) or if a predator moves in 3D and a prey in 2D (e.g. pelagic predator on benthic prey). The ID is classified as 3D when both predator and prey move in 3D (e.g. both pelagic) or if the predator moves in 2D and the prey in 3D (e.g. benthic predator, pelagic prey) (Pawar et al., 2012).
GATEWAy v.1.0 provides information on the mean body mass for consumers and resources, except for “detritus” and “sediment”, and the dimensionality for the majority of the interactions, though the latter is missing in some cases (924 interactions). To complete the missing data on species “dimensionality”, we used information about the movement type of predators and prey included in GATEWAy.
The main equation we used for estimating the interaction strength IS was
where α is the search rate, xR is the resource density, and mR and mC are the body mass for the resource and the consumer, respectively (Pawar et al., 2012).
We obtained estimates for resource density and the search rate from the scaling relationships with the resource and the consumer mass, respectively (Pawar et al., 2012). The coefficients of such relationships, determined by ordinary least squares regression, vary with the ID. On the one hand, resource density scales with resource mass as a power law with different exponents in 2D and in 3D. Since mean mass for the resources “phytodetritus” and “sediment” was not available in GATEWAy, we considered the body mass of the smallest phytoplankton species (Fragilariopsis cylindrus) to be a proxy. This is justified by the fact that “phytodetritus” and “sediment” are mainly composed of dead or senescent phytoplankton reaching the seabed (Wolanski et al., 2011). On the other hand, search rate scales with consumer mass as a power law with exponents in 2D and in 3D (Pawar et al., 2012). The equations for estimating the search rate and the resource density are specified in the Supplement.
To quantify the variability in IS estimates, we considered the uncertainties inherent in estimating the exponents via standard linear regression. In this regard, the exponents reported in Pawar et al. (2012) can be viewed as the mean of a normal random variable, with the standard deviation given by the associated standard errors. We generated 1000 random samples and calculated an IS value for each sample, with the final outcome of a distribution of IS estimates for each interaction. Given that the IS distributions are right-skewed and bounded below zero, we characterised the central tendency using the median IS. To represent the distribution variability, we reported the interquartile range (Supplement, Fig. S1). This approach accounts for the propagation of uncertainty from the exponent estimates into the derived IS values, allowing us to systematically assess the robustness of conclusions based on these interaction metrics.
We fitted the IS distribution (i.e. medians for each interaction) to six candidate models (exponential, gamma, log-normal, normal, power law, and uniform), using maximum likelihood (McCallum, 2008), and selected the best-fitting model by computing the Akaike information criterion (AIC; Burnham and Anderson, 2002).
2.3.2 Species properties
To characterise the role of each species in the food web, we considered unweighted and weighted food web properties (Fig. 2). Unweighted properties are related to properties commonly used in qualitative food web studies and only describe the presence or absence of interactions without any information on link strength (Martinez, 1991; Dunne et al., 2002a; Borrelli and Ginzburg, 2014). In contrast, weighted properties capture the importance of a trophic interaction by considering its strength.
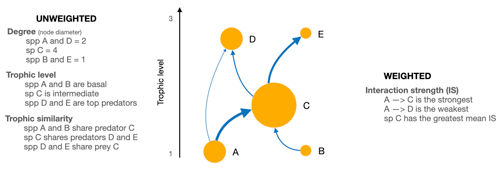
Figure 2Scheme of a network showing the weighted and unweighted properties we used to characterise the species of the Weddell Sea food web. Directed arrows indicate the flow of energy; the width of the arrow represents the interaction strength of it.
To assess species roles as a function of the weighted food web, we focused on mean IS defined as the average strength of all interactions for a given species. Further, we calculated three unweighted species properties: (a) species degree, i.e. the sum of in- and outgoing interactions; (b) trophic level; and (c) trophic similarity, i.e. the trophic overlap based on shared and unique resources and consumers. These metrics were chosen to assess species' roles based on the unweighted network. The species degree has often been linked to a species importance for the structure and functioning within a food web; i.e. perturbations to high-degree species may therefore have more significant effects on the food web robustness than low-degree species (Dunne et al., 2002b; references in Cirtwill et al., 2018). The trophic level offers information about a species vertical position in the food web, and top predators at higher trophic levels and primary producers at the base are particularly important for food web dynamics through top-down and bottom-up control (references in Cirtwill et al., 2018). Trophic similarity is an index of trophic overlap considering the set of prey and predators for a pair of species; it measures one of the most important aspects of a species' niches, the trophic niche (Martinez, 1991; Williams and Martinez, 2000).
Furthermore, we took species habitat affiliation into account, which describes the physical position of a species within the ecosystem. Species were categorised as (1) benthic, if it lives on the seafloor; (2) pelagic, if it lives close to the surface; (3) benthopelagic, if it moves between and connects the aforementioned environments; (4) demersal, if it lives and feeds on, or near, the bottom of the sea; and (5) land-based, if the consumer is not strictly aquatic but feeds predominantly on marine species. Species habitat affiliations were retrieved from Jacob et al. (2011).
To study the relationship between species mean IS (weighted property) and the unweighted species properties, we performed linear regression analyses between the log mean IS and each of the aforementioned unweighted properties. Thus, we considered the IS a dependent variable and the given unweighted property an independent variable and obtained the coefficients (slope and intercept) for the linear model. Models were fitted using the least-squares approach. We also explored the mean IS distribution with the species' habitat.
Formulas used to obtain the above species properties are described in the Supplement.
2.3.3 Extinction simulations and stability
To analyse the impact of species on food web stability, we performed extinction simulations deleting one species at a time; that is for every extinction, network size was reduced by one species only. After each extinction, we calculated the stability of the network minus the removed species (489 nodes) and compared it with that of the whole network (490 nodes in total).
To estimate stability, we utilised the Quasi-Sign Stability (QSS) index, defined as the mean of the real part of the maximum eigenvalue of the Jacobian (or community) matrix, employing random values for the elements of the Jacobian while preserving the predator–prey sign structure (Saravia et al., 2022; Allesina and Pascual, 2008; Pawar, 2009). The Jacobian matrix, denoted as C, is fundamental to understanding the population dynamics and inter-species interactions within an ecological network. Each element cij of C represents the effect of a change in the jth species' density on the ith species, at equilibrium. When i equals j, cij reflects the dependence of the ith species on its own density, offering insights into intra-species interactions and self-regulation. The maximum eigenvalue describes the rate at which a small disturbance decays or amplifies over time in the vicinity of an equilibrium. Precisely, the real parts of the eigenvalues indicate the rate of exponential growth (if positive) or decay (if negative) of perturbations. Thus, a more negative index is indicative of a more stable food web with reduced probability of perturbation amplification; in other words, the network is more resilient to disturbances.
We conducted 1000 simulations for the removal of each species, calculating the maximum eigenvalue for the network in each case. For each simulation, we compared this value against the median maximum eigenvalue obtained from 1000 simulations of the complete network, thus generating a distribution of differences. A positive difference indicates that the food web's stability is greater without the targeted species, suggesting that the species in question contributes to the network's instability. Conversely, a negative difference implies that the network is less stable without the species, indicating a stabilising effect. Due to the variability in the estimation of the eigenvalues, we decided to consider that a substantial impact on stability was reached when the proportion of either negative or positive differences within this distribution exceeded 0.55, meaning that 55 % of the time the difference in stability was positive or negative. A detailed description of the stability calculations can be found in the Supplement (Fig. S2).
To identify the species with the highest effect on food web stability and their characteristics, we plotted the results of each species' extinction and its effect on food web stability (i.e. the stability difference between the whole network and the network minus one species) against the weighted property (interaction strength) and the three unweighted properties (trophic level, degree, and trophic similarity) and species habitat affiliation.
All analyses were performed in R software, using the R packages igraph (Csárdi et al., 2023), cheddar (Hudson et al., 2013), and multiweb (Saravia, 2019). The source code and data are available at https://doi.org/10.5281/zenodo.10569545 (Marina and Saravia, 2024).
3.1 Interaction strength distribution
The statistical distribution that best fitted the empirical interaction strength distribution of the Weddell Sea food web was log-normal due to the high proportion of weak interactions and the existence of a few strong interactions (Fig. 3, Table S3).
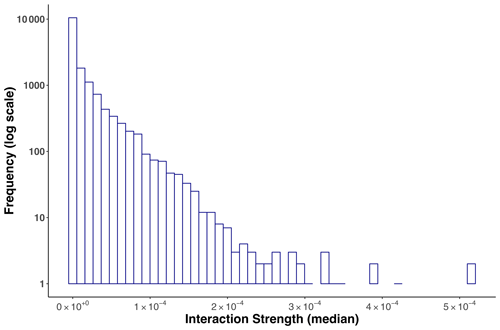
Figure 3Frequency distribution of (median) interaction strengths for the Weddell Sea food web. The distribution was best fitted to a log-normal model. The total number of interactions was 16 041.
Here it is important to note that we observed an important variability when estimating each IS. The interactions that showed the greatest variability, represented by a wide interquartile range, were those where consumer and resource body masses were very small (approximately kg). The frequency distribution of the interquartile range is presented in the Supplement (Fig. S1).
3.2 Species' role related to their mean interaction strength
We found that the species' mean IS (weighted property) shows different relationships with the unweighted properties analysed (Fig. 4a–d). In this regard, there is a positive relationship between IS and trophic level; i.e. the higher the trophic level of the species, the higher its mean IS. We also found a significant but less evident positive relationship with species degree. Conversely, there was no significant relationship between mean IS and trophic similarity. Considering species habitat affiliation, the “benthopelagic” and “pelagic” categories contained the two species with the highest mean IS, the orca Orcinus orca and the colossal squid Mesonychoteuthis hamiltoni, respectively. However, the majority of the species with relatively higher IS belonged to the “demersal” and “land-based” habitat groups. Species inhabiting the benthic realm showed the lowest mean IS (Fig. 4d).
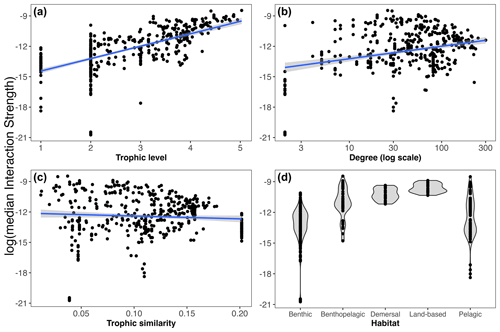
Figure 4Relationships between weighted (median interaction strength) and unweighted properties including habitat. Linear regressions are shown between log(median interaction strength) and trophic level (a), degree (b), and trophic similarity (c). Linear regressions for trophic level (, R2=0.46, p value < ), degree (, R2=0.03, p value = ) and trophic similarity (, R2=0.03, p value = 0.11). Panel (d) shows the distribution of the species' median interaction strength by habitat.
3.3 Species impact on food web stability
Our extinction analyses showed that the majority of species had no significant impact on food web stability after being removed (Fig. 5). Most of the species (yellow points in Fig. 5) did not change the stability of the network considerably after being removed, except for a few species (purple points in Fig. 5). Only 12 out of 490 species (2.45 %) gave rise to substantial changes in the food web's stability after their removal (Table 1). Network stability increased after the removal of most of these species; i.e. these species have a negative effect on stability. Only two species significantly decreased network stability after being removed, the minke whale Balaenoptera acutorostrata and the sea urchin Aporocidaris milleri. These two species have a positive effect on network stability.
Table 1Properties for the species with highest impact on the food web stability. Summary of maximum eigenvalue (Quasi-Sign Stability index – QSS) distribution of differences before and after performing extinction simulations in the Weddell Sea food web. Ordered by decreasing proportion of positive differences. medianIS: median interaction strength. TL: trophic level. Deg: degree. TS: trophic similarity. Prop dif QSS +: proportion of positive differences. Prop dif QSS −: proportion of negative differences.
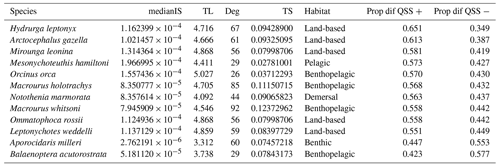
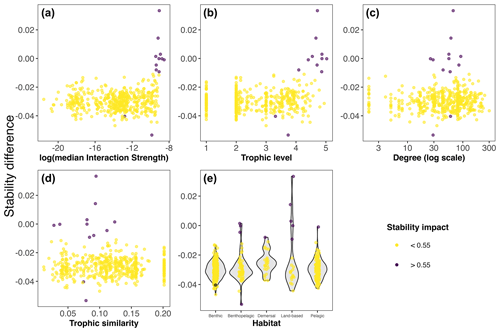
Figure 5Stability difference (median maximum eigenvalue) between the whole Weddell Sea food web (n=490) and the food web minus one species (n=489) for weighted (interaction strength) and unweighted species properties and habitat. Point colour indicates the impact on the stability; if substantial (>0.55 relative difference), the extinction of that species altered the stability of the food web.
After exploring the stability difference against the species properties (Fig. 5), we found that the species that generated a substantial impact on the stability of the food web were characterised by (1) high mean IS, (2) middle to high trophic levels (TL > 3.3), (3) a relatively high number of interactions (Degree > 25), and (4) middle to low trophic similarity (TS < 0.13). Habitat wise, species with a significant impact on stability were present in all habitats. Table 1 shows the results for the species with the highest impact on the food web stability.
4.1 Many weak and a few strong interactions
Our analyses show that the distribution of species IS at the network level is asymmetric; i.e. the Weddell Sea food web contains many weak interactions and only a few strong ones. This finding is consistent with many previous theoretical and empirical studies (e.g. McCann et al., 1998; Neutel et al., 2002; Emmerson and Raffaelli, 2004; Wootton and Emmerson, 2005; Kortsch et al., 2021). The asymmetric distribution of IS in food webs has been interpreted as an explanation for the persistence of complex communities in nature (Bascompte et al., 2005; Allesina et al., 2015; Nilsson and McCann, 2016). Here we show that this pattern is also prevalent in the Weddell Sea, one of the most complex food webs to date, comprising 490 species and 16 041 predator–prey interactions. This finding may also be used to validate our method.
4.2 Species' role related to their mean interaction strength
We employed a range of descriptors using both unweighted and weighted food web properties to characterise the dynamic and multifaceted nature of the Weddell Sea food web. Our results show a positive relationship between IS and trophic level and between IS and species degree. In the Weddell Sea, species with high degree also tend to have high mean ISs. This positive relationship between IS and species degree reinforces the central role of species in many interactions: species with a high degree (hubs) have a large impact on overall food web structure and functioning (Dunne et al., 2002b; Kortsch et al., 2015). On the other hand, the positive IS–trophic level relationship contradicts studies that suggest that mid-trophic level species (e.g. krill, mesopelagic fish, squid) are involved in the major pathways of energy flow in high-latitude marine ecosystems (Pinkerton and Bradford-Grieve, 2014; Murphy et al., 2016; McCormack et al., 2020; Riccialdelli et al., 2020). This contradiction may be explained by the lack of species biomass information in the calculation of IS in this study (Pawar et al., 2012). While the methodology allows information on species biomass or density to be included, this type of data was not available for the majority of species of the Weddell Sea food web. Overall, the combination of information on the quantity and quality of interactions and its relationship enabled a robust assessment of species' roles with regard to stability.
4.3 Species impact on food web stability
Based on our results, only a few species (2.45 %) play a key role with respect to the Weddell Sea food web stability. This is in concordance with other studies on complex empirical food webs in marine polar ecosystems (Kortsch et al., 2015; Marina et al., 2018; Rodriguez et al., 2022). Here these key species are characterised by a particular set of properties: high to mean IS, a middle to high trophic level, a relatively high number of interactions, and middle to low trophic similarity. In a previous study on sequential extinction simulations for the Weddell Sea food web (Jacob et al., 2011), it was found that larger-bodied-sized species could be lost without causing a collapse of the network. A caveat of this finding, also recognised by the authors, was that they did not consider the possibility of top–down extinctions or other indirect effects. In our study, we considered such top–down effects by including information on IS, which is of paramount importance when analysing the response of perturbations in ecological communities (McCann et al., 1998; Montoya et al., 2009; Novak et al., 2011). Thus, our study suggests that species with high mean IS and high trophic levels need to be considered with particular attention when trying to predict the effects of perturbations on the Weddell Sea ecosystem. This conclusion is further reinforced by the finding that these species have middle to low trophic similarity, which means that few other species of the food web can occupy the same trophic role.
The Weddell Sea ecosystem is subjected to multiple stressors triggered by global warming, of which spatial and temporal reduction in sea ice is one of the major structuring drivers in pelagic and benthic communities (Constable et al., 2014; Gutt et al., 2021). The opening in the ice might bring marine mammals (seals and whales), together altering both diet and foraging behaviour, as shown in the Ross Sea (Ainley et al., 2015). The mobile predators at high trophic levels, such as marine mammals, are the ones with the highest impact on community stability, according to our analyses. Therefore, if the Weddell Sea were to become warmer and ice-free for longer periods of time (Turner et al., 2020), this could lead to changes in food web stability due to top–down effects, potentially resulting in trophic cascades.
4.4 Caveats and uncertainties
One of the primary uncertainties in our analysis stems from structural variability due to, for instance, temporal changes. The Weddell Sea food web, like many high-latitude marine ecosystems, exhibits significant seasonal changes (McMeans et al., 2015; Rossi et al., 2019; Kortsch et al., 2021; Pecuchet et al., 2022). Our study primarily focuses on data from late spring to late summer, potentially overlooking the intricacies of the winter season. Seasonal shifts can lead to variations in species interactions, trophic levels, and overall network structure. Novotny et al. (2023) recently highlighted the importance of considering seasonal variability in food web analyses by showing distinct differences in species diet over seasons.
Another source of uncertainty stems from the parameters and data used to estimate species IS. While we accounted for variability from estimated resource density and consumer search rate (Pawar et al., 2012), we did not consider potential variability arising from changes in species' body mass. Indeed, one could estimate the interaction strength based on body-mass distributions rather than a single empirical value. Future research should consider this source of variability since it could alter food web stability outcomes (Brose et al., 2006; Allesina et al., 2015; Gross et al., 2009; Landi et al., 2018). Concerning body mass, the model utilised in this study exhibits sensitivity in estimating interaction strengths (IS) among species with exceedingly small body masses (approximately kg), as evidenced by a broad interquartile range. Additionally, some of the observed results may stem from the fact that the applied methodology does not incorporate empirically measured biomasses for species. For example, we would have expected a relatively high impact on community stability when removing the Antarctic krill Euphausia superba as it is one of the most important prey species in the food web based on field observations (Atkinson et al., 2019). Moreover, it is one of the species most affected by warming, and its population has already declined as a result of productivity changes and increased access by predators (Atkinson et al., 2004; Kawaguchi et al., 2009). Although our results did not show a significant impact on the stability of the Weddell Sea food web when krill went extinct, we were able to frame it as a key species in terms of its weighted and unweighted characteristics due to its high mean IS, mid-trophic level, and high number of interactions.
The best solution when estimating and evaluating the importance of IS in highly resolved food webs would probably be to consider more than one methodological approach.Various methodologies have been developed to estimate species IS in food webs, for example, the conceptual framework of the food web energetics (Gauzens et al., 2019) and the inverse model perspective (Gellner et al., 2023). Although we applied a particular methodology (Pawar et al., 2012), we anticipate that a promising research endeavour would be to compare and contrast our results with those derived from alternative methodologies, which is beyond the scope of our study. Furthermore, a comprehensive review of the diverse methodologies to assign interaction strengths might provide insights into the opportunities and limitations of each approach, guiding the usefulness of each for addressing particular questions in future research.
Our study goes beyond the current understanding of how species influence ecosystem structure and stability in polar marine ecosystems (Murphy et al., 2016; McCormack et al., 2021). The integration of weighted (IS) and unweighted network and species properties allowed us to identify which species and which species' food web characteristics have a destabilising or stabilising effect on the food web. This enables a more complete assessment of species' role with respect to food web structure and stability.
We consider that the information provided in this study can be useful for the development of effective policies and management strategies, particularly given the ongoing initiative to implement a marine protected area (MPA) in the Weddell Sea region (Teschke et al., 2021).
The code used to perform all analyses and create the figures of this work are publicly available via Zenodo: https://doi.org/10.5281/zenodo.10569545 (Marina and Saravia, 2024).
The dataset used in this study was retrieved from GlobAL daTabasE of traits and food Web Architecture (GATEWAy, v1.0, https://doi.org/10.25829/IDIV.283-3-756, Brose, 2018) and modified accordingly. Modifications are explained in the main text (Sect. 2.2 and 2.3 in Methodology). The original and modified versions of the dataset are available via Zenodo: https://doi.org/10.5281/zenodo.10569545 (Marina and Saravia, 2024).
The supplement related to this article is available online at: https://doi.org/10.5194/os-20-141-2024-supplement.
TIM and LAS: conceptualisation (lead), data curation (lead), formal analysis (lead), methodology (lead), coding (lead), writing – original draft (lead), and writing – review and editing (lead). SK: conceptualisation (lead), formal analysis (supporting), methodology (supporting), coding (supporting), writing – original draft (supporting), and writing – review and editing (supporting).
The contact author has declared that none of the authors has any competing interests.
Publisher’s note: Copernicus Publications remains neutral with regard to jurisdictional claims made in the text, published maps, institutional affiliations, or any other geographical representation in this paper. While Copernicus Publications makes every effort to include appropriate place names, the final responsibility lies with the authors.
This article is part of the special issue “The Weddell Sea and the ocean off Dronning Maud Land: unique oceanographic conditions shape circumpolar and global processes – a multi-disciplinary study (OS/BG/TC inter-journal SI)”. It is not associated with a conference.
We thank all persons that dedicated time to build and curate the dataset GATEWAy that enabled us to carry out this work. We also thank the organisers of the different virtual workshops of the Weddell Sea and Droning Maud Land (WSDML) Regional Working Group for giving us the possibility to participate in and contribute to this special issue.
This paper was edited by Mario Hoppema and reviewed by one anonymous referee.
Ainley, D. G., Ballard, G., Jones, R. M., Jongsomjit, D., Pierce, S. D., Smith Jr., W. O., and Veloz, S.: Trophic Cascades in the Western Ross Sea, Antarctica: Revisited, Mar. Ecol.-Prog. Ser., 534, 1–16, https://doi.org/10.3354/meps11394, 2015. a
Allesina, S. and Pascual, M.: Network Structure, Predator–Prey Modules, and Stability in Large Food Webs, Theor. Ecol., 1, 55–64, https://doi.org/10.1007/s12080-007-0007-8, 2008. a
Allesina, S., Grilli, J., Barabás, G., Tang, S., Aljadeff, J., and Maritan, A.: Predicting the Stability of Large Structured Food Webs, Nat. Commun., 6, 7842, https://doi.org/10.1038/ncomms8842, 2015. a, b, c
Atkinson, A., Siegel, V., Pakhomov, E., and Rothery, P.: Long-Term Decline in Krill Stock and Increase in Salps within the Southern Ocean, Nature, 432, 100–103, https://doi.org/10.1038/nature02996, 2004. a
Atkinson, A., Hill, S. L., Pakhomov, E. A., Siegel, V., Reiss, C. S., Loeb, V. J., Steinberg, D. K., Schmidt, K., Tarling, G. A., Gerrish, L., and Sailley, S. F.: Krill (Euphausia Superba) Distribution Contracts Southward during Rapid Regional Warming, Nat. Clim. Change, 9, 142–147, https://doi.org/10.1038/s41558-018-0370-z, 2019. a
Bascompte, J., Melián, C. J., and Sala, E.: Interaction Strength Combinations and the Overfishing of a Marine Food Web, P. Natl. Acad. Sci. USA, 102, 5443–5447, https://doi.org/10.1073/pnas.0501562102, 2005. a
Berlow, E. L., Neutel, A.-M., Cohen, J. E., De Ruiter, P. C., Ebenman, B., Emmerson, M., Fox, J. W., Jansen, V. A. A., Iwan Jones, J., Kokkoris, G. D., Logofet, D. O., McKane, A. J., Montoya, J. M., and Petchey, O.: Interaction Strengths in Food Webs: Issues and Opportunities, J. Anim. Ecol., 73, 585–598, https://doi.org/10.1111/j.0021-8790.2004.00833.x, 2004. a
Borrelli, J. J. and Ginzburg, L. R.: Why There Are so Few Trophic Levels: Selection against Instability Explains the Pattern, Food Webs, 1, 10–17, https://doi.org/10.1016/j.fooweb.2014.11.002, 2014. a
Brose, U.: GlobAL daTabasE of Traits and Food Web Architecture (GATEWAy) Version 1.0, iDiv repository [data set], https://doi.org/10.25829/IDIV.283-3-756, 2018. a, b
Brose, U., Jonsson, T., Berlow, E. L., Warren, P., Banasek-Richter, C., Bersier, L.-F., Blanchard, J. L., Brey, T., Carpenter, S. R., Blandenier, M.-F. C., Cushing, L., Dawah, H. A., Dell, T., Edwards, F., Harper-Smith, S., Jacob, U., Ledger, M. E., Martinez, N. D., Memmott, J., Mintenbeck, K., Pinnegar, J. K., Rall, B. C., Rayner, T. S., Reuman, D. C., Ruess, L., Ulrich, W., Williams, R. J., Woodward, G., and Cohen, J. E.: Consumer–Resource Body-Size Relationships in Natural Food Webs, Ecology, 87, 2411–2417, https://doi.org/10.1890/0012-9658(2006)87[2411:CBRINF]2.0.CO;2, 2006. a, b
Brose, U., Archambault, P., Barnes, A. D., Bersier, L.-F., Boy, T., Canning-Clode, J., Conti, E., Dias, M., Digel, C., Dissanayake, A., Flores, A. A. V., Fussmann, K., Gauzens, B., Gray, C., Häussler, J., Hirt, M. R., Jacob, U., Jochum, M., Kéfi, S., McLaughlin, O., MacPherson, M. M., Latz, E., Layer-Dobra, K., Legagneux, P., Li, Y., Madeira, C., Martinez, N. D., Mendonça, V., Mulder, C., Navarrete, S. A., O'Gorman, E. J., Ott, D., Paula, J., Perkins, D., Piechnik, D., Pokrovsky, I., Raffaelli, D., Rall, B. C., Rosenbaum, B., Ryser, R., Silva, A., Sohlström, E. H., Sokolova, N., Thompson, M. S. A., Thompson, R. M., Vermandele, F., Vinagre, C., Wang, S., Wefer, J. M., Williams, R. J., Wieters, E., Woodward, G., and Iles, A. C.: Predator Traits Determine Food-Web Architecture across Ecosystems, Nat. Ecol. Evol., https://doi.org/10.1038/s41559-019-0899-x, 2019. a, b
Burnham, K. P. and Anderson, D. R.: Model Selection and Multimodel Inference: A Practical Information-Theoretic Approach, Springer, New YorkBurnham KP, Anderson DR, Huyvaert KP (2011) AIC model selection and multimodel inference in behavioral ecology: some background, observations, and comparisons, Behav. Ecol. Sociobiol., 65, 23–35, 2002. a
Carrara, F., Giometto, A., Seymour, M., Rinaldo, A., and Altermatt, F.: Inferring Species Interactions in Ecological Communities: A Comparison of Methods at Different Levels of Complexity, Meth. Ecol. Evol., 6, 895–906, https://doi.org/10.1111/2041-210X.12363, 2015. a, b
Carscallen, W. M. A. and Romanuk, T. N.: Structure and Robustness to Species Loss in Arctic and Antarctic Ice-Shelf Meta-Ecosystem Webs, Ecol. Model., 245, 208–218, https://doi.org/10.1016/j.ecolmodel.2012.03.027, 2012. a
Chang, C.-W., Miki, T., Ushio, M., Ke, P.-J., Lu, H.-P., Shiah, F.-K., and Hsieh, C.-H.: Reconstructing Large Interaction Networks from Empirical Time Series Data, Ecol. Lett., 24, 2763–2774, https://doi.org/10.1111/ele.13897, 2021. a
Cirtwill, A. R., Dalla Riva, G. V., Gaiarsa, M. P., Bimler, M. D., Cagua, E. F., Coux, C., and Dehling, D. M.: A Review of Species Role Concepts in Food Webs, Food Webs, 16, e00093, https://doi.org/10.1016/j.fooweb.2018.e00093, 2018. a, b, c
Constable, A. J., Melbourne-Thomas, J., Corney, S. P., Arrigo, K. R., Barbraud, C., Barnes, D. K. A., Bindoff, N. L., Boyd, P. W., Brandt, A., Costa, D. P., Davidson, A. T., Ducklow, H. W., Emmerson, L., Fukuchi, M., Gutt, J., Hindell, M. A., Hofmann, E. E., Hosie, G. W., Iida, T., Jacob, S., Johnston, N. M., Kawaguchi, S., Kokubun, N., Koubbi, P., Lea, M.-A., Makhado, A., Massom, R. A., Meiners, K., Meredith, M. P., Murphy, E. J., Nicol, S., Reid, K., Richerson, K., Riddle, M. J., Rintoul, S. R., Smith, W. O., Southwell, C., Stark, J. S., Sumner, M., Swadling, K. M., Takahashi, K. T., Trathan, P. N., Welsford, D. C., Weimerskirch, H., Westwood, K. J., Wienecke, B. C., Wolf-Gladrow, D., Wright, S. W., Xavier, J. C., and Ziegler, P.: Climate Change and Southern Ocean Ecosystems I: How Changes in Physical Habitats Directly Affect Marine Biota, Glob. Change Biol., 20, 3004–3025, https://doi.org/10.1111/gcb.12623, 2014. a
Cordone, G., Salinas, V., Marina, T. I., Doyle, S. R., Pasotti, F., Saravia, L. A., and Momo, F. R.: Green vs Brown Food Web: Effects of Habitat Type on Multidimensional Stability Proxies for a Highly-Resolved Antarctic Food Web, Food Webs, 25, e00166, https://doi.org/10.1016/j.fooweb.2020.e00166, 2020. a
Csárdi, G., Nepusz, T., Traag, V., Horvát, S., Zanini, F., Noom, D., Müller, K.: igraph: Network Analysis and Visualization in R, Zenodo [data set], https://doi.org/10.5281/zenodo.7682609, 2023 (R package version 1.6.0, available at: https://CRAN.R-project.org/package=igraph, last access: 25 January 2024). a
Dayton, P. K.: Polar Benthos. Polar Oceanography Part B: Chemistry, Biology and Geology, edited by: Smith, W. O., Academic Press, London, 631–685, https://doi.org/10.1016/B978-0-08-092595-0.50010-6, 1990. a
de Santana, C. N., Rozenfeld, A. F., Marquet, P. A., and Duarte, C. M.: Topological Properties of Polar Food Webs, Mar. Ecol.-Prog. Ser., 474, 15–26, https://doi.org/10.3354/meps10073, 2013. a
de Steur, L., Gutt, J., and Moreau, S.: Report from the Workshop on the Development of the Weddell Sea – Dronning Maud Land Regional Working Group SOOS, Zenodo, https://doi.org/10.5281/zenodo.3941419, 2019. a
Dunne, J. A., Williams, R. J., and Martinez, N. D.: Food-Web Structure and Network Theory: The Role of Connectance and Size, P. Natl. Acad. Sci. USA, 99, 12917–12922, https://doi.org/10.1073/pnas.192407699, 2002a. a
Dunne, J. A., Williams, R. J., and Martinez, N. D.: Network Structure and Biodiversity Loss in Food Webs: Robustness Increases with Connectance, Ecol. Lett., 5, 558–567, https://doi.org/10.1046/j.1461-0248.2002.00354.x, 2002b. a, b
Emmerson, M. C. and Raffaelli, D.: Predator–Prey Body Size, Interaction Strength and the Stability of a Real Food Web, J. Anim. Ecol., 73, 399–409, https://doi.org/10.1111/j.0021-8790.2004.00818.x, 2004. a, b, c
Fahimipour, A. K. and Hein, A. M.: The Dynamics of Assembling Food Webs, Ecol. Lett., 17, 606–613, https://doi.org/10.1111/ele.12264, 2014. a
Fahrbach, E., Beszczynska-Möller, A., and Rohardt, G.: Polar Oceans – an Oceanographic Overview, in: Biological Studies in Polar Oceans – Exploration of Life in Icy Waters, edited by: Hempel, G. and Hempel, H., 17–36, Wirtschaftsverlag NW, Bremerhaven, ISBN 978-3-86509-865-8, 2009. a
Gauzens, B., Barnes, A., Giling, D. P., Hines, J., Jochum, M., Lefcheck, J. S., Rosenbaum, B., Wang, S., and Brose, U.: Fluxweb: An R Package to Easily Estimate Energy Fluxes in Food Webs, Meth. Ecol. Evol., 10, 270–279, https://doi.org/10.1111/2041-210X.13109, 2019. a, b
Gellner, G., McCann, K., and Hastings, A.: Stable Diverse Food Webs Become More Common When Interactions Are More Biologically Constrained, P. Natl. Acad. Sci. USA, 120, e2212061120, https://doi.org/10.1073/pnas.2212061120, 2023. a
Griffiths, H. J., Meijers, A. J. S., and Bracegirdle, T. J.: More Losers than Winners in a Century of Future Southern Ocean Seafloor Warming, Nat. Clim. Change, 7, 749–754, https://doi.org/10.1038/nclimate3377, 2017. a
Gross, T., Rudolf, L., Levin, S. A., and Dieckmann, U.: Generalized Models Reveal Stabilizing Factors in Food Webs, Science, 325, 747–750, https://doi.org/10.1126/science.1173536, 2009. a
Gutt, J., Isla, E., Xavier, J. C., Adams, B. J., Ahn, I.-Y., Cheng, C.-H. C., Colesie, C., Cummings, V. J., di Prisco, G., Griffiths, H., Hawes, I., Hogg, I., McIntyre, T., Meiners, K. M., Pearce, D. A., Peck, L., Piepenburg, D., Reisinger, R. R., Saba, G. K., Schloss, I. R., Signori, C. N., Smith, C. R., Vacchi, M., Verde, C., and Wall, D. H.: Antarctic Ecosystems in Transition – Life between Stresses and Opportunities, Biol. Rev., 96, 798–821, https://doi.org/10.1111/brv.12679, 2021. a
Houstin, A., Zitterbart, D. P., Heerah, K., Eisen, O., Planas-Bielsa, V., Fabry, B., and Le Bohec, C.: Juvenile Emperor Penguin Range Calls for Extended Conservation Measures in the Southern Ocean, Roy. Soc. Open Sci., 9, 211708, https://doi.org/10.1098/rsos.211708, 2022. a
Hudson, L. N., Emerson, R., Jenkins, G. B., Layer, K., Ledger, M. E., Pichler, D. E., Thompson, M. S. A., O'Gorman, E. J., Woodward, G., and Reuman, D. C.: Cheddar: Analysis and Visualisation of Ecological Communities in R, Meth. Ecol. Evol., 4, 99–104, https://doi.org/10.1111/2041-210X.12005, 2013. a
Jacob, U.: Trophic Dynamics of Antarctic Shelf Ecosystems-Food Webs and Energy Flow Budgets, PhD thesis, Universität Bremen, https://media.suub.uni-bremen.de/handle/elib/2798 (last access: 25 January 2024), 2005. a, b
Jacob, U., Thierry, A., Brose, U., Arntz, W. E., Berg, S., Brey, T., Fetzer, I., Jonsson, T., Mintenbeck, K., Möllmann, C., Petchey, O. L., Riede, J. O., and Dunne, J. A.: The Role of Body Size in Complex Food Webs: A Cold Case, in: Advances in Ecological Research, edited by: Belgrano, A., Academic Press, https://doi.org/10.1016/B978-0-12-386475-8.00005-8, 2011. a, b, c, d, e, f
Kawaguchi, S., Nicol, S., and Press, A. J.: Direct Effects of Climate Change on the Antarctic Krill Fishery, Fisheries Manag. Ecol., 16, 424–427, https://doi.org/10.1111/j.1365-2400.2009.00686.x, 2009. a
Kortsch, S., Primicerio, R., Fossheim, M., Dolgov, A. V., and Aschan, M.: Climate Change Alters the Structure of Arctic Marine Food Webs Due to Poleward Shifts of Boreal Generalists, P. Roy. Soc. B-Biol. Sci., 282, 20151546, https://doi.org/10.1098/rspb.2015.1546, 2015. a, b, c
Kortsch, S., Primicerio, R., Aschan, M., Lind, S., Dolgov, A. V., and Planque, B.: Food-Web Structure Varies along Environmental Gradients in a High-Latitude Marine Ecosystem, Ecography, 42, 295–308, https://doi.org/10.1111/ecog.03443, 2019. a, b
Kortsch, S., Frelat, R., Pecuchet, L., Olivier, P., Putnis, I., Bonsdorff, E., Ojaveer, H., Jurgensone, I., Strāķe, S., Rubene, G., Krūze, Ē., and Nordström, M. C.: Disentangling Temporal Food Web Dynamics Facilitates Understanding of Ecosystem Functioning, J. Anim. Ecol., 90, 1205–1216, https://doi.org/10.1111/1365-2656.13447, 2021. a, b, c, d, e, f
Landi, P., Minoarivelo, H. O., Brännström, Å., Hui, C., and Dieckmann, U.: Complexity and Stability of Ecological Networks: A Review of the Theory, Popul. Ecol., 60, 319–345, https://doi.org/10.1007/s10144-018-0628-3, 2018. a
Marina, T. I. and Saravia, L. A.: EcoComplex/WeddellSea: New insights into the Weddell Sea ecosystem applying a network approach (v1.0.0), Zenodo [code, data set], https://doi.org/10.5281/zenodo.10569545, 2024. a, b, c
Marina, T. I., Salinas, V., Cordone, G., Campana, G., Moreira, E., Deregibus, D., Torre, L., Sahade, R., Tatián, M., Barrera Oro, E., De Troch, M., Doyle, S., Quartino, M. L., Saravia, L. A., and Momo, F. R.: The Food Web of Potter Cove (Antarctica): Complexity, Structure and Function, Estuar. Coast. Shelf S., 200, 141–151, https://doi.org/10.1016/j.ecss.2017.10.015, 2018. a, b
Martinez, N. D.: Artifacts or Attributes? Effects of Resolution on the Little Rock Lake Food Web, Ecol. Monogr., 61, 367–392, https://doi.org/10.2307/2937047, 1991. a, b
McCallum, H.: Population Parameters: Estimation for Ecological Models, John Wiley & Sons, ISBN 978-0-470-75742-0, 2008. a
McCann, K., Hastings, A., and Huxel, G. R.: Weak Trophic Interactions and the Balance of Nature, Nature, 395, 794–798, https://doi.org/10.1038/27427, 1998. a, b, c, d
McCormack, S. A., Melbourne-Thomas, J., Trebilco, R., Blanchard, J. L., and Constable, A.: Alternative Energy Pathways in Southern Ocean Food Webs: Insights from a Balanced Model of Prydz Bay, Antarctica, Deep-Sea Res. Pt. II, 174, 1–13, https://doi.org/10.1016/j.dsr2.2019.07.001, 2020. a
McCormack, S. A., Melbourne-Thomas, J., Trebilco, R., Griffith, G., Hill, S. L., Hoover, C., Johnston, N. M., Marina, T. I., Murphy, E. J., Pakhomov, E. A., Pinkerton, M., Plagányi, É., Saravia, L. A., Subramaniam, R. C., Van de Putte, A. P., and Constable, A. J.: Southern Ocean Food Web Modelling: Progress, Prognoses, and Future Priorities for Research and Policy Makers, Front. Ecol. Evol., 9, 626, https://doi.org/10.3389/fevo.2021.624763, 2021. a
McMeans, B. C., McCann, K. S., Humphries, M., Rooney, N., and Fisk, A. T.: Food Web Structure in Temporally-Forced Ecosystems, Trend. Ecol. Evol., 30, 662–672, https://doi.org/10.1016/j.tree.2015.09.001, 2015. a
Montoya, J. M., Woodward, G., Emmerson, M. C., and Solé, R. V.: Press Perturbations and Indirect Effects in Real Food Webs, Ecology, 90, 2426–2433, https://doi.org/10.1890/08-0657.1, 2009. a
Murphy, E. J., Cavanagh, R. D., Drinkwater, K. F., Grant, S. M., Heymans, J. J., Hofmann, E. E., Hunt, G. L., and Johnston, N. M.: Understanding the Structure and Functioning of Polar Pelagic Ecosystems to Predict the Impacts of Change, P. Roy. Soc. B, 283, 20161646, https://doi.org/10.1098/rspb.2016.1646, 2016. a, b
Neutel, A.-M. and Thorne, M. A. S.: Interaction Strengths in Balanced Carbon Cycles and the Absence of a Relation between Ecosystem Complexity and Stability, Ecol. Lett., 17, 651–661, https://doi.org/10.1111/ele.12266, 2014. a
Neutel, A.-M., Heesterbeek, J. A. P., and de Ruiter, P. C.: Stability in Real Food Webs: Weak Links in Long Loops, Science, 296, 1120–1123, https://doi.org/10.1126/science.1068326, 2002. a, b
Nilsson, K. A. and McCann, K. S.: Interaction Strength Revisited–Clarifying the Role of Energy Flux for Food Web Stability, Theor. Ecol., 9, 59–71, https://doi.org/10.1007/s12080-015-0282-8, 2016. a, b
Novak, M., Wootton, J. T., Doak, D. F., Emmerson, M., Estes, J. A., and Tinker, M. T.: Predicting Community Responses to Perturbations in the Face of Imperfect Knowledge and Network Complexity, Ecology, 92, 836–846, https://doi.org/10.1890/10-1354.1, 2011. a
Novotny, A., Serandour, B., Kortsch, S., Gauzens, B., Jan, K. M. G., and Winder, M.: DNA Metabarcoding Highlights Cyanobacteria as the Main Source of Primary Production in a Pelagic Food Web Model, Sci. Adv., 9, eadg1096, https://doi.org/10.1126/sciadv.adg1096, 2023. a
Paine, R. T.: Food-Web Analysis through Field Measurement of per Capita Interaction Strength, Nature, 355, 73–75, 1992. a, b
Pascual, M., Dunne, J. A., and Dunne, J. A.: Ecological Networks: Linking Structure to Dynamics in Food Webs, edited by: Pascual, M. and Dunne, J. A., Oxford University Press, USA, ISBN 978-0-19-518816-5, 2006. a
Pawar, S.: Community Assembly, Stability and Signatures of Dynamical Constraints on Food Web Structure, J. Theor. Biol., 259, 601–612, https://doi.org/10.1016/j.jtbi.2009.04.006, 2009. a
Pawar, S., Dell, A. I., and Savage, V. M.: Dimensionality of Consumer Search Space Drives Trophic Interaction Strengths, Nature, 486, 485–489, https://doi.org/10.1038/nature11131, 2012. a, b, c, d, e, f, g, h, i, j
Pecuchet, L., Jørgensen, L. L., Dolgov, A. V., Eriksen, E., Husson, B., Skern-Mauritzen, M., and Primicerio, R.: Spatio-Temporal Turnover and Drivers of Bentho-Demersal Community and Food Web Structure in a High-Latitude Marine Ecosystem, Divers. Distrib., 28, 2503–2520, https://doi.org/10.1111/ddi.13580, 2022. a, b, c, d
Perkins, D. M., Hatton, I. A., Gauzens, B., Barnes, A. D., Ott, D., Rosenbaum, B., Vinagre, C., and Brose, U.: Consistent Predator-Prey Biomass Scaling in Complex Food Webs, Nat. Commun., 13, 4990, https://doi.org/10.1038/s41467-022-32578-5, 2022. a
Pinkerton, M. H. and Bradford-Grieve, J. M.: Characterizing Foodweb Structure to Identify Potential Ecosystem Effects of Fishing in the Ross Sea, Antarctica, ICES J. Mar. Sci., 71, 1542–1553, https://doi.org/10.1093/icesjms/fst230, 2014. a
Riccialdelli, L., Becker, Y. A., Fioramonti, N. E., Torres, M., Bruno, D. O., Rey, A. R., and Fernández, D. A.: Trophic Structure of Southern Marine Ecosystems: A Comparative Isotopic Analysis from the Beagle Channel to the Oceanic Burdwood Bank Area under a Wasp-Waist Assumption, Mar. Ecol.-Prog. Ser., 655, 1–27, https://doi.org/10.3354/meps13524, 2020. a
Rodriguez, I. D., Marina, T. I., Schloss, I. R., and Saravia, L. A.: Marine Food Webs Are More Complex but Less Stable in Sub-Antarctic (Beagle Channel, Argentina) than in Antarctic (Potter Cove, Antarctic Peninsula) Regions, Mar. Environ. Res., 174, 105561, https://doi.org/10.1016/j.marenvres.2022.105561, 2022. a, b
Rossi, L., Sporta Caputi, S., Calizza, E., Careddu, G., Oliverio, M., Schiaparelli, S., and Costantini, M. L.: Antarctic Food Web Architecture under Varying Dynamics of Sea Ice Cover, Sci. Rep., 9, 12454, https://doi.org/10.1038/s41598-019-48245-7, 2019. a, b, c
Saravia, L. A.: Multiweb: R Package for Multiple Interaction Ecological Networks, Zenodo, https://doi.org/10.5281/zenodo.3370396, 2019. a
Saravia, L. A., Marina, T. I., Kristensen, N. P., De Troch, M., and Momo, F. R.: Ecological Network Assembly: How the Regional Metaweb Influences Local Food Webs, J. Anim. Ecol., 91, 630–642, https://doi.org/10.1111/1365-2656.13652, 2022. a
Teixidó, N., Garrabou, J., and Arntz, W.: Spatial Pattern Quantification of Antarctic Benthic Communities Using Landscape Indices, Mar. Ecol.-Prog. Ser., 242, 1–14, https://doi.org/10.3354/meps242001, 2002. a
Teschke, K., Brtnik, P., Hain, S., Herata, H., Liebschner, A., Pehlke, H., and Brey, T.: Planning Marine Protected Areas under the CCAMLR Regime – The Case of the Weddell Sea (Antarctica), Mar. Policy, 124, 104370, https://doi.org/10.1016/j.marpol.2020.104370, 2021. a, b
Thompson, R. M., Brose, U., Dunne, J. A., Hall Jr., R. O., Hladyz, S., Kitching, R. L., Martinez, N. D., Rantala, H., Romanuk, T. N., Stouffer, D. B., and Tylianakis, J. M.: Food Webs: Reconciling the Structure and Function of Biodiversity, Trend. Ecol. Evol., 27, 689–697, https://doi.org/10.1016/j.tree.2012.08.005, 2012. a
Turner, J., Guarino, M. V., Arnatt, J., Jena, B., Marshall, G. J., Phillips, T., Bajish, C. C., Clem, K., Wang, Z., Andersson, T., Murphy, E. J., and Cavanagh, R.: Recent Decrease of Summer Sea Ice in the Weddell Sea, Antarctica, Geophys. Res. Lett., 47, e2020GL087127, https://doi.org/10.1029/2020GL087127, 2020. a
Williams, R. J. and Martinez, N. D.: Simple Rules Yield Complex Food Webs, Nature, 404, 180–183, https://doi.org/10.1038/35004572, 2000. a
Wolanski, E., McLusky, D., Wilson, J. G., and Luczkovich, J. J.: Trophic Relationships of Coastal and Estuarine Ecosystems, Vol. 6, Academic Press, London, UK, ISBN 978-0-12-374711-2, 2011. a
Wootton, J. T. and Emmerson, M.: Measurement of Interaction Strength in Nature, Annu. Rev. Ecol. Evol. S., 36, 419–444, 2005. a, b