the Creative Commons Attribution 4.0 License.
the Creative Commons Attribution 4.0 License.
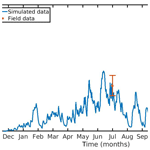
Modelling mussel (Mytilus spp.) microplastic accumulation
Natalia Stamataki
Yannis Hatzonikolakis
Kostas Tsiaras
Catherine Tsangaris
George Petihakis
Sarantis Sofianos
George Triantafyllou
Microplastics (MPs) are a contaminant of growing concern due to their widespread distribution and interactions with marine species, such as filter feeders. To investigate the MPs accumulation in wild and cultured mussels, a dynamic energy budget (DEB) model was developed and validated with the available field data of Mytilus edulis (M. edulis, wild) from the North Sea and Mytilus galloprovincialis (M. galloprovincialis, cultured) from the northern Ionian Sea. Towards a generic DEB model, the site-specific model parameter, half-saturation coefficient (Xk), was applied as a power function of food density for the cultured mussel, while for the wild mussel it was calibrated to a constant value. The DEB-accumulation model simulated the uptake and excretion rate of MPs, taking into account environmental characteristics (temperature and chlorophyll a). An accumulation of MPs equal to 0.53 particles per individual (fresh tissue mass 1.9 g) and 0.91 particles per individual (fresh tissue mass 3.3 g) was simulated for the wild and cultured mussel after 4 and 1 years respectively, in agreement with the field data. The inverse experiments investigating the depuration time of the wild and cultured mussel in a clean-from-MPs environment showed a 90 % removal of MPs load after 2.5 and 12 d respectively. Furthermore, sensitivity tests on model parameters and forcing functions highlighted that besides MPs concentration, the accumulation is highly dependent on temperature and chlorophyll a of the surrounding environment. For this reason, an empirical equation was found, directly relating the environmental concentration of MPs, with the seawater temperature, chlorophyll a, and the mussel's soft tissue MPs load.
- Article
(2520 KB) - Full-text XML
- BibTeX
- EndNote
Microplastic (MP) particles are synthetic organic polymers with size below 5 mm (Arthur et al., 2009) that originate from a variety of sources including the following: those particles that are manufactured for particular household or industrial activities, such as facial scrubs, toothpastes, and resin pellets used in the plastic industry (primary MPs), and those formed from the fragmentation of larger plastic items (secondary MPs) (GESAMP, 2015). Eriksen et al. (2014) estimated that more than 5 trillion microplastic particles, weighing over 250 000 t, float in the oceans. Due to their composition, density, and shape, MPs are highly persistent in the environment and are, therefore, accumulating at different rates in different marine compartments: surface and deeper layers in the water column, as well as at the seafloor and within the sediments (Moore et al., 2001; Lattin et al., 2004; Thompson, 2004; Lusher, 2015). Since the majority of MPs entering the marine environment originate from the land (i.e. landfills, littering of beaches and coastal areas, rivers, floodwaters, untreated municipal sewerage, and industrial emissions), the threat of MPs pollution in the coastal zone puts considerable pressure on the coastal ecosystems (Cole et al., 2011; Andrady, 2011). In recent years, initiatives under various projects (i.e. CLAIM, DeFishGear) aim at evaluating the threat and impact of marine litter pollution; the European framework of JERICO-RI focuses on a sustainable research infrastructure in the coastal area to support the monitoring, science, and management of coastal marine areas (http://www.jerico-ri.eu/, last access: 27 July 2020). In the framework of JERICO-NEXT, a recent study addressed the environmental threats and gaps with monitoring programmes in European coastal waters, including the marine litter (i.e. MPs), as one of the most commonly identified threats to the marine environment and highlighted the need for improved monitoring of the MPs distribution and their impacts in European coastal environments (Painting et al., 2020).
Numerous studies have revealed that MPs are ingested either directly or through lower trophic prey by animals at all levels of the food web – from zooplankton (Cole et al., 2013), small pelagic fish, and mussels (Digka et al., 2018a) to mesopelagic fish (Wieczorek et al., 2018) and large predators like tuna and swordfish (Romeo et al., 2015). Microplastic ingestion by marine animals can potentially affect animal health and raises toxicity concerns, since plastics can facilitate the transfer of chemical additives and/or hydrophobic organic contaminants to biota (Mato et al., 2001; Rios et al., 2007; Teuten et al., 2007, 2009; Hirai et al., 2011). Humans, as top predators, are also contaminated by MPs (Schwabl et al., 2019). Mussels and small fish that are commonly consumed whole, without removing digestive tracts, where MPs are concentrated, are among the most likely pathways for MPs to embed in the human diet (Smith et al., 2018). Especially regarding marine organisms (i.e. mussels), it is notable that the levels of their contamination have been added to the European database (http://www.ecsafeseafooddbase.eu, last access: 27 July 2020) as an environmental variable of growing concern, reflecting the health status (Marine Strategy Framework Directive, MSFD, Descriptor 10 – Marine Litter; Decision 2017/848/EU) (De Witte et al., 2014; Vandermeersch et al., 2015; Digka et al., 2018a). Today, a series of studies have noted the presence of MPs in mussels' tissue intended for human consumption (Van Cauwenberghe and Janssen, 2014; Mathalon and Hill, 2014; Li et al., 2016, 2018; Hantoro et al., 2019). For instance, in a recent study, Li et al. (2018) sampled mussels from coastal waters and supermarkets in the UK and estimated that a plate of 100 g mussels contains 70 MPs that will be ingested by the consumer. The presence of MPs in mussels has been also demonstrated during laboratory trials in their faeces, intestinal tract (Von Moos et al., 2012; Van Cauwenberghe et al., 2015; Wegner et al., 2012; Khan and Prezant, 2018), and circulatory system (Browne et al., 2008). Other laboratory studies showed several effects of microplastic ingestion in laboratory-exposed mussels, including histological changes, inflammatory responses, immunological alterations, lysosomal membrane destabilization, reduced filtering activity, neurotoxic effects, oxidative stress effects, increase in haemocyte mortality, dysplasia, genotoxicity, and transcriptional responses (reviewed by Li et al., 2019). However, the tested concentrations of MPs in laboratory experiments are frequently unrealistic, being several orders of magnitude higher (2 to 7 orders of magnitude) than the observed seawater concentrations (Van Cauwenberge et al., 2015; Lenz et al., 2016).
Mussels, through their extensive filtering activity, feed on planktonic organisms that have a similar size to MPs (Browne et al., 2007), and considering also their inability to select particles with high energy value (i.e. phytoplankton) during filtration (Vahl, 1972; Saraiva et al., 2011a), they are directly exposed to MPs contamination. Recent studies suggest a positive linear correlation between MPs concentration in mussels and surrounding waters (Capolupo et al., 2018; Qu et al., 2018; Li et al., 2019). The filtering activity of mussels, which directly affects the resulting MPs accumulation, is a complicated process that is controlled by other factors (food availability, temperature, tides etc.).
The purpose of the present work is to study the accumulation of MPs in mussels and reveal relations between the accumulated concentrations in mussels' soft parts and environmental features. In this context, an accumulation model was developed based on dynamic energy budget theory (DEB; Kooijman, 2000) and applied in two different regions, in two different modes of life (wild and cultivated): in the North Sea (Mytilus edulis (M. edulis), wild) and in the northern Ionian Sea (Mytilus galloprovincialis (M. galloprovincialis), cultivated). DEB theory provides all the necessary detail to model the feeding processes and aspects of the mussel metabolism, taking into account the impact of the environmental variability on the simulated individual. Apart from modelling the growth of bivalves (Rosland et al., 2009; Sarà et al., 2012; Thomas et al., 2011; Saraiva et al., 2012; Hatzonikolakis et al., 2017; Monaco and McQuaid, 2018), DEB models have been used to study other processes as well, such as bioaccumulation of PCBs (polychlorinated biphenyls) and POPs (persistent organic compounds) (Zaldivar, 2008), trace metals (Casas and Bacher, 2006), and the impact of climate change on individual's physiology (Sarà et al., 2014). However, to our knowledge this is the first time that a DEB-based model is used to assess the uptake and excretion rates of MPs in mussels.
2.1 Study areas and field data
The North Sea is a marginal sea on the continental shelf of north-western Europe with a total surface area of 850 000 km2 and is bounded by the coastlines of nine countries. The sea is shallow (mean depth 90 m), getting deeper towards the north (up to 725 m), and the semidiurnal tide (tidal range 0–5 m) is the dominant feature of the region (Otto et al., 1990). Major rivers, such as the Rhine, Elbe, Weser, Ems, and Thames discharge into the southern part of the sea (Lacroix et al., 2004), making this area a productive ecosystem. In this study, the area is limited to along the French, Belgian, and Dutch North Sea coast (50.98–51.46∘ N, 1.75–3.54∘ W). This is located close to harbours, where shipping, industrial, and agricultural activity is high, putting considerable pressure on the ecological systems of the region (Van Cauwenberghe et al., 2015).
The MPs concentration in mussels' tissue and seawater that were used to validate and force the model respectively at its North Sea implementation were derived from Van Cauwenberghe et al. (2015). Van Cauwenberghe et al. (2015) examined the presence of MPs in wild mussels (M. edulis) and thus collected both biota and water at six sampling stations along the French, Belgian, and Dutch North Sea coast in late summer of 2011. M. edulis (mean shell length: 4±0.5 cm and wet weight (w.w.): 2±0.7 g) and water samples were randomly collected in the local breakwaters, in order to assess the MPs concentration in the organisms and their habitat. MPs were present in all analysed samples, both organisms and water. Seawater samples (N=12) had MPs (<1 mm) on average 0.4±0.3 particles L−1 (range: 0.0–0.8 particles L−1), and M. edulis contained on average 0.2±0.3 particles g−1 w.w. (or 0.4±0.3 particles per individual) (Van Cauwenberghe et al., 2015). The size range of MPs found within the mussels was 20–90 µm.
The northern Ionian Sea is located in the transition zone between the Adriatic and Ionian Sea. The long and complex coastline presents a high diversity of hydrodynamic and sedimentary features. Rivers discharging into the northern Ionian Sea include Kalamas/Thyamis (Greece) and Butrinto (Albania) (Skoulikidis et al., 2009; Vlachogianni et al., 2017), making the area suitable for aquaculture. Small farming sites and shellfish grounds are operating in Thesprotia (northwestern Ionian Sea) (Theodorou et al., 2011). The main source of marine litter inputs in the area originates from anthropogenic activities that mainly include shoreline tourism and recreational activities, poor wastewater management, agriculture, fisheries, aquacultures, and shipping (Vlachogianni et al., 2017; Digka et al., 2018a). According to Politikos et al. (2020), the area around the Corfu island (northern Ionian Sea) is characterized as a retention area of litter particles probably due to the prevailing weak coastal circulation. Furthermore, a northward current on the east Ionian Sea facilitates the transfer of litter particles towards the Adriatic Sea, which has been characterized as a hotspot of marine litter and one of the most affected areas in the Mediterranean Sea (Pasquini et al., 2016; Vlachogianni et al., 2017; Liubartseva et al., 2018; Politikos et al., 2020).
The field data used to validate the model output in the N Ionian Sea were obtained from Digka et al. (2018a, b). In the framework of the “DeFishGear” project, mussels (M. galloprovincialis) were collected by hand from a longline-type mussel culture farm in Thesprotia (39.606567∘ N, 20.149421∘ E), in summer 2015 (end of June) at a sampling depth up to 3 m (Digka et al., 2018a). The average MPs accumulation was calculated from a total population of 40 mussels originated from the farm, with 18 of them were found to be contaminated with MPs (46.25 %). The average load of MPs (size <1 mm) per mussel (mean shell length 5.0±0.3 cm) was 0.9±0.2 particles per individual, and the size of MPs found in the mussel's tissue ranged from 55 to 620 µm. Both clean and contaminated mussels were included in the calculated mean value in order to represent the mean state of the contamination level for the individual inhabiting the study area.
The seawater concentration of MPs for the N Ionian Sea implementation was obtained from Digka et al. (2018b) and the DeFishGear project results (http://www.defishgear.net/project/main-lines-of-activities, last access: 27 July 2020). In total, 12 manta net tows were conducted in the region, collecting a total number of n1=2027 particles on October 2014 and n2=1332 on April 2015, leading to an average of 280 particles per tow with size <1 mm and >330 µm (Digka et al., 2018b). In order to estimate the mean MPs concentration in the region, expressed as particles per volume, the dimensions of the manta net (W: 60 cm, H: 24 cm, rectangular frame opening; mesh size 330 µm) and the sampling distance of each tow (∼2 km) were used by multiplying the sample surface of the net by the trawled distance in metres (Maes et al., 2017), which resulted in a mean MPs concentration of 1.17 particles m−3 (233 333 particles km−2). Moreover, in the wider region of the Adriatic Sea, Zeri et al. (2018) found a mean density of 315 009±568 578 particles km−2 (1.58±2.84 particles m−3), out of which 34 % were sized <1 mm. A relatively high value of standard deviation (1 order of magnitude higher than the mean value) is adopted (0.0012±0.024 particles L−1), considering that the mussel farm is established in an enclosed gulf and close to the coast, since, according to Zeri et al. (2018), the abundance of MPs is 1 order of magnitude higher in inshore (<4 km) compared to offshore waters (>4 km). Furthermore, it may be assumed that the adopted range (standard deviation is also multiplied by a factor of 2) includes also the smaller particles sized between 50 and <330 µm, which have been found in mussel tissue (Digka et al., 2018a) but were overlooked during the seawater sampling due to the manta net's mesh size (>330 µm). According to Enders et al. (2015) the relative abundance of small particles (50–300 µm) compared to particles larger than 300 µm is approximately 50 %.
2.2 DEB model description
In the present study, a DEB (Kooijman, 2000, 2010) model is used as basis to simulate the accumulation of MPs by mussels. In DEB theory (Kooijman, 2000), the energy assimilated through food by the simulated individual is stored in a reserve compartment from where a fixed energy fraction κ is allocated for growth and somatic maintenance, with a priority for maintenance. The remaining energy (1−κ) is spent on maturity maintenance and reproduction. The individual's condition is defined by the dynamics of three state variables: energy reserves E (joules), structural volume V (cm3), and energy allocated to reproduction R (joules). The energy flow through the organism is controlled by the fluctuations of the available food density and temperature characterizing the surrounding environment.
The DEB model implemented here is an extended version of the model described in Hatzonikolakis et al. (2017), where the growth of the Mediterranean mussel is simulated taking into account only the assimilation rate of the individual. Since the present study focuses on the MPs accumulation, it is crucial to include a detailed representation of the mussel's feeding mechanism. In this context, the DEB model was extended by including the clearance (Cr), filtration (), and ingestion () rates of the mussel, following Saraiva et al. (2011a), assuming that all parameters referred to as silt (or inedible particles) are applicable also to MP particles. In this approach, a pre-ingestive selection occurs between filtration and ingestion, returning the rejected material in the water through pseudofaeces (Jpfi). Consequently, energy is assimilated through food, and the non-assimilated particles are excreted through the faeces production (Jf). The model's equations, variables, and parameters are shown in Tables 1, 2, and 3 respectively. The scaled functional response f (Eq. 5, Table 1), which regulates the assimilation rate, is modified following Kooijman (2006) to include an inorganic term representing the non-digestible matter, i.e. microplastics: and ), where Y and Yk are the concentration of MPs, converted from particles per litre to grams per cubic metre (Everaert et al., 2018) and the half-saturation coefficient of inorganic particles here represented by MPs (g m−3) respectively. Thus, the assimilation rate that is regulated by f is decreasing when the concentration of MPs is increased. The same approach is followed by other authors who considered inedible particles in the mussel's diet (Ren, 2009; Troost et al., 2010). During the filtration process the same clearance rate for all particles is used ), representing the same searching rate for food that depends on the organism maximum capacity ) and environmental particle concentrations (Vahl, 1972; Widdows et al., 1979; Cucci et al., 1989). During the ingestion process the mussel is able to selectively ingest food particles and reject inedible material, in order to increase the organic content of the ingested material (Kiørboe and Møhlenberg, 1981; Jørgensen et al., 1990; Prins et al., 1991; Maire et al., 2007; Ren, 2009; Saraiva et al., 2011a). This selection is reflected by the different binding probabilities adopted for each type of particle (ρ1 for algae particles and ρ2 for inorganic particles, i.e. MPs; see Eq. 14 and Table 3). The equations representing the feeding processes handle each type of particle separately, while there is interference between the simultaneous handling of different particle types (Eqs. 12–14, Table 1) (Saraiva et al., 2011a). Finally, during the assimilation process, suspended matter (i.e. MPs) that the mussel is not able to assimilate due to its different chemical composition from the reserve compartment (Saraiva et al., 2011a) or incipient saturation at high algal concentrations (Riisgård et al., 2011) results in the faeces production (Eq. 16, Table 1).
Table 1Dynamic energy budget model: equations. See Table 2 for model variables, Table 3 for parameters, and Table 5 for initial values.
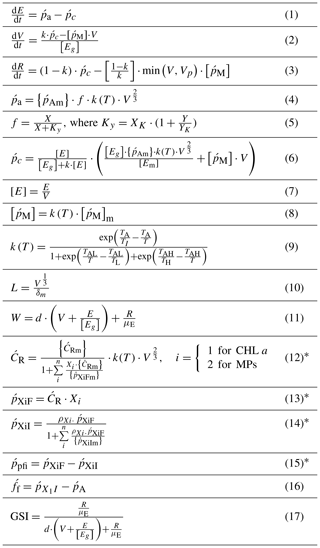
* Notation refers to feeding equations handling each type of suspended matter separately (i=1 for algae and i=2 for microplastics) where unit transformation is applied when it is necessary (see Table 3).
2.3 Microplastics accumulation submodel
With the DEB model as a basis, a submodel describing the MPs accumulation by the mussel was developed, assuming that the presence of MPs in the ambient water does not cause a significant adverse effect on the organisms' overall energy budget, in accordance with laboratory experiments, conducted in mussel species (Van Cauwenberghe et al., 2015, for Mytilus edulis; Santana et al., 2018, for the mussel Perna perna). Additionally, it was assumed that the mussel filtrates MPs present in the water, without the ability of selecting between the high-energy-valued particles and the MPs during the filtration process (Van Cauwenberghe et al., 2015; Von Moos et al., 2012; Browne et al., 2008; Digka et al., 2018a among others). The uptake of MPs from the environment is taken into account through the process of clearance/filtration rate, while the excretion of the contaminant is derived from two processes: (i) pseudofaeces production and (ii) faeces production. The resulting MPs accumulation is influenced by external environmental factors (MPs concentration, food availability, temperature) and internal biological processes (clearance, filtration, ingestion, growth). The following differential equation describes the change of the individual MPs accumulation (C, particles per individual), taking into account the processes mentioned above:
where is the clearance rate for water (L h−1), containing a concentration of MPs Cenv (particles L−1). The terms of and represent the elimination rate of MPs through pseudofaeces (particles h−1) and the nondimensional rate of faeces production with respect to the ingestion rate respectively (see Table 1, Eqs. 15–16). The parameter kf represents the post-ingestive selection mechanism utilized by the mussel to incorporate indigestible material (i.e. MPs) into faeces and was calibrated using the available field data of mussel MPs accumulation from both study areas (Table 3). A mussel is able to discriminate among particles in the gut based on size, density and chemical properties of the particles (i.e. between microalgae and inorganic material) and thus to eliminate them through faeces (Ward et al., 2019a, and references therein). In this context, the pseudofaeces production incorporates the rejected MPs prior to the ingestion, while the faeces production includes MPs that are rejected along with the food particles that are not assimilated by the mussel. The model's time step has been set to 1 h in order to capture the dynamics of the rapidly changing processes, such as feeding and excretion.
2.4 Environmental drivers
Besides MPs concentration in the seawater, the DEB model is forced by sea surface temperature (SST) and food availability, represented by chlorophyll a concentrations (CHL a, an index of phytoplankton biomass). M. edulis has been demonstrated to filter suspended particles greater than 1 µm, a size class that includes all of the phytoplankton, zooplankton, and much of the detritus (Vahl, 1972; Møhlenberg and Riisgård, 1978; Saraiva et al., 2011a; Strohmeier et al., 2012), including even aggregated picoplankton-size particles (i.e. marine snow) (Kach and Ward, 2007; Ward and Kach, 2009). CHL a has been considered the most reliable food quantifier for the calculation of DEB shellfish parameters (Pouvreau et al., 2006; Sarà et al., 2012; Hatzonikolakis et al., 2017, and references therein). Hatzonikolakis et al. (2017) have tested the performance of the model, considering also particulate organic carbon (POC) in the mussel's diet, which, however, did not have an important impact on the model's skill against field data in the Mediterranean Sea study areas. This outcome agrees with Troost et al. (2010) demonstration that POC contributes to the mussel's diet when CHL a concentrations are low in the southwest of Netherlands. Thus, in the present study, only CHL a is considered as the available food source for mussels originated from the southern North Sea and the northern Ionian Sea. For both study areas SST and CHL a are derived from daily satellite data, a method also used by other authors (i.e. Thomas et al., 2011; Monaco and McQuaid, 2018).
In the North Sea, SST data were obtained from daily satellite images provided by Copernicus Marine Environmental Monitoring Service (CMEMS) at 0.04∘ spatial resolution. CHL a data obtained from the Globcolour daily multi-sensor product provided by CMEMS at 1 km spatial resolution, based on the OC5 algorithm of Gohin et al. (2002) (http://marine.copernicus.eu/, last access: 27 July 2020, generated using CMEMS Products, production centre ACRI-ST). The environmental forcing data (SST, CHL a) were averaged over the study area (51.08–51.44∘ N, 2.19–3.45∘ E), covering the period 2007–2011 (5 years), in order to realistically simulate the wild mussel's growth harvested in late summer 2011 (Van Cauwenberghe et al., 2015). It is notable that the study area of the North Sea belongs to Case II waters (coastal region), where algorithms tend to overestimate CHL a concentrations. In optically complex Case II waters, CHL a cannot readily be distinguished from particulate matter and/or yellow substances (dissolved organic matter), and so global chlorophyll algorithms are less reliable (IOCCG, 2000). However, the CHL a dataset that was used was found in good agreement with available in situ data from the ICES database (https://www.ices.dk/data/Pages/default.aspx, last access: 27 July 2020) for the specific study area and time period (Fig. 1), showing a relatively smaller bias and better time–space coverage, as compared with other tested remote sensing datasets (not shown) (i.e. regional chlorophyll product available for the North West Shelf Seas in the CMEMS catalogue, http://marine.copernicus.eu/, last access: 27 July 2020).
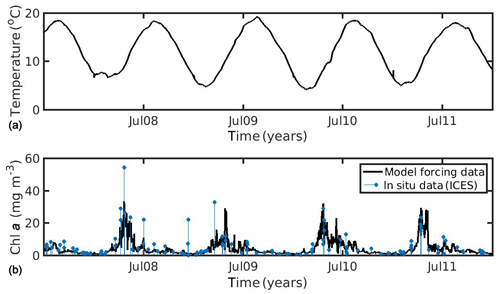
Figure 1Environmental data used for the forcing of the dynamic energy budget model (DEB) in the North Sea simulation, showing (a) temperature and (b) chlorophyll a concentration against in situ data from the ICES database.
In the northern Ionian Sea, daily satellite SST data were also obtained from the CMEMS database for the Mediterranean Sea with 0.04∘ spatial resolution, while CHL a daily data were derived from the Globcolour multi-sensor (i.e. SeaWiFS, MERIS, MODIS, VIIRS, and OLCI a) merged product (http://globcolour.info last access: 27 July 2020) at 1 km spatial resolution based on the OC5 algorithm suitable for coastal regions (Gohin et al., 2002). The forcing data were averaged over the study area (39.49–39.65∘ N, 20.09–20.23∘ E) covering the period 2014–2015 (2 years), when the cultured mussel is ready for the market. The chosen CHL a dataset was found preferable, as compared with other available remote sensing datasets (i.e. CMEMS chlorophyll product for Mediterranean Sea), since it presented a better spatial and temporal coverage (El Hourany et al., 2019; Garnesson et al., 2019) and a slightly lower error, as compared with the very few available in situ data in the study area (not shown). Unfortunately, these were very scarce, and therefore an extended comparison between remote and in situ data could not be conducted. Satellite data have facilitated large-scale ecological studies by providing maps of phytoplankton functional types and sea surface temperature (Raitsos et al., 2005, 2008, 2012, 2014; Palacz et al., 2013; Di Cicco et al., 2017; Brewin et al., 2017). The daily environmental forcing data are shown in Figs. 1 and 2 for the North Sea and the N Ionian Sea respectively. The two coastal environments present some important differences regarding both CHL a and SST. Specifically, in the N Ionian Sea, CHL a is relatively low (annual mean ∼0.88 mg m−3) and peaks during winter (maximum ∼2.64 mg m−3 at December 2014), while in the North Sea CHL a is about 4 times higher (annual mean 4.25 mg m−3), peaking in April every year (maximum range 29.44–33.38 mg m−3), as soon as light availability reaches a critical level (Van Beusekom et al., 2009). The higher productivity during the spring season in the North Sea is related with the nutrient inputs from the English Channel, the North Atlantic, and particularly the river discharge of nutrient-rich waters along the Belgian–French–Dutch coastline, which peaks earlier, during winter period (Van Beusekom et al., 2009). The SST peaks during August in both areas (Figs. 1 and 2) but is significantly higher in the N Ionian Sea (maximum 28.8 ∘C), as compared to the North Sea (maximum 18–19.3 ∘C).
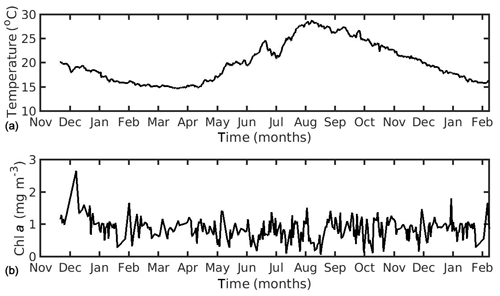
Figure 2Environmental data used for the forcing of the dynamic energy budget model in the northern Ionian Sea simulation, showing (a) temperature and (b) chlorophyll a concentration.
The environmental concentration of MPs, Cenv (particles L−1), was also obtained at a daily time step as randomly generated values of the Gaussian distribution that is determined by the mean value and standard deviation of the observed field data (0.4±0.3 particles L−1, North Sea; Van Cauwenberghe et al., 2015; 0.0012±0.024 particles L−1, N Ionian Sea, Digka et al., 2018a). Considering that these values originate from surface waters and that mussels live in the near-surface layer (0–5 m), Cenv is estimated as a mean value of the upper layer with the methods described by Kooi et al. (2016), which studied the vertical distribution of MPs, considering an exponential decrease with depth. Specifically, in the N Ionian Sea, mussels were collected from a depth up to 3 m (Digka et al., 2018a), while in the North Sea (Van Cauwenberghe et al., 2015), there is no information, and thus a maximum depth of 5 m is adopted. In the North Sea simulation, the effect of tides is taken into account by considering that the mussel originated from the intertidal zone is submerged 12 h during the day (Van Cauwenberghe et al., 2015). In the N Ionian Sea simulation, tides are not considered, given the very small tide amplitude (few centimetres) in the Mediterranean (i.e. Sarà et al., 2011; Hatzonikolakis et al., 2017), and thus the cultured mussel is assumed permanently submerged. In situ hourly tide data (2007–2011) from the coastal zone of the region (Dunkerque station 51.04820∘ N, 2.36650∘ E) obtained from Coriolis and Copernicus data provider (http://marine.copernicus.eu, http://www.coriolis.eu.org, last access: 28 July 2020) showed that mussels experience alternating periods of aerial exposure and submergence at approximately every 6 h (two high and two low tides). During aerial exposure, the model suspends the feeding processes (Sarà et al., 2011) and simulates metabolic depression (Monaco and McQuaid, 2018) where the Arrhenius thermal sensitivity equation (Eq. 9) is corrected by a metabolic depression constant (Md=0.15), a value representative for M. galloprovincialis and here applied also for M. edulis. In the present study, the mussel's body temperature change during low tide is ignored, inducing a model error. The mussel's body temperature (i.e. surrounding water temperature for submerged mussels) during air exposure depends on many factors, such as solar radiation, air temperature, wind speed and wave height, according to studies investigating the temperature effect on intertidal mussels (Kearney et al., 2010; Sarà et al., 2011). However, the present study aims to primarily examine the MPs accumulation, and thus the intertidal mussel's body temperature was not thoroughly examined. Nonetheless, the time that the mussel is able to filter, ingest, and excrete the suspended matter (i.e. food and MP particles) and the effect on the mussel's growth through the modified relation of k(T) are included, since the assimilation process occurs whether the mussel is submerged or not (Kearney et al., 2010).
2.5 Parameter values
Most of the DEB model parameters were obtained from Van der Veer et al. (2006) and refer to the blue mussel M. edulis in the northeast Atlantic (see Table 3 for the exceptions). This assumption has also been adopted in previous studies which showed that this parameter set for M. edulis applies also for M. galloprovincialis (i.e. Casas and Bacher, 2006; Hatzonikolakis et al., 2017). The half-saturation coefficient Xk represents the density of food at which the food uptake rate reaches half of its maximum value and should be treated as a site-specific parameter (Troost et al., 2010; Pouvreau et al., 2006). In order to estimate the value of Xk, a different approach was followed for each study area.
For the North Sea simulation, Xk was tuned so that the simulated individual has the recorded size at the corresponding estimated age (Van Cauwenberghe et al., 2015) growing with the representative growth rates of wild M. edulis at the region (Saraiva et al., 2012; Sukhotin et al., 2007). For the N Ionian Sea simulation, an alternative method was adopted, aiming to generalize the DEB model to overcome the problem of site-specific parameterization. The DEB model was tuned against literature field data for cultured mussels originated from different areas in the Mediterranean and Black seas, where the average CHL a concentration ranged between 1.0 and 5.0 mg m−3, and one Xk value was found for each area. The four areas used, their characteristics, and the corresponding value of Xk adopted are shown in Table 4. These values of Xk are related to the prevailing CHL a concentration of each area ([CHL a]) through three different functions: linear: ; exponential: ; and power: . The curve fitting app of MATLAB (MATLAB R2015a) was used for the determination of a,b, and c of each function, taking into account the 95 % confidence level. The score of each function regarding the somatic/mussel growth simulation in all four regions is tested through target diagrams (Jolliff et al., 2009) by computing the bias and unbiased root-mean-square deviation (RMSD) between field and simulated data of all four regions, and the function with the best score is adopted. A similar approach was followed by Alunno-Bruscia et al. (2011) for the oyster Crassostrea gigas in six Atlantic ecosystems, expressing the Xk as a linear function of food density (e.g. phytoplankton). Unfortunately, the approach described for the N Ionian Sea simulation could not be applied in the North Sea, as the limited amount of growth data from the literature for wild M. edulis in similar environments did not permit a statistically significant fit of a similar function, Xk=f(chl a).
2.6 Simulation of reproduction–initialization of the model
The reproductive buffer (R) is assumed to be completely emptied at spawning (R=0) (Sprung, 1983; Van Haren et al., 1994). In order to simulate mussel spawning, the gonado-somatic index (GSI) defined as gonad dry mass over total dry flesh mass was computed at every model's time step (Eq. 17 Table 1; the water content of the fresh tissue mass was assumed 80 % according to Thomas et al., 2011). Spawning was induced by a critical value of GSI (GSIth, Table 3) and a minimum temperature threshold (Tth) at each study area, obtained from the literature. In the North Sea implementation, Tth was set at 9.6 ∘C (Saraiva et al., 2012), while in the N Ionian Sea, at 15 ∘C (Honkoop and Van der Meer, 1998). This kind of formulation for the spawning event in bivalves has been used in previous studies (i.e. Pouvreau et al., 2006; Troost et al., 2010; Thomas et al., 2011; Monaco and McQuaid, 2018). The simulated abrupt losses of the mussel's tissue mass correspond to spawning events, and the model's prediction was compared with the available literature data regarding the spawning period in each study area. Theodorou et al. (2011) demonstrated that the spawning events occur during winter for M. galloprovincialis in the mussel farms of Greece, while in the North Sea the spawning period for M. edulis is extended from the end of April until the end of June (Sprung, 1983; Cardoso et al., 2007).
In both areas, the model was initialized so that the simulated individual is in the juvenile phase (V<Vp; Table 3) and the reproductive buffer can be considered to be empty (R=0) (Thomas et al., 2011). As stated by Jacobs et al. (2015) amongst others, juvenile mussels (M. edulis) range between 1.5 and 25 mm in size. Specifically, in the North Sea the settlement of mussel larvae (M. edulis) takes place in June and the juveniles grow to a maximum size of 25 mm within 4 months (Jacobs et al., 2014). In the N Ionian Sea, the operating mussel farms follow the life cycle of M. galloprovincialis, starting the operational cycle each year by dropping seed collectors from late November until March, and the juvenile mussels grow up to 6–6.5 cm after approximately 1 year according to the information obtained from the local farms in the region and Theodorou et al. (2011). The initial fresh tissue mass was distributed between the structural volume (V) and reserves energy (E). Energy allocated to those two compartments was firstly constrained by the initial length (L), and then energy allocated to V was in Eq. (10) (Table 1). The initial value of E was set so that the simulated individual has an initial weight that corresponds to the juvenile phase (V<Vp) (Table 5). Finally, for both model implementations, the initial accumulation of MPs in the mussel's tissue (C) was set to zero.
2.7 Simulation runs
The DEB-accumulation model simulates at an hourly basis the growth and MPs accumulation of the wild mussel from the North Sea and the cultured mussel from the N Ionian Sea. Initially, a model run is performed at each study area during the periods from July 2007 to August 2011 (4 years) for the North Sea simulation and late November 2014 to January 2016 (∼1 year) for the N Ionian Sea simulation. Additionally, the inverse simulations were performed in order to evaluate the depuration phase of both cultured and wild mussel, by setting the environmental MPs concentration equal to zero (Cenv=0), after a period of 1 year simulation at the N Ionian Sea, when the cultured mussel has the appropriate size for market, and after 4 years in the North Sea, when literature field data are available (Van Cauwenberghe et al., 2015). In this simulation, the mussel's gut clearance is achieved by the excretion of MPs through faeces (third term of Eq. 18), and thus it is necessary to maintain the existence of food in the mussel's environment in order to ensure that the feeding–excretion processes will occur.
Furthermore, to examine the model's uncertainty related to the environmental MPs concentration, a series of 15 and 13 simulations were performed in the North Sea and N Ionian Sea respectively, adopting different constant values of Cenv within the observed range of each area. Finally, the effect of the environmental forcing data and some model parameters on the resulting MPs accumulation by both mussels was explored through sensitivity experiments. These were used to derive a new function that predicts the level of MPs pollution in the environment.
2.8 Sensitivity tests and regression analysis
The effect of the environmental data (CHL a, temperature, Cenv) and two parameters representative of mussel's growth (Xk, Yk) on the MPs accumulation by the mussel for each study area was examined through sensitivity experiments with the DEB-accumulation model. Each variable (CHL a, T, Cenv) and parameter (Xk, Yk) was perturbed by ±10 % to examine its effect on the simulated MPs accumulation, and the results of each run were analysed using a sensitivity index (SI). SI calculates the percentage change in the mussel's MPs accumulation; (%), where n is the simulated time steps, is the MPs accumulation predicted with the standard simulation at time t, and is the MPs accumulation with a perturbed variable/parameter at time t; for details see Bacher and Gangnery (2006). The same method has been also applied to other studies, which examined the model's sensitivity for specific variables/parameters regarding the mussel growth (Casas and Bacher, 2006; Rosland et al., 2009; Béjaoui-Omri et al., 2014; Hatzonikolakis et al., 2017). In order to also examine the effect of tides, in the North Sea implementation, the sensitivity experiments were conducted twice as follows: the first time assuming that the mussel is permanently submerged and the second time assuming that the mussel is periodically exposed to the air.
Preliminary sensitivity experiments showed that the MPs accumulation is highly dependent on the prevailing conditions regarding the CHL a, temperature, and Cenv and the mussel's growth that is regulated by the half-saturation coefficient (Xk). Therefore an attempt was made using the model's output to describe the MPs accumulation as a function of these variables through a custom regression model as follows:
where y (particles per individual) is the response variable and represent the predicted MPs accumulation by the mussel; W (g) is the mussel's fresh tissue mass, T (K) is the sea surface temperature; CHL a and Cenv are the concentrations of chlorophyll a and MPs in the water respectively, which are the predictor variables. The values of coefficients b1, b2, b3, and b4 are calculated using the nonlinear regression function (nlinfit, MATLAB R2015a), which attempts to find values of the parameters b that minimize the least-squared differences between the model's MPs accumulation output C and the predictions of the regression model [CHL a], Cenv, b).
The ultimate aim of this analysis, once coefficients are determined, is to use Eq. (19) to obtain the environmental MPs concentration:
which could be a very useful tool to predict the MPs concentration in the environment, when all involved variables are known (mussel's accumulated MPs, C; wet weight, W; temperature, T; and CHL a), using the mussel as a potential bioindicator (Li et al., 2016, 2019). The score of this custom model was tested by applying Eq. (20) in our study areas and six more areas around the UK, where information on a mussel's wet weight and both the mussels' and environment's MPs load is available (Li et al., 2018). CHL a and temperature, which were not included in Li et al. (2018), were obtained from daily satellite images (same source as in the North Sea; see Sect. 2.4), covering the period that the mussels were harvested (Li et al., 2018).
3.1 Growth simulations
The growth simulations of M. edulis and M. galloprovincialis for the North Sea and the N Ionian Sea are shown in Figs. 3 and 4 respectively. In the North Sea implementation, Xk was tuned to a constant value: Xk=8 mg m−3 (±1.5 mg m−3). The fitted value was higher, as compared to the one (Xk=3.88 mg m−3) used by Casas and Bacher (2006) in productive areas of the French Mediterranean shoreline (average CHL a concentration: 1.45 mg m−3; maximum peak at 20 mg m−3), as a consequence of the higher productivity in the North Sea (average CHL a concentration: 4.25 mg m−3; maximum peak at ∼33.40 mg m−3). The high value of Xk could also be explained by the presence of inedible particles (i.e. MPs) that led to lower-quality food in the mussel's diet compared with an assumed clean-from-inedible-particles environment (Kooijman, 2006; Ren, 2009). In the present study the inedible particles (i.e. MPs) have been incorporated in the mussel's diet through the modified relation of the functional response f (Eq. 5, Table 1), which regulates the assimilation rate and thus the mussel's growth. However, the DEB model applied at the French site, did not account for inedible particles in the mussel's food. Furthermore, it has been reported that wild mussels grow considerably slower than farmed mussels (∼1.7 times) (Sukhotin and Kulakowski, 1992), and thus, a higher value of Xk promotes less mussel growth, which is the case for the North Sea mussel. The simulated mussel shell length after 4 years, in August, is 4.35 cm, and the fresh tissue mass is 1.87 g, in agreement with Van Cauwenberghe et al. (2015) and other studies conducted on wild mussels (Sukhotin et al., 2007; Saraiva et al., 2012; MarLIN, 2016). In particular, Saraiva et al. (2012) found that after 16 years of simulation, the wild mussel of the Wadden Sea (North Sea) is 7 cm long, while according to Bayne and Worral (1980) a mussel with shell length 4 cm corresponds to the age of 4 years, in agreement with the current study. The simulated growth presents a strong seasonal pattern, being higher during the spring and summer season, as compared to autumn and winter, which is consistent with the seasonal cycle of temperature and CHL a concentration, for a typical year in the region (Fig. 1). The increase in food availability and temperature during spring (April) results in high mussel growth for a 4-month period, while the decrease in CHL a from summer until the end of the year, in conjunction with the temperature decrease in autumn, results in a less mussel growth. Spawning events that occurred in late April–early May (30 April–2 May) each year are responsible for the sharp decline in a mussel's fresh tissue mass, shown in Fig. 4 (Handa et al., 2011; Zaldivar, 2008), which is in agreement with the literature (Sprung, 1983; Cardoso et al., 2007; Saraiva et al., 2012). The predicted weight loss due to spawning was around 7 % at the first year of simulation, while the second, third, and fourth year the percentage of weight loss increased gradually to 8.3 %, 12.6 %, and 14.4 % respectively. Bayne and Worral (1980) demonstrated that the weight losses on spawning for individuals of 1 g weight vary between 2.1 % and 39.8 %, presenting a weight-specific increase with size.
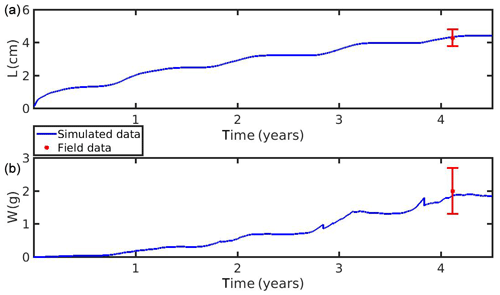
Figure 3(a) Simulated mussel shell length (L) and (b) fresh tissue mass (W) against North Sea data (red star: mean ± SD), using chlorophyll a, X=[CHL a], in the mussel diet.
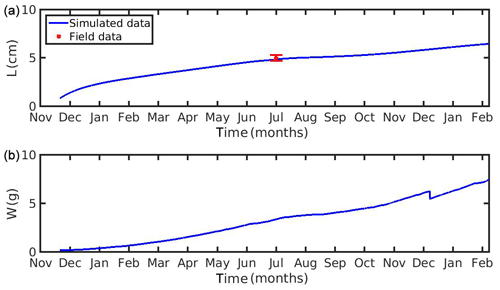
Figure 4(a) Simulated mussel shell length (L) and (b) fresh tissue mass (W) against North Sea data (red star: mean ± SD), using chlorophyll a, X=[CHL a], in the mussel diet.
In the N Ionian Sea implementation, Xk is applied as a function of CHL a concentration through the method described in Section 2.5. The target diagram showing the performance of each tested function (linear: , where a=0.959 and ; exponential: , where a=0.2 and b=0.567; power: , where a=0.01, b=3.529 and c=0.480) is shown in Fig. 5. The linear and power function of Xk present good skill, with the power function leading to the most successful simulation of the cultured mussel's growth in all four areas (diagram marks for mussel length and fresh tissue mass are closer to the target's centre). The power function applied in the N Ionian Sea resulted in a mussel's shell length of 5.8 cm and fresh tissue mass of 5.92 g after 1 year of simulation, in agreement with Theodorou et al. (2011). The spawning event occurred at the beginning of December (Theodorou et al., 2011) and was illustrated by a 12.6 % tissue mass decline.
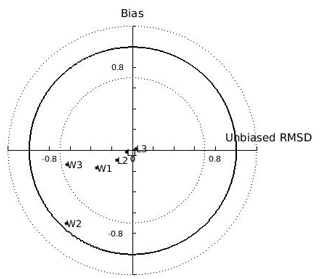
Figure 5Target diagram of simulated shell length (L) and fresh mass tissue weight (W) against field data from the Thermaikos and Maliakos gulfs (eastern Mediterranean Sea), Black Sea, and Bizerte Lagoon (southwestern Mediterranean Sea), using the power (L1,W1), exponential (L2,W2), and linear (L3,W3) functions of the half-saturation coefficient. The model bias is indicated on the y axis, while the unbiased root-mean-square deviation (RMSD) is indicated on the x axis.
3.2 Microplastics accumulation and depuration phase
The hourly simulated MPs accumulation by the mussel in the North Sea and N Ionian Sea are shown in Figs. 6 and 7 respectively. Calibration of the parameter kf (1.2 d−1) led to a model which was well fitted to the observed MPs accumulation in the mussel of both study areas. In the North Sea, a 4-year-old wild mussel (L=4.35 cm, W=1.87 g) contains 0.53 particles per individual in August, within the range value found by Van Cauwenberghe et al. (2015) (0.4±0.3 particles per individual), although the model overestimated the data range, reproducing a seasonal increase that was not observed. This is most likely due to the fact that Van Cauwenberghe et al. (2015) allowed a 24 h clearance period before analysing the mussels' tissue for MPs, resulting in slightly lower MPs accumulation than the model's prediction. The MPs egested through faeces by the 4-year-old mussel after 24 h were 0.2±0.2 particles per individual (Van Cauwenberghe et al., 2015), which agree also with model's output (0.3 particles per individual, Fig. 8) regarding the depuration phase and could compensate for the observed difference in the mussel's MPs load between the simulated and field data. In the N Ionian Sea, the simulated MPs accumulation by the cultured mussel with L=4.85 cm and W=3.33 g was 0.91 particles per individual at the end of June, in agreement with field observations obtained from Digka et al. (2018a) (0.9±0.2 particles per individual). Overall, the developed model simulated the MPs accumulation by both mussels in the two different areas, using the same parameter set (see Table 3 for the exceptions), under the assumption that parameters referred to as silt particles (i.e. inedible particles) may also be used to describe the MPs accumulation. Both simulations were in good agreement with the available field data, with a small deviation for the North Sea. This may lead to the assumption that mussels present a common behaviour against all inedible particles. In the model's results, based on the uptake and excretion rates of MPs by the mussels in both study areas, the majority of MPs are rejected through pseudofaeces and fewer through faeces production (not shown). This is in agreement with Woods et al. (2018), which found that most microplastic fibres (71 %) were quickly rejected as pseudofaeces and <1 % excreted in faeces.
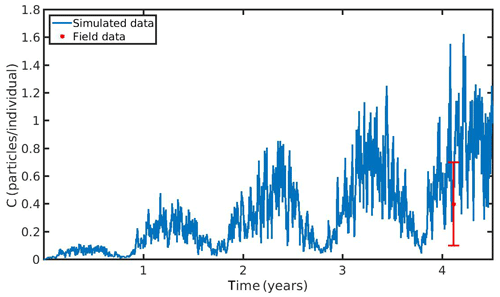
Figure 6Microplastics (MPs) accumulation by the mussel (blue line) against field data (red star: mean ± SD), using daily environmental concentration of MPs (Cenv mean value ± SD: 0.4±0.3 particles L−1) in the North Sea.
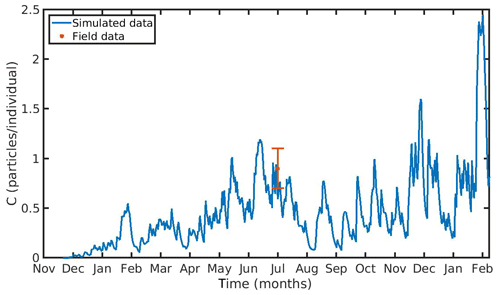
Figure 7 Microplastics (MPs) accumulation by the mussel (blue line) against field data (red star: mean value ± SD), using daily environmental concentration of MPs (Cenv mean value ± SD: 0.0012±0.024 particles L−1) in the northern Ionian Sea.
The small-scale (daily) fluctuations of MPs in the mussel (wild and cultivated) reflect the adopted random variability in the environmental MPs concentration Cenv and the daily fluctuations of the environmental forcing (CHL a, temperature). The large-scale (seasonal) variability follows mainly the variability of the clearance rate. The seasonal variability in the CHL a concentration and temperature greatly determines the variability in the clearance rate and hence the variability in MPs in the individual. Moreover, the model predicts that mussel's energy needs are increased as it grows, and therefore the clearance rate is increased, resulting in higher MPs accumulation.
The simulated time needed to clean the mussel's gut from the MPs load for both areas is shown in Fig. 8. In both areas, the cleaning follows an exponential decay, in agreement with laboratory experiments by Woods et al. (2018). In particular, the model predicts a 90 % mussel's cleaning after 284 h (∼12 d) and 56 h (∼2.5 d) for the N Ionian Sea and North Sea respectively. The cleaning process is more rapid in the North Sea simulation, which can be attributed to the higher CHL a concentration found in this area, leading to increased production of faeces by the mussel and hence faster excretion of the accumulated MPs. In the N Ionian Sea, on the other hand, the rate of the mussel's cleaning is slower, due to the limited food availability.
3.3 Model's uncertainty regarding the environmental microplastics concentration
The MPs concentration in the environment presents a strong variability in both temporal and spatial scales. To examine the model's uncertainty related to the environmental MPs concentration (Cenv), a series of 15 and 13 simulations were performed in the North Sea and N Ionian Sea respectively, adopting different values of Cenv within the observed range of each area. In the North Sea, the adopted Cenv ranged between 0.1 and 0.8 particles L−1 with a step of 0.05 (15 runs), while in the N Ionian Sea Cenv ranged between 0.0012 and 0.0252 particles L−1 with a step of 0.002 (13 runs). The mean seasonal values and standard deviation of the 15 simulations in the North Sea and the mean monthly values and standard deviation of the 13 simulations in the N Ionian Sea were computed and plotted in Figs. 9 and 10 respectively. Each error bar represents the uncertainty in the simulated accumulation at the specific time, related to the environmental MPs concentration.
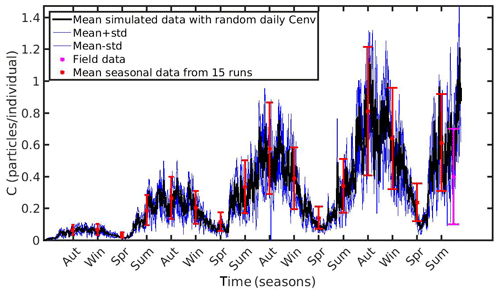
Figure 9Mean seasonally values and standard deviation of microplastics (MPs) accumulation (red error bars: mean value ± SD) by the mussel in North Sea derived from 15 model runs with different constant values of environmental MPs concentration (Cenv range: 0.1–0.8 particles L−1); Mean hourly simulated data (black line) and standard deviation (blue lines) of microplastics accumulation derived from three model runs with stochastic sequences of daily random Cenv values.
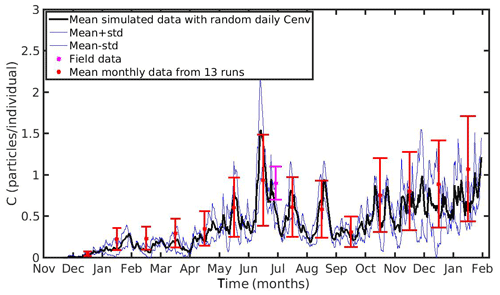
Figure 10Mean monthly values and standard deviation of microplastics accumulation (red error bars: mean value ± SD) by the mussel in northern Ionian Sea derived from 13 model runs with different constant values of environmental MPs concentration (Cenv range: 0.0012–0.024 particles L−1); Mean hourly simulated data (back line) and standard deviation (blue lines) of microplastics accumulation derived from three model runs with stochastic sequences of daily random Cenv values.
In both case studies, the uncertainty of the model appears to increase as the MPs accumulation is increased. As the mussel grows in the North Sea, the mean value and standard deviation of MPs accumulation is increased during the same season every year, illustrating the effect of the mussel's weight. Moreover, the seasonal variability in the MPs accumulation appears to be related with the seasonality of CHL a concentration. This is apparent during each year's spring, when CHL a concentration peaks at its maximum value (∼30 mg m−3; see Fig. 1); the filtration rate is decreased (Riisgård et al., 2003, 2011), leading to lower MPs accumulation by the mussel and thus lower model uncertainty. In the N Ionian Sea, the effect of the mussel's weight is more apparent in the early months (∼6 months), resulting in higher MPs accumulation and model uncertainty as the mussel grows. Afterwards, the seasonality of both CHL a concentration and temperature plays the major role. During summer, when the CHL a concentration is progressively decreased, reaching minimum values (∼0.7 mg m−3), and temperature is increased (>20 ∘C), the filtration rate is significantly decreased or stopped, resulting in lower MPs accumulation and lower model uncertainty. This is in line with studies reporting that the mussel suspends the filtering activity and thus closes its valves until better conditions occur (Pascoe et al., 2009; Riisgård et al., 2011). Overall, the available field data lie within the model's uncertainty, apart from the North Sea case, where the range of field data variability and model uncertainty dot not overlap significantly at the time of the observations.
Moreover, to evaluate the scenario adopted with the set-up of the previous experiments (random Cenv at a daily time step) three additional model runs are performed in each study area, adopting each time different stochastic sequences of daily random Cenv values within the observed range, which is considered to reflect the high spatial and temporal variability in the environmental MPs concentration. The mean value and standard deviation of these “stochastic” runs lie most of the time within the standard deviation of the overall model uncertainty in both case study areas (Figs. 9 and 10).
3.4 Sensitivity and regression analysis results
The results of the sensitivity experiments regarding the MPs accumulation by the mussels are shown in Figs. 11 and 12 for the North Sea and N Ionian Sea respectively. The comparison between the intertidal and subtidal mussel of the North Sea revealed that both +10 % and −10 % perturbation of CHL a and Xk have a slightly lower effect on the MPs accumulation by the intertidal mussel, which is probably attributed to the intermittent feeding periods experienced by the individual due to the tide effect. As far as the temperature effect, both a +10 % and −10 % perturbed value led to higher sensitivity to the MPs accumulation by the intertidal mussel, due to the adopted modified temperature relation during low tide. Especially, if the mussel's body temperature change during air exposure would be considered, the perturbed temperature will probably affect the MPs accumulation even more for the intertidal than the subtidal mussel. The sensitivity of the Cenv to the MPs accumulation when perturbed either +10 % or −10 % is almost the same for the intertidal and subtidal mussel, indicating that the environmental MPs concentration affects similarly both mussels, regardless the continuous or intermittent feeding–excretion process.
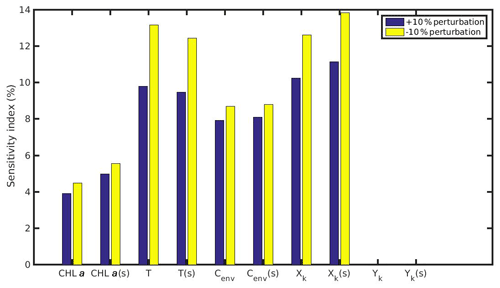
Figure 11Sensitivity index of MPs accumulation for the wild mussel of the North Sea when variables (CHL a, temperature, Cenv) and parameters (Xk,Yk) are perturbed ±10 %. The notation (s) refers to the permanently submerged mussel.
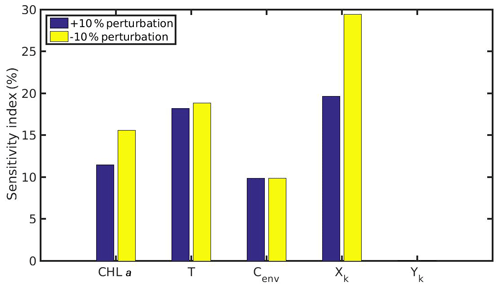
Figure 12Sensitivity index of MPs accumulation for the cultured mussel of the northern Ionian Sea when variables (CHL a, temperature, Cenv) and parameters (Xk,Yk) are perturbed ±10 %.
The comparison between the mussel sensitivity indexes in the N Ionian and the North Sea (in conditions of submergence) study areas reveals some important differences. Generally, most of the perturbed (either +10 % or −10 %) variables and parameters (i.e. CHL a, temperature, Xk) present higher sensitivity to the MPs accumulation by the mussel from the N Ionian Sea. This is attributed to the prevailing environmental conditions and specifically the lower food availability (CHL a) and the higher temperature range in the N Ionian Sea compared to the North Sea, which greatly determine the feeding processes, the mussel's growth, and hence the MPs accumulation. The perturbed Cenv in both study areas appears to affect similarly the MPs accumulation for both mussels (∼10 %), with the small difference (<2 %) probably attributed to the higher abundance of seawater MPs present in the North Sea compared to the N Ionian Sea. Finally, the half-saturation coefficient for the inorganic particles (Yk) has no effect on the MPs accumulation of both North Sea and N Ionian Sea mussels, indicating that the amount of inedible particles (i.e. MPs) is relatively low in both areas, and thus the Yk does not affect the way that the organic particles are being ingested (Kooijman, 2006). According to Ren (2009), when the inorganic matter is low, the K(y) (Eq. 5; Table 1) is approximately equal to Xk, and then Yk is the least sensitive parameter for the ingestion rate and thus growth.
The DEB-accumulation model output was used to determine the coefficients in Eq. (19) by the nonlinear regression analysis: b1=0.1909 (±0.0006), b2=0.0412 (±0.0019), b3=0.1315 (±0.0021), and b4=1.1060 (±0.0253). The accurate estimation of the coefficients (b1, b2, b3, b4) is indicated by the low confidence intervals, while the mean squared error of the regression model appears also sufficiently small (MSE =0.0523). Subsequently, as shown in Fig. 13, Eq. (20) may be used to predict the MPs concentration of the environment where mussels live. In most cases, the predicted MPs concentration is found within the standard deviation of the field data. Two exceptions are shown in Hastings-A and Plymouth areas. The reasons behind these discrepancies may be related to the environmental conditions prevailing in each area at the sampling time. For example, Eq. (20) does not take into account the impact of tides that may affected the mussel's MPs load (C), and the lack of information on the exact sampling date led to using a mean SST and CHL a value representative of the given sampling time period (Li et al., 2018). Although Eq. (20) does not account for the tide effect, the sensitivity analysis (Fig. 11) showed that the effect of Cenv on the mussel's MPs accumulation was the same for both the intertidal and subtidal mussel in the North Sea. This result may also apply at the two exceptions areas, leading to the assumption that the discrepancies are due to the lack of the ambient temperature and CHL a information during the sampling date. In any case, this is a first rough demonstration of the method and should be implemented in more environments in order to be further validated.
A DEB-accumulation model was developed and validated with data available from the North Sea and the N Ionian Sea, to study the MPs accumulation by wild M. edulis and cultured M. galloprovincialis, grown in different, representative environments. Although the study is limited by scarce validation data, it should be noted the MPs accumulation model parameter set, except one tuning parameter (kf), was extracted from the literature (Table 3), assuming that mussels adopt a common defensive mechanism against inedible particles (i.e. silt, MPs). Thus, the theoretical background constructed by Saraiva et al. (2011a) (based on Kooijman, 2010) regarding the feeding and excretion processes of the mussel remains unspoiled. Through the strong theoretical background of DEB theory, this study highlights that the accumulation of MPs by the mussel is highly dependent on the prevailing environmental conditions which control the amount of MPs that the mussel filtrates and excretes.
Towards a generic DEB model, the applied function of the half-saturation coefficient, , successfully captures the physiological responses and thus the growth rate of the cultured mussel at the N Ionian Sea implementation. In the current study, this method led to a robust and generic DEB growth model able to simulate the mussel growth in representative mussel habitats of the Mediterranean Sea, covering a range of productivity and sea surface temperature. This approach supports and takes one step further by using the Bourlès et al. (2008) suggestion about a seasonally varied half-saturation coefficient, demonstrating an improvement of the food quantifier. The applied function of Xkconsiders the daily CHL a fluctuations and, thus, the seasonal variation of the seawater composition. As more field data become available from various environments, the applied approach could result to more generic formulations for the site-specific parameter Xk, so that the model could be applied in several areas of interest, where field growth data are absent and/or to simulate the potential mussel growth in the 2D space.
The simulation of MPs accumulation by the mussels, using the DEB-accumulation model, is in good agreement with the available field data (Figs. 3 and 4). The simulated values lie within the observed field data range (mean ± SD), although the seasonal increase reproduced by the model in the North Sea implementation did not exactly overlap with the field data at the time of observations. This could be attributed to the clearance period (24 h) that allowed mussels to excrete MPs through faeces (0.2±0.2 particles per individual) before the mussel's tissue analysis (Van Cauwenberghe et al., 2015). The measured loss of the mussel's MPs is in agreement with the model's result for the depuration experiment after 24 h. The MPs accumulation by the cultivated mussel (fresh tissue mass 3.33 g) originated from the N Ionian Sea with mean particles L−1 is 0.91 particles per individual and by the wild mussel (fresh tissue mass 1.87 g) from the North Sea with mean particles L−1 is 0.53 particles per individual. If these concentrations are expressed per gram of wet tissue of mussel, the cultivated mussel contamination (0.27 particles g−1 w.w.) is comparable with the wild mussel (0.28 particles g−1 w.w.), despite the much lower environmental MPs concentration (Cenv) in the N Ionian Sea than in the North Sea. This comparison aims to highlight the significant impact of the prevailing environmental conditions (CHL a and temperature) on the MPs accumulation by the mussels, although they originate from different areas and lived during different time periods. The generally high abundance of CHL a in the North Sea simulation contributes to a reduction of the filtering activity and hence of the MPs accumulation. The threshold algal concentration for reduction of the mussel's filtration rate (incipient saturation) has been found to lie between 6.3 and 10.0 mg m−3 (Riisgård et al., 2011), which is a range comparable to the CHL a concentrations in the North Sea. Van Cauwenberghe and Janssen (2014) found that cultivated M. edulis from the North Sea contained on average 0.36±0.07 particles g−1 w.w., a slightly higher value than that found in the present study for the wild mussel of the North Sea (0.28 particles g−1 w.w.). This could be attributed to mussel farms acting as a potential source of MPs contamination for the mussels due to plastic materials (i.e. plastic sock nets and polypropylene long lines) used during cultivation (Mathalon and Hill, 2014; Santana et al., 2018). Moreover, the intertidal wild mussel (present study) is assumed to filter and excrete MPs half of the time in comparison with the submerged cultured mussel in the North Sea, resulting though in similar accumulation level. The model also predicts the time needed for the 90 % gut clearance of both cultured (N Ionian Sea) and wild (North Sea) mussels to be almost 284 and 56 h (equivalent to 12 and 2.5 d) respectively, when MPs contamination is removed from their habitat. This is in line with a series of studies which demonstrated that the depuration time varies between 6–72 h and can last up to 40 d depending on several factors such as species, environmental conditions (Bayne et al., 1987), size, and type of MPs (Browne et al., 2008; Ward and Kach, 2009; Woods et al., 2018; Birnstiel et al., 2019).
The strong dependence of food (CHL a), temperature, and seawater MPs concentration on the MPs accumulation by the mussel, regarding its wet weight, is demonstrated through sensitivity experiments that were used to derive a rather simple nonlinear regression model (Eq. 19). The comparison of the regression model's with the DEB model's output resulted in a quite accurate estimation of the coefficients, which in turn sparked the idea of a “new” relationship (Eq. 20) that could potentially predict the MPs concentration in the environment (Cenv) when certain conditions are known (CHL a, T, C, W). The latter equation was applied in eight areas in total (two from the present study areas and six from Li et al., 2018), with relatively good results since there is general overlapping of regressed and observed MPs concentration in the environment (Cenv), except for Hastings-A and Plymouth areas, probably due to missing information on the environmental conditions (CHL a, SST) during the sampling, suggesting that the mussels can be used as potential bioindicators. Mussels have been previously proposed as bioindicators for marine microplastic pollution (<1 mm), although the efficient gut clearance and selective feeding behaviour limit their quantitative ability (Lusher et al., 2017; Bråte et al., 2018; Beyer et al., 2017; Fossi et al., 2018; Li et al., 2019).The recent study by Ward et al. (2019b) demonstrated that bivalves are poor bioindicators of MPs pollution due to the particle selection during feeding and excretion processes that is based on the physical characteristics of the MPs. Considering that the MPs accumulation is site-dependent and that sampling of mussels is usually easier than seawater (Karlsson et al., 2017; Bråte et al., 2018), models like the one described in Eq. (20), besides the MPs accumulation, take into account also characteristics of the environment that are crucial for the way that mussels accumulate MPs. This method could be possibly used at a global level and allow comparisons between various environments. However, the method described should be validated in more environments with more frequent field data to be able to provide secure results.
In addition to the scarce validation data regarding the MPs accumulation in mussels, this study has some more limitations. First of all, the data regarding the concentration of MPs in the mussels' environment are also scarce; since MPs are a relatively recent subject of study, the existing knowledge of the spatial and temporal distribution is still quite limited (Law and Thompson, 2014; Browne, 2015; Anderson et al., 2016; de Sá et al., 2018; Smith et al., 2018; Troost et al., 2018). To overcome the lack of environmental MPs time series, a function of randomly generated values within the observed range of each area was applied, and its uncertainty was examined through an ensemble forecasting. Specifically, the model's uncertainty due to the environmental MPs concentration (Cenv) was tested by performing a series of model runs forced by an envelope of representative values of Cenv. The results (Sect. 3.3) showed that the adopted stochastic scenario simulated quite satisfactorily the MPs accumulation by the mussels, lying within the observed field range, although a slight overestimation was found in the North Sea. The approach used is assumed to represent the natural variability since it has been reported that tides, wind, wave action, ocean currents, river inputs, and hydrodynamic features lead to high spatial and temporal variability in MPs distribution even on very small scales (Messinetti et al., 2018; Goldstein et al., 2013). In addition, the nature of the variable Cenv makes it difficult to estimate, presenting large observational errors, not only due to the intense physical variation but also due to different sampling and analysis techniques that were used. In a future work the DEB-accumulation model could be coupled with a high-resolution MPs distribution model (Kalaroni et al., 2019), being extensively validated against field data that will have been collected and processed according to a common scientifically defined protocol, to overcome this limitation. Moreover, the approach followed in calculating the value of MPs concentration in the near-surface layer (0–5 m depth) (Kooi et al., 2016) resulted in a representative value of the upper-ocean layer. In-depth knowledge of the MPs distribution, both horizontally and vertically, is essential to understand and mitigate their impact not only on the various marine compartments but also on the organisms inhabiting those compartments (Van Sebille et al., 2015; Kooi et al., 2016). For that reason, it is important to enhance the monitoring activity especially in the vulnerable coastal environments, adopting integrated cross-disciplinary approaches and monitoring of biological, physical, and chemical parameters which provide information on the ecosystem function, in order to improve the assessment of emerging pollutants (i.e. MPs) and their impacts on biota (objective of JERICO-RI framework).
Our assumption that the mussel has the same filtration rate for all particles, independent of their chemical composition, size, and shape, is a simplification and an open theme of discussion (see Saraiva et al., 2011a for details). However, in our model application, a pre-ingestive particle selection by the mussel is implied based on the organic–inorganic content of the suspended matter illustrating the different binding probabilities applied for algal and MP particles during the ingestion process. Through an investigation of wild mussel's faeces and pseudofaeces production in laboratory conditions, Zhao et al. (2018) found that the length of MPs was significantly longer in pseudofaeces than in the digestive gland and faeces. Furthermore, Van Cauwenberghe et al. (2015) demonstrated that mussel's faeces contained larger MPs (15–500 µm) compared to the mussel's tissue (20–90 µm). Apparently, smaller-sized MPs seem to be dominant within the mussels in comparison with the size of the MPs in the ambient environment (Li et al., 2018; Qu et al., 2018; Digka et al., 2018b), implying that the mussel is more prone to ingest and retain smaller-sized MPs. As an example, Digka et al. (2018b) confirmed that the smaller MPs (<1 mm) occupy 62.3 %, 96.9 %, and 100 % of the total MPs in seawater, sediments, and mussels from the N Ionian Sea respectively. In a future work this selection pattern regarding size could be simulated by suitable preference weights among different MPs sizes. This will improve the knowledge of the feeding and excretion mechanisms used by the mussels against MPs pollution and the assessment of the ecological footprint (Rist et al., 2019).
Our assumption that the contamination by MPs does not affect the energy budget in terms of growth might also be a simplification as this is a subject currently under investigation. Van Cauwenberghe et al. (2015) found that although mussels M. edulis exposed to MPs increased their energy consumption, the energy reserves were not affected compared to the control organisms, implying that mussels are able to adopt a defensive mechanism against the suspended inorganic particles (i.e. MPs) (Ward and Shumway, 2004). Furthermore, MPs exposure showed no significant effect on a mussel's (Perna perna) energy budget, despite its long duration and relatively realistic intensity, leading to the hypothesis that mussels can acclimate to the MPs exposure to maintain their health (Santana et al., 2018). On the contrary, other authors suggested a significant energy shift from reproduction to structural growth and elevated maintenance costs, probably attributed to the reduced energy intake, when the organisms (i.e. oyster Crassostrea gigas) were contaminated with high and unrealistic concentration of MPs (Sussarellu et al., 2016). Moreover, Gardon et al. (2018) showed that the overall energy balance of oyster Pinctada margaritifera was significantly impacted by the reduced assimilation efficiency in correlation with the exposed dose of MPs, and for that reason energy had to be withdrawn from reproduction to compensate for the energy loss. In the future, dedicated experiments exploring the effects on all components of a DEB model should be carried out considering long-term realistic MPs exposure.
Our use of the tide data led to some model bias, since the model does not take into account the mussel's body temperature change when this is exposed to air. Assessing the mussel's body temperature requires extended experiments in field conditions (Tagliarolo and McQuaid, 2015; Monaco and McQuaid, 2018). The study by Seuront et al. (2019) along the French coast of the eastern English Channel found no significant correlation between air and a mussel's body temperature but demonstrated a significant positive correlation between the body temperature and the hard substrate (i.e. rocks) temperature. However, in the present study the tide effect on processes that are affected by the thermal equation, k(T), is considered indirectly through the metabolic depression (details in Sect. 2.4). Sarà et al. (2011) coupled a DEB model with a biophysical model (Kearney et al., 2010), incorporating the change of mussel's body temperature during emersion by using information of various climatological variables (i.e. solar radiation, air temperature, wind speed, wave height), but ignored the temperature sensitivity for the physiological processes. In a future study, a combined approach of coupling the present DEB-accumulation model with a biophysical model, which includes both the tide effect on the physiological processes and the mussel's body temperature respectively, could be followed and lead to a more detailed simulation of the intertidal mussel.
In a future study the model should be corroborated further by using a larger dataset of MPs accumulation, with sampling of mussels of various sizes and life stages. Currently, the model is mainly limited by the insufficient validation, as a larger dataset could be also used for a better model calibration. However, this study provides a new approach in studying the accumulation of MPs by filter feeders and reveals the relations between characteristics of the mussel's surrounding environment and the MPs accumulation, which is presented with high seasonal fluctuations. Additionally, in a future study the DEB-accumulation model will be coupled to a hydrodynamic–biochemical model (e.g. Petihakis et al., 2002, 2012; Triantafyllou et al., 2003; Tsiaras et al., 2014; Ciavatta et al., 2019; Kalaroni et al., 2020) and a MPs distribution model (Kalaroni et al., 2019) that will provide fields of temperature, food availability, and MPs concentration respectively at the Mediterranean scale and eventually lead to an integrated representation of the MPs accumulation by mussels (Daewel et al., 2008). This fully coupled model will be downscaled to the Cretan Sea SuperSite (a marine observatory dedicated to multiple in situ observations at appropriate spatio-temporal resolution, in a restricted geographical region, maintained over long timescales, and designed to address interdisciplinary objectives, driven by science and society needs), while the parameterization of important biological processes will be redesigned based on the new data which will be acquired in the framework of the JERICO S3 project (http://www.jerico-ri.eu, last access: 28 July 2020). The present study highlights the urgent need for adopting a multidisciplinary monitoring activity by measuring physical, biological, and chemical parameters that are crucial for mapping the MPs distribution, assessing the contamination level of the marine organisms, and investigating the impact on the health status. Overall, despite the limitations mentioned, taking into account that plastics are one of the global hot issues, this particular study could help design next efforts, since it provides indications of the future related priority issues.
The CMEMS Globcolour chlorophyll products are available on the CMEMS web portal (http://marine.copernicus.eu/, last access: 28 July 2020). The dataset used is available as daily-filled (spatial and temporal interpolation) product with a 1 km spatial resolution for the European North West Shelf Seas: OCEANCOLOUR_ATL_CHL_L4_REP_OBSERVATIONS_009_098 (Atlantic and daily-filled). The Globcolour chlorophyll product is available as a daily product with 1 km resolution for Europe, and access is free after registration at http://www.globcolour.info/ (last access: 28 July 2020). GlobColour data (http://globcolour.info) used in this study have been developed, validated, and distributed by ACRI-ST, France. The CMEMS SST products used include daily gap-free maps of sea surface temperature, referred to as L4 product, at 0.04∘ spatial resolution for the European North West Shelf Seas and for the Mediterranean Sea: SST_ATL_SST_L4_REP_OBSERVATIONS_010_026 and SST_MED_SST_L4_REP_OBSERVATIONS_010_021, respectively. Access to all products from CMEMS is granted after free registration at http://marine.copernicus.eu/ (last access: 28 July 2020). The research data regarding the MPs accumulation in the mussels and the ambient environment are publicly accessible and obtained from published research for both study areas (already cited in the paper).
GT conceived the basic idea of the present study and was responsible for the management and coordination of the research planning and execution. NS and YH developed the model code with a contribution from KT. NS collected the existing information on the subject and performed the simulations of the present study with the help of YH when needed. GT, GP, KT, YH, and NS contributed to the interpretation of the results. CT provided the field data of the mussel's microplastic accumulation in the northern Ionian Sea. NS prepared the paper, with critical review, commentary, and revision contributed from all coauthors.
The authors declare that they have no conflict of interest.
This article is part of the special issue “Coastal marine infrastructure in support of monitoring, science, and policy strategies”. It is not associated with a conference.
This study has been conducted using E.U. Copernicus Marine Service Information (http://marine.copernicus.eu/, last access: 28 July 2020).
This work was partially funded by the project Blue Growth with Innovation and application in the Greek Seas – GLAFKI (MIS 5002438) funded by national and EU funds under National Strategic Reference Framework 2014–2020 and the EC H2020 CLAIM project (grant agreement no. 774586). Part of this research has been supported by the JERICO-NEXT project (grant agreement no. 654410).
This paper was edited by Ingrid Puillat and reviewed by two anonymous referees.
Alunno-Bruscia, M., Bourlès, Y., Maurer, D., Robert, S., Mazurié, J., Gangnery, A., Goulletquer, P., and Pouvreau, S.: A single bio-energetics growth and reproduction model for the oyster Crassostrea gigas in six Atlantic ecosystems, J. Sea Res., 66, 340–348, https://doi.org/10.1016/j.seares.2011.07.008, 2011.
Anderson, J. C., Park, B. J., and Palace, V. P.: Microplastics in aquatic environments: Implications for Canadian ecosystems, Environ. Pollut., 218, 269–280, https://doi.org/10.1016/j.envpol.2016.06.074, 2016.
Andrady, A. L.: Microplastics in the marine environment, Mar. Pollut. Bull., 62, 1596–1605, https://doi.org/10.1016/j.marpolbul.2011.05.030, 2011.
ACRI-ST: GlobColour data, available at: http://www.globcolour.info/, last access: 28 July 2020.
Arthur, C., Baker, J., and Bamford, H. (Eds): Proceedings of the International Research Workshop on the Occurrence, Effects and Fate of Microplastic Marine Debris, University of Washington Tacoma, Tacoma, WA, USA, 9–11 September 2008, NOAA Technical Memorandum NOS-OR and R-30, 2009.
Bacher, C. and Gangnery, A.: Use of dynamic energy budget and individual based models to simulate the dynamics of cultivated oyster populations, J. Sea Res., 56, 140–155, https://doi.org/10.1016/j.seares.2006.03.004, 2006.
Bayne, B. and Worrall, C.: Growth and Production of Mussels Mytilus edulis from Two Populations, Mar. Ecol. Prog. Ser., 3, 317–328, https://doi.org/10.3354/meps003317, 1980.
Bayne, B. L., Hawkins, A. J. S., and Navarro, E.: Feeding and digestion by the mussel Mytilus edulis L. (Bivalvia: Mollusca) in mixtures of silt and algal cells at low concentrations, J. Exp. Mar. Bio. Ecol., 111, 1–22, https://doi.org/10.1016/0022-0981(87)90017-7, 1987.
Béjaoui-Omri, A., Béjaoui, B., Harzallah, A., Aloui-Béjaoui, N., El Bour, M., and Aleya, L.: Dynamic energy budget model: a monitoring tool for growth and reproduction performance of Mytilus galloprovincialis in Bizerte Lagoon (Southwestern Mediterranean Sea), Environ. Sci. Pollut. Res., 21, 13081–13094, https://doi.org/10.1007/s11356-014-3265-1, 2014.
Beyer, J., Green, N. W., Brooks, S., Allan, I. J., Ruus, A., Gomes, T., Bråte, I. L. N., and Schøyen, M.: Blue mussels (Mytilus edulis spp.) as sentinel organisms in coastal pollution monitoring: A review, Mar. Environ. Res., 130, 338–365, https://doi.org/10.1016/j.marenvres.2017.07.024, 2017.
Birnstiel, S., Soares-Gomes, A., and da Gama, B. A. P.: Depuration reduces microplastic content in wild and farmed mussels, Mar. Pollut. Bull., 140, 241–247, https://doi.org/10.1016/j.marpolbul.2019.01.044, 2019.
Bourlès, Y., Alunno-Bruscia, M., Pouvreau, S., Tollu, G., Leguay, D., Arnaud, C., Goulletquer, P., and Kooijman, S. A. L. M.: Modelling growth and reproduction of the Pacific oyster Crassostrea gigas: Advances in the oyster-DEB model through application to a coastal pond, J. Sea Res., 62, 62–71, https://doi.org/10.1016/j.seares.2009.03.002, 2009.
Bråte, I. L. N., Hurley, R., Iversen, K., Beyer, J., Thomas, K. V., Steindal, C. C., Green, N. W., Olsen, M., and Lusher, A.: Mytilus spp. as sentinels for monitoring microplastic pollution in Norwegian coastal waters: A qualitative and quantitative study, Environ. Pollut., 243, 383–393, https://doi.org/10.1016/j.envpol.2018.08.077, 2018.
Brewin, R., Ciavatta, S., Sathyendranath, S., Jackson, T., Tilstone, G., and Curran, K.: Uncertainty in Ocean-Color Estimates of Chlorophyll for Phytoplankton Groups, Front. Mar. Sci., 4, 104, https://doi.org/10.3389/fmars.2017.00104, 2017.
Browne, M. A., Galloway, T., and Thompson, R.: Microplastic – an emerging contaminant of potential concern?, Integr. Environ. Assess. Manag., 3, 559–561, https://doi.org/10.1002/ieam.5630030412, 2007.
Browne, M. A., Dissanayake, A., Galloway, T. S., Lowe, D. M., and Thompson, R. C.: Ingested microscopic plastic translocates to the circulatory system of the mussel, Mytilus edulis (L.), Environ. Sci. Technol., 42, 5026–5031, https://doi.org/10.1021/es800249a, 2008.
Browne, M. A.: Sources and Pathways of Microplastics to Habitats, in: Marine Anthropogenic Litter, edited by: Bergmann M., Gutow L., and Klages M., Springer, Cham., 229–244, https://doi.org/10.1007/978-3-319-16510-3_9, 2015.
Capolupo, M., Franzellitti, S., Valbonesi, P., Lanzas, C. S., and Fabbri, E.: Uptake and transcriptional effects of polystyrene microplastics in larval stages of the Mediterranean mussel Mytilus galloprovincialis, Environ. Pollut., 241, 1038–1047, https://doi.org/10.1016/j.envpol.2018.06.035, 2018.
Cardoso, J. F. M. F., Dekker, R., Witte, J. I. J., and ven der Veer, H. W.: Is reproductive failure responsible for reduced recruitment of intertidal Mytilus edulis L. in the western Dutch Wadden Sea?, Senck. Marit., 37, 83–92, https://doi.org/10.1007/BF03043695, 2007.
Casas, S. and Bacher, C.: Modelling trace metal (Hg and Pb) bioaccumulation in the Mediterranean mussel, Mytilus galloprovincialis, applied to environmental monitoring, J. Sea Res., 56, 168–181, https://doi.org/10.1016/j.seares.2006.03.006, 2006.
Ciavatta, S., Kay, S., Brewin, R. J. W., Cox, R., Di Cicco, A., Nencioli, F., Polimene, L., Sammartino, M., Santoleri, R., Skákala, J., and Tsapakis, M.: Ecoregions in the Mediterranean Sea Through the Reanalysis of Phytoplankton Functional Types and Carbon Fluxes, J. Geophys. Res.-Oceans, 124, 6737–6759, https://doi.org/10.1029/2019JC015128, 2019.
Cole, M., Lindeque, P., Halsband, C., and Galloway, T. S.: Microplastics as contaminants in the marine environment: A review, Mar. Pollut. Bull., 62, 2588–2597, https://doi.org/10.1016/j.marpolbul.2011.09.025, 2011.
Cole, M., Lindeque, P., Fileman, E., Halsband, C., Goodhead, R., Moger, J., and Galloway, T. S.: Microplastic ingestion by zooplankton, Environ. Sci. Technol., 47, 6646–6655, https://doi.org/10.1021/es400663f, 2013.
Cucci, T. L., Shumway, S. E., Brown, W. S., and Newell, C. R.: Using phytoplankton and flow cytometry to analyze grazing by marine organisms, Cytometry, 10, 659–669, https://doi.org/10.1002/cyto.990100523, 1989.
Daewel, U., Peck, M. A., Kühn, W., St. John, M. A., Alekseeva, I., and Schrum, C.: Coupling ecosystem and individual-based models to simulate the influence of environmental variability on potential growth and survival of larval sprat (Sprattus sprattus L.) in the North Sea, Fish. Oceanogr., 17, 333–351, https://doi.org/10.1111/j.1365-2419.2008.00482.x, 2008.
de Sá, L. C., Oliveira, M., Ribeiro, F., Rocha, T. L., and Futter, M. N.: Studies of the effects of microplastics on aquatic organisms: What do we know and where should we focus our efforts in the future?, Sci. Total Environ., 645, 1029–1039, https://doi.org/10.1016/j.scitotenv.2018.07.207, 2018.
De Witte, B., Devriese, L., Bekaert, K., Hoffman, S., Vandermeersch, G., Cooreman, K., and Robbens, J.: Quality assessment of the blue mussel (Mytilus edulis): Comparison between commercial and wild types, Mar. Pollut. Bull., 85, 146–155, https://doi.org/10.1016/j.marpolbul.2014.06.006, 2014.
Di Cicco, A., Sammartino, M., Marullo, S., and Santoleri, R.: Regional Empirical Algorithms for an Improved Identification of Phytoplankton Functional Types and Size Classes in the Mediterranean Sea Using Satellite Data, Front. Mar. Sci., 4, 126, https://doi.org/10.3389/fmars.2017.00126, 2017.
Digka, N., Tsangaris, C., Torre, M., Anastasopoulou, A., and Zeri, C.: Microplastics in mussels and fish from the Northern Ionian Sea, Mar. Pollut. Bull., 135, 30–40, https://doi.org/10.1016/j.marpolbul.2018.06.063, 2018a.
Digka, N., Tsangaris, C., Kaberi, H., Adamopoulou, A., and Zeri, C.: Microplastic Abundance and Polymer Types in a Mediterranean Environment, in: Proceedings of the International Conference on Microplastic Pollution in the Mediterranean Sea, Springer Water, edited by: Cocca, M., Di Pace, E., Errico, M., Gentile, G., Montarsolo, A., Mossotti, R., Springer, Cham, 17–24, https://doi.org/10.1007/978-3-319-71279-6_3, 2018b.
El Hourany, R., Abboud-Abi Saab, M., Faour, G., Mejia, C., Crépon, M., and Thiria, S.: Phytoplankton Diversity in the Mediterranean Sea From Satellite Data Using Self-Organizing Maps, J. Geophys. Res.-Oceans, 124, 5827–5843, https://doi.org/10.1029/2019jc015131, 2019.
Enders, K., Lenz, R., Stedmon, C. A., and Nielsen, T. G.: Abundance, size and polymer composition of marine microplastics ≥10 µm in the Atlantic Ocean and their modelled vertical distribution, Mar. Pollut. Bull., 100, 70–81, https://doi.org/10.1016/j.marpolbul.2015.09.027, 2015.
Eriksen, M., Lebreton, L. C. M., Carson, H. S., Thiel, M., Moore, C. J., Borerro, J. C., Galgani, F., Ryan, P. G., and Reisser, J.: Plastic Pollution in the World's Oceans: More than 5 Trillion Plastic Pieces Weighing over 250,000 Tons Afloat at Sea, PloS one, 9, e111913 https://doi.org/10.1371/journal.pone.0111913, 2014.
Everaert, G., Van Cauwenberghe, L., De Rijcke, M., Koelmans, A. A., Mees, J., Vandegehuchte, M., and Janssen, C. R.: Risk assessment of microplastics in the ocean: Modelling approach and first conclusions, Environ. Pollut., 242, 1930–1938, https://doi.org/10.1016/j.envpol.2018.07.069, 2018.
Fossi, M. C., Pedà, C., Compa, M., Tsangaris, C., Alomar, C., Claro, F., Ioakeimidis, C., Galgani, F., Hema, T., Deudero, S., Romeo, T., Battaglia, P., Andaloro, F., Caliani, I., Casini, S., Panti, C., and Baini, M.: Bioindicators for monitoring marine litter ingestion and its impacts on Mediterranean biodiversity, Environ. Pollut., 237, 1023–1040, https://doi.org/10.1016/j.envpol.2017.11.019, 2018.
Gardon, T., Reisser, C., Soyez, C., Quillien, V., and Le Moullac, G.: Microplastics Affect Energy Balance and Gametogenesis in the Pearl Oyster Pinctada margaritifera, Environ. Sci. Technol., 52, 5277–5286, https://doi.org/10.1021/acs.est.8b00168, 2018.
Garnesson, P., Mangin, A., Fanton d'Andon, O., Demaria, J., and Bretagnon, M.: The CMEMS GlobColour chlorophyll a product based on satellite observation: multi-sensor merging and flagging strategies, Ocean Sci., 15, 819–830, https://doi.org/10.5194/os-15-819-2019, 2019.
GESAMP: Sources, fate and effects of microplastics in the marine environment: a global assessment, edited by: Kershaw, P. J., IMO/FAO/UNESCO-IOC/UNIDO/WMO/IAEA/UN/UNEP/UNDP Joint Group of Experts on the Scientific Aspects of Marine Environmental Protection, Rep. Stud. GESAMP No. 90, 96 pp., https://doi.org/10.13140/RG.2.1.3803.7925, 2015.
Gohin, F., Druon, J. N., and Lampert, L.: A five channel chlorophyll concentration algorithm applied to Sea WiFS data processed by SeaDAS in coastal waters, Int. J. Remote Sens., 23, 1639–1661, https://doi.org/10.1080/01431160110071879, 2002.
Goldstein, M. C., Titmus, A. J., and Ford, M.: Scales of spatial heterogeneity of plastic marine debris in the northeast Pacific Ocean, Plos One, 8, 80020, https://doi.org/10.1371/journal.pone.0080020, 2013.
Handå, A., Alver, M., Edvardsen, C. V., Halstensen, S., Olsen, A. J., Øie, G., Reitan, K. I., Olsen, Y., and Reinertsen, H.: Growth of farmed blue mussels (Mytilus edulis L.) in a Norwegian coastal area; comparison of food proxies by DEB modeling, J. Sea Res., 66, 297–307, https://doi.org/10.1016/j.seares.2011.05.005, 2011.
Hantoro, I., Löhr, A. J., Van Belleghem, F. G. A. J., Widianarko, B., and Ragas, A. M. J.: Microplastics in coastal areas and seafood: implications for food safety, Food Addit. Contam. – Part A Chem. Anal. Control. Expo. Risk Assess., 36, 674–711, https://doi.org/10.1080/19440049.2019.1585581, 2019.
Hatzonikolakis, Y., Tsiaras, K., Theodorou, J. A., Petihakis, G., Sofianos, S., and Triantafyllou, G.: Simulation of mussel Mytilus galloprovincialis growth with a dynamic energy budget model in Maliakos and Thermaikos Gulfs (Eastern mediterranean), Aquacult Env. Interac., 9, 371–383, https://doi.org/10.3354/aei00236, 2017.
Hirai, H., Takada, H., Ogata, Y., Yamashita, R., Mizukawa, K., Saha, M., Kwan, C., Moore, C., Gray, H., Laursen, D., Zettler, E. R., Farrington, J. W., Reddy, C. M., Peacock, E. E., and Ward, M. W.: Organic micropollutants in marine plastics debris from the open ocean and remote and urban beaches, Mar. Pollut. Bull., 62, 1683–1692, https://doi.org/10.1016/j.marpolbul.2011.06.004, 2011.
International Ocean-Colour Coordinating Group – IOCCG: Remote sensing of ocean colour in coastal, and other optically-complex waters, Rep. Int. Ocean-Colour Coord. Group 3, edited by S. Sathyendranath, IOCCG, Dartmouth, NS, Canada, 140 pp. https://doi.org/10.25607/OBP-95, 2000.
Jacobs, P., Beauchemin, C., and Riegman, R.: Growth of juvenile blue mussels (Mytilus edulis) on suspended collectors in the Dutch Wadden Sea, J. Sea Res., 85, 365–371, https://doi.org/10.1016/j.seares.2013.07.006, 2014.
Jacobs, P., Troost, K., Riegman, R., and van der Meer, J.: Length- and weight-dependent clearance rates of juvenile mussels (Mytilus edulis) on various planktonic prey items, Helgoland Mar. Res., 69, 101–112, https://doi.org/10.1007/s10152-014-0419-y, 2015.
Jolliff, J. K., Kindle, J. C., Shulman, I., Penta, B., Friedrichs, M. A. M., Helber, R., and Arnone, R. A.: Summary diagrams for coupled hydrodynamic-ecosystem model skill assessment, J. Marine Syst., 76, 64–82, https://doi.org/10.1016/j.jmarsys.2008.05.014, 2009.
Jørgensen, C., Larsen, P., and Riisgård, H.: Effects of temperature on the mussel pump, Mar. Ecol. Prog. Ser., 64, 89–97, https://doi.org/10.3354/meps064089, 1990.
Kach, D. and Ward, J.: The role of marine aggregates in the ingestion of picoplankton-size particles by suspension-feeding molluscs, Mar. Biol., 153, 797–805, https://doi.org/10.1007/s00227-007-0852-4, 2007.
Kalaroni, S., Hatzonikolakis, Y., Tsiaras, K., Gkanasos, A., and Triantafyllou, G.: Modelling the Marine Microplastic Distribution from Municipal Wastewater in Saronikos Gulf (E. Mediterranean), Oceanogr Fish Open Access J., 9, 555752, https://doi.org/10.19080/OFOAJ.2019.09.555752, 2019.
Kalaroni, S., Tsiaras, K., Petihakis, G., Economou-Amilli, A., and Triantafyllou, G.: Modelling the Mediterranean pelagic ecosystem using the POSEIDON ecological model. Part I: Nutrients and chlorophyll a dynamics, Deep-Sea Res. Pt. II, 171, 104647, https://doi.org/10.1016/j.dsr2.2019.104647, 2020.
Karayücel, S., Çelik, M. Y., Karayücel, I., and Erik, G.: Karadeniz'de Sinop İlinde Akdeniz Midyesinin (Mytilus galloprovincialis Lamarck, 1819) Sal Sisteminde Büyümesi ve Üretimi, Turk. J. Fish. Aquat. Sc., 10, 9–17, https://doi.org/10.4194/trjfas.2010.0102, 2010.
Karlsson, T. M., Vethaak, A. D., Almroth, B. C., Ariese, F., van Velzen, M., Hassellöv, M., and Leslie, H. A.: Screening for microplastics in sediment, water, marine invertebrates and fish: Method development and microplastic accumulation, Mar. Pollut. Bull., 122, 403–408, https://doi.org/10.1016/j.marpolbul.2017.06.081, 2017.
Kearney, M., Simpson, S. J., Raubenheimer, D., and Helmuth, B.: Modelling the ecological niche from functional traits, Philos. T. Roy. Soc. B, 365(1557), 3469–3483, https://doi.org/10.1098/rstb.2010.0034, 2010.
Khan, M. B. and Prezant, R. S.: Microplastic abundances in a mussel bed and ingestion by the ribbed marsh mussel Geukensia demissa, Mar. Pollut. Bull., 130, 67–75, https://doi.org/10.1016/j.marpolbul.2018.03.012, 2018.
Kiørboe, T. and Møhlenberg, F.: Particle Selection in Suspension-Feeding Bivalves, Mar. Ecol. Prog. Ser., 5, 291–296, https://doi.org/10.3354/meps005291, 1981.
Kooi, M., Reisser, J., Slat, B., Ferrari, F. F., Schmid, M. S., Cunsolo, S., Brambini, R., Noble, K., Sirks, L. A., Linders, T. E. W., Schoeneich-Argent, R. I., and Koelmans, A. A.: The effect of particle properties on the depth profile of buoyant plastics in the ocean, Sci. Rep., 6, 33882 https://doi.org/10.1038/srep33882, 2016.
Kooijman, S. A. L. M.: Dynamic Energy and Mass Budgets in Biological Systems. Cambridge University Press, Cambridge, 2000.
Kooijman, S. A. L. M.: Pseudo-faeces production in bivalves, J. Sea Res., 56, 103–106, https://doi.org/10.1016/j.seares.2006.03.003, 2006.
Kooijman S. A. L. M.: Dynamic Energy Budget Theory for Metabolic Organisation, Cambridge University Press, Cambridge, 2010.
Lacroix, G., Ruddick, K., Ozer, J., and Lancelot, C.: Modelling the impact of the Scheldt and Rhine/Meuse plumes on the salinity distribution in Belgian waters (southern North Sea), J. Sea Res., 52, 149–163, https://doi.org/10.1016/j.seares.2004.01.003, 2004.
Lattin, G. L., Moore, C. J., Zellers, A. F., Moore, S. L., and Weisberg, S. B.: A comparison of neustonic plastic and zooplankton at different depths near the southern California shore, Mar. Pollut. Bull., 49, 291–294, https://doi.org/10.1016/j.marpolbul.2004.01.020, 2004.
Law, K. L. and Thompson, R. C.: Microplastics in the seas, Science, 345, 144–145, https://doi.org/10.1126/science.1254065, 2014.
Lenz, R., Enders, K., and Nielsen, T. G.: Microplastic exposure studies should be environmentally realistic, P. Natl. Acad. Sci. USA, 113, E4121–E4122, https://doi.org/10.1073/pnas.1606615113, 2016.
Li, J., Qu, X., Su, L., Zhang, W., Yang, D., Kolandhasamy, P., Li, D., and Shi, H.: Microplastics in mussels along the coastal waters of China, Environ. Pollut., 214, 177–184, https://doi.org/10.1016/j.envpol.2016.04.012, 2016.
Li, J., Green, C., Reynolds, A., Shi, H., and Rotchell, J. M.: Microplastics in mussels sampled from coastal waters and supermarkets in the United Kingdom, Environ. Pollut., 241, 35–44, https://doi.org/10.1016/j.envpol.2018.05.038, 2018.
Li, J., Lusher, A. L., Rotchell, J. M., Deudero, S., Turra, A., Bråte, I. L. N., Sun, C., Shahadat Hossain, M., Li, Q., Kolandhasamy, P., and Shi, H.: Using mussel as a global bioindicator of coastal microplastic pollution, Environ. Pollut., 244, 522–533, https://doi.org/10.1016/j.envpol.2018.10.032, 2019.
Liubartseva, S., Coppini, G., Lecci, R., and Clementi, E.: Tracking plastics in the Mediterranean: 2D Lagrangian model, Mar. Pollut. Bull., 129, 151–162, https://doi.org/10.1016/j.marpolbul.2018.02.019, 2018.
Lusher, A.: Microplastics in the marine environment: Distribution, interactions and effects, Mar. Anthropog. Litter, 245–307, https://doi.org/10.1007/978-3-319-16510-3_10, 2015.
Lusher, A., Bråte, I. L. N., Hurley, R., Iversen, K., and Olsen, M.: Testing of methodology for measuring microplastics in blue mussels (Mytilus spp) ans sediments, and recommendations for future monitoring of microplastics, Norwegian Institute for Water Research, 87, 7209, https://doi.org/10.13140/RG.2.2.24399.59041, 2017.
Maes, T., Van der Meulen, M. D., Devriese, L. I., Leslie, H. A., Huvet, A., Frère, L., Robbens, J., and Vethaak, A. D.: Microplastics baseline surveys at the water surface and in sediments of the North-East Atlantic, Front. Mar. Sci., 4, 135, https://doi.org/10.3389/fmars.2017.00135, 2017.
Maire, O., Amouroux, J. M., Duchêne, J. C., and Grémare, A.: Relationship between filtration activity and food availability in the Mediterranean mussel Mytilus galloprovincialis, Mar. Biol., 152, 1293–1307, https://doi.org/10.1007/s00227-007-0778-x, 2007.
MarLIN: The Marine Life Information Network – Common mussel (Mytilus edulis), available at: https://www.marlin.ac.uk/species/detail/1421 (last access: 28 July 2020), 2016.
Mathalon, A. and Hill, P.: Microplastic fibers in the intertidal ecosystem surrounding Halifax Harbor, Nova Scotia, Mar. Pollut. Bull., 81, 69–79, https://doi.org/10.1016/j.marpolbul.2014.02.018, 2014.
Mato, Y., Isobe, T., Takada, H., Kanehiro, H., Ohtake, C., and Kaminuma, T.: Plastic resin pellets as a transport medium for toxic chemicals in the marine environment, Environ. Sci. Technol., 35, 318–324, https://doi.org/10.1021/es0010498, 2001.
Messinetti, S., Mercurio, S., Parolini, M., Sugni, M., and Pennati, R.: Effects of polystyrene microplastics on early stages of two marine invertebrates with different feeding strategies, Environ. Pollut., 237, 1080–1087, https://doi.org/10.1016/j.envpol.2017.11.030, 2018.
Møhlenberg, F., and Riisgård, H. : Efficiency of particle retention in 13 species of suspension feeding bivalves, Ophelia, 17, 239–246, https://doi.org/10.1080/00785326.1978.10425487, 1978.
Monaco, C. J. and McQuaid, C. D.: Applicability of Dynamic Energy Budget (DEB) models across steep environmental gradients, Sci. Rep., 8, 16384, https://doi.org/10.1038/s41598-018-34786-w, 2018.
Moore, C. J., Moore, S. L., Leecaster, M. K., and Weisberg, S. B.: A comparison of plastic and plankton in the North Pacific Central Gyre, Mar. Pollut. Bull., 42, 1297–1300, https://doi.org/10.1016/S0025-326X(01)00114-X, 2001.
Otto, L., Zimmerman, J. T. F., Furnes, G. K., Mork, M., Saetre, R., and Becker, G.: Review of the physical oceanography of the North Sea, Neth. J. Sea Res., 26, 161, https://doi.org/10.1016/0077-7579(90)90091-T, 1990.
Painting, S. J., Collingridge, K. A., Durand, D., Grémare, A., Créach, V., Arvanitidis, C., and Bernard, G.: Marine monitoring in Europe: is it adequate to address environmental threats and pressures?, Ocean Sci., 16, 235–252, https://doi.org/10.5194/os-16-235-2020, 2020.
Palacz, A. P., John, M. A. St., Brewin, R. J. W., Hirata, T., and Gregg, W. W.: Distribution of phytoplankton functional types in high-nitrate, low-chlorophyll waters in a new diagnostic ecological indicator model, Biogeosciences, 10, 7553–7574, https://doi.org/10.5194/bg-10-7553-2013, 2013.
Pascoe, P. L., Parry, H. E., and Hawkins, A. J. S.: Observations on the measurement and interpretation of clearance rate variations in suspension-feeding bivalve shellfish, Aquat. Biol., 6, 181–190, https://doi.org/10.3354/ab00123, 2009.
Pasquini, G., Ronchi, F., Strafella, P., Scarcella, G., and Fortibuoni, T.: Seabed litter composition, distribution and sources in the Northern and Central Adriatic Sea (Mediterranean), Waste Manag., 58, 41–51, https://doi.org/10.1016/j.wasman.2016.08.038, 2016.
Petihakis, G., Triantafyllou, G., Allen, I. J., Hoteit, I., and Dounas, C.: Modelling the spatial and temporal variability of the Cretan Sea ecosystem, J. Marine Syst., 36, 173–196, https://doi.org/10.1016/S0924-7963(02)00186-0, 2002.
Petihakis, G., Triantafyllou, G., Korres, G., Tsiaras, K., and Theodorou, A.: Ecosystem modelling: Towards the development of a management tool for a marine coastal system part-II, ecosystem processes and biogeochemical fluxes, J. Marine Syst., 94, 49–64, https://doi.org/10.1016/j.jmarsys.2011.11.006, 2012.
Politikos, D. V., Tsiaras, K., Papatheodorou, G., and Anastasopoulou, A.: Modeling of floating marine litter originated from the Eastern Ionian Sea: Transport, residence time and connectivity, Mar. Pollut. Bull., 150, 110727, https://doi.org/10.1016/j.marpolbul.2019.110727, 2020.
Pouvreau, S., Bourles, Y., Lefebvre, S., Gangnery, A., and Alunno-Bruscia, M.: Application of a dynamic energy budget model to the Pacific oyster, Crassostrea gigas, reared under various environmental conditions, J. Sea Res., 56, 156–167, https://doi.org/10.1016/j.seares.2006.03.007, 2006.
Prins, T. C., Smaal, A. C., and Pouwer, A. J.: Selective ingestion of phytoplankton by the bivalves Mytilus edulis L. and Cerastoderma edule (L.), Hydrobiol. Bull., 25, 93–100, https://doi.org/10.1007/BF02259595, 1991.
Qu, X., Su, L., Li, H., Liang, M., and Shi, H.: Assessing the relationship between the abundance and properties of microplastics in water and in mussels, Sci. Total Environ., 621, 679–686, https://doi.org/10.1016/j.scitotenv.2017.11.284, 2018.
Raitsos, D. E., Reid, P. C., Lavender, S. J., Edwards, M., and Richardson, A. J.: Extending the SeaWiFS chlorophyll data set back 50 years in the northeast Atlantic, Geophys. Res. Lett., 32, 1–4, https://doi.org/10.1029/2005GL022484, 2005.
Raitsos, D. E., Lavender, S. J., Maravelias, C. D., Haralabous, J., Richardson, A. J., and Reid, P. C.: Identifying four phytoplankton functional types from space: An ecological approach, Limnol. Oceanogr., 53, 605–613, https://doi.org/10.4319/lo.2008.53.2.0605, 2008.
Raitsos, D. E., Korres, G., Triantafyllou, G., Petihakis, G., Pantazi, M., Tsiaras, K., and Pollani, A.: Assessing chlorophyll variability in relation to the environmental regime in Pagasitikos Gulf, Greece, J. Marine Syst., 94, 16–22, https://doi.org/10.1016/j.jmarsys.2011.11.003, 2012.
Raitsos, D. E., Pradhan, Y., Lavender, S. J., Hoteit, I., Mcquatters-Gollop, A., Reid, P. C., and Richardson, A. J.: From silk to satellite: Half a century of ocean colour anomalies in the Northeast Atlantic, Glob. Change Biol., 20, 2117–2123, https://doi.org/10.1111/gcb.12457, 2014.
Ren, J. S.: Effect of food quality on energy uptake, J. Sea Res., 62, 72–74, https://doi.org/10.1016/j.seares.2008.11.002, 2009.
Riisgård, H. U., Kittner, C., and Seerup, D. F.: Regulation of opening state and filtration rate in filter-feeding bivalves (Cardium edule, Mytilus edulis, Mya arenaria) in response to low algal concentration, J. Exp. Mar. Bio. Ecol., 284, 105–127, https://doi.org/10.1016/S0022-0981(02)00496-3, 2003.
Riisgård, H. U., Egede, P. P., and Barreiro Saavedra, I.: Feeding Behaviour of the Mussel, Mytilus edulis?: New Observations, with a Minireview of Current Knowledge, J. Mar. Biol., 2011, 1–13, https://doi.org/10.1155/2011/312459, 2011.
Rios, L. M., Moore, C., and Jones, P. R.: Persistent organic pollutants carried by synthetic polymers in the ocean environment, Mar. Pollut. Bull., 54, 1230–1237, https://doi.org/10.1016/j.marpolbul.2007.03.022, 2007.
Rist, S., Steensgaard, I. M., Guven, O., Nielsen, T. G., Jensen, L. H., Møller, L. F., and Hartmann, N. B.: The fate of microplastics during uptake and depuration phases in a blue mussel exposure system, Environ. Toxicol. Chem., 38, 99–105, https://doi.org/10.1002/etc.4285, 2019.
Romeo, T., Pietro, B., Pedà, C., Consoli, P., Andaloro, F., and Fossi, M. C.: First evidence of presence of plastic debris in stomach of large pelagic fish in the Mediterranean Sea, Mar. Pollut. Bull., 95, 358–361, https://doi.org/10.1016/j.marpolbul.2015.04.048, 2015.
Rosland, R., Strand, Alunno-Bruscia, M., Bacher, C., and Strohmeier, T.: Applying Dynamic Energy Budget (DEB) theory to simulate growth and bio-energetics of blue mussels under low seston conditions, J. Sea Res., 62, 49–61, https://doi.org/10.1016/j.seares.2009.02.007, 2009.
Santana, M. F. M., Moreira, F. T., Pereira, C. D. S., Abessa, D. M. S., and Turra, A.: Continuous Exposure to Microplastics Does Not Cause Physiological Effects in the Cultivated Mussel Perna perna, Arch. Environ. Contam. Toxicol., 74, 594–604, https://doi.org/10.1007/s00244-018-0504-3, 2018.
Sarà, G., Kearney, M., and Helmuth, B.: Combining heat-transfer and energy budget models to predict thermal stress in Mediterranean intertidal mussels, Chem. Ecol., 27, 135–145, https://doi.org/10.1080/02757540.2011.552227, 2011.
Sarà, G., Reid, G. K., Rinaldi, A., Palmeri, V., Troell, M., and Kooijman, S. A. L. M.: Growth and reproductive simulation of candidate shellfish species at fish cages in the Southern Mediterranean: Dynamic Energy Budget (DEB) modelling for integrated multi-trophic aquaculture, Aquaculture, 324–325, 259–266, https://doi.org/10.1016/j.aquaculture.2011.10.042, 2012.
Sarà, G., Milanese, M., Prusina, I., Sarà, A., Angel, D. L., Glamuzina, B., Nitzan, T., Freeman, S., Rinaldi, A., Palmeri, V., Montalto, V., Lo Martire, M., Gianguzza, P., Arizza, V., Lo Brutto, S., De Pirro, M., Helmuth, B., Murray, J., De Cantis, S., and Williams, G. A.: The impact of climate change on mediterranean intertidal communities: Losses in coastal ecosystem integrity and services, Reg. Environ. Chang., 14, 5–17, https://doi.org/10.1007/s10113-012-0360-z, 2014.
Saraiva, S., van der Meer, J., Kooijman, S. A. L. M., and Sousa, T.: Modelling feeding processes in bivalves: A mechanistic approach, Ecol. Model., 222, 514–523, https://doi.org/10.1016/j.ecolmodel.2010.09.031, 2011a.
Saraiva, S., van der Meer, J., Kooijman, S. A. L. M., and Sousa, T.: DEB parameters estimation for Mytilus edulis, J. Sea Res., 66, 289–296, https://doi.org/10.1016/j.seares.2011.06.002, 2011b.
Saraiva, S., Van Der Meer, J., Kooijman, S. A. L. M., Witbaard, R., Philippart, C. J. M., Hippler, D., and Parker, R.: Validation of a Dynamic Energy Budget (DEB) model for the blue mussel Mytilus edulis, Mar. Ecol. Prog. Ser., 463, 141–158, https://doi.org/10.3354/meps09801, 2012.
Schwabl, P., Koppel, S., Konigshofer, P., Bucsics, T., Trauner, M., Reiberger, T., and Liebmann, B.: Detection of various microplastics in human stool: A prospective case series, Ann. Intern. Med., 171, 453–457, https://doi.org/10.7326/M19-0618, 2019.
Seuront, L., Nicastro, K. R., Zardi, G. I., and Goberville, E.: Decreased thermal tolerance under recurrent heat stress conditions explains summer mass mortality of the blue mussel Mytilus edulis, Sci. Rep., 9, 17498, https://doi.org/10.1038/s41598-019-53580-w, 2019.
Skoulikidis, N. T., Economou, A. N., Gritzalis, K. C., and Zogaris, S.: Rivers of the Balkans, in: Rivers Europe, edited by: Tockner, K., Uehlinger, U., and Robinson, C., Academic Press, 421–466, https://doi.org/10.1016/B978-0-12-369449-2.00011-4, 2009.
Smith, M., Love, D. C., Rochman, C. M., and Neff, R. A.: Microplastics in Seafood and the Implications for Human Health, Curr. Environ. Heal. Reports, 5(3), 375–386, https://doi.org/10.1007/s40572-018-0206-z, 2018.
Sprung, M.: Reproduction and fecundity of the mussel mytilus edulis at helgoland (North sea), Helgolander Meeresun., 36, 243–255, https://doi.org/10.1007/BF01983629, 1983.
Strohmeier, T., Strand, Ø., Alunno-Bruscia, M., Duinker, A., and Cranford, P.: Variability in particle retention efficiency by the mussel Mytilus edulis, J. Exp. Mar. Biol. Ecol., 412, 96–102, https://doi.org/10.1016/j.jembe.2011.11.006, 2012.
Sukhotin, A. A. and Kulakowski, E. E.: Growth and population dynamics in mussels (Mytilus edulis L.) cultured in the White Sea, Aquaculture, 101, 59–73, https://doi.org/10.1016/0044-8486(92)90232-A, 1992.
Sukhotin, A. A., Strelkov, P. P., Maximovich, N. V., and Hummel, H.: Growth and longevity of Mytilus edulis (L.) from northeast Europe, Mar. Biol. Res., 3, 155–167, https://doi.org/10.1080/17451000701364869, 2007.
Sussarellu, R., Suquet, M., Thomas, Y., Lambert, C., Fabioux, C., Pernet, M. E. J., Goïc, N. Le, Quillien, V., Mingant, C., Epelboin, Y., Corporeau, C., Guyomarch, J., Robbens, J., Paul-Pont, I., Soudant, P., and Huvet, A.: Oyster reproduction is affected by exposure to polystyrene microplastics, P. Natl. Acad. Sci. USA, 113, 2430–2435, https://doi.org/10.1073/pnas.1519019113, 2016.
Tagliarolo, M. and McQuaid, C. D.: Sub-lethal and sub-specific temperature effects are better predictors of mussel distribution than thermal tolerance, Mar. Ecol. Prog. Ser., 535, 145–159, https://doi.org/10.3354/meps11434, 2015.
Teuten, E. L., Rowland, S. J., Galloway, T. S., and Thompson, R. C.: Potential for plastics to transport hydrophobic contaminants, Environ. Sci. Technol., 41, 7759–7764, https://doi.org/10.1021/es071737s, 2007.
Teuten, E. L., Saquing, J. M., Knappe, D. R. U., Barlaz, M. A., Jonsson, S., Björn, A., Rowland, S. J., Thompson, R. C., Galloway, T. S., Yamashita, R., Ochi, D., Watanuki, Y., Moore, C., Viet, P. H., Tana, T. S., Prudente, M., Boonyatumanond, R., Zakaria, M. P., Akkhavong, K., Ogata, Y., Hirai, H., Iwasa, S., Mizukawa, K., Hagino, Y., Imamura, A., Saha, M., and Takada, H.: Transport and release of chemicals from plastics to the environment and to wildlife, Philos. T. Roy. Soc. B, 364, 2027–2045, https://doi.org/10.1098/rstb.2008.0284, 2009.
Theodorou, J. A., Viaene, J., Sorgeloos, P., and Tzovenis, I.: Production and Marketing Trends of the Cultured Mediterranean Mussel Mytilus galloprovincialis Lamarck 1819, in Greece, J. Shellfish Res., 30, 859–874, https://doi.org/10.2983/035.030.0327, 2011.
Thomas, Y., Mazurié, J., Alunno-Bruscia, M., Bacher, C., Bouget, J. F., Gohin, F., Pouvreau, S., and Struski, C.: Modelling spatio-temporal variability of Mytilus edulis (L.) growth by forcing a dynamic energy budget model with satellite-derived environmental data, J. Sea Res., 66, 308–317, https://doi.org/10.1016/j.seares.2011.04.015, 2011.
Thompson, R. C., Olson, Y., Mitchell, R. P., Davis, A., Rowland, S. J., John, A. W. G., McGonigle, D., and Russell, A. E.: Lost at Sea: Where Is All the Plastic?, Science, 30, 838, https://doi.org/10.1126/science.1094559, 2004.
Triantafyllou, G., Petihakis, G., and Allen, I. J.: Assessing the performance of the Cretan Sea ecosystem model with the use of high frequency M3A buoy data set, Ann. Geophys., 21, 365–375, https://doi.org/10.5194/angeo-21-365-2003, 2003.
Troost, T. A., Wijsman, J. W. M., Saraiva, S., and Freitas, V.: Modelling shellfish growth with dynamic energy budget models: An application for cockles and mussels in the Oosterschelde (southwest Netherlands), Philos. T. Roy. Soc. B, 365, 3567–3577, https://doi.org/10.1098/rstb.2010.0074, 2010.
Troost, T. A., Desclaux, T., Leslie, H. A., van Der Meulen, M. D., and Vethaak, A. D.: Do microplastics affect marine ecosystem productivity?, Mar. Pollut. Bull., 135, 17–29, https://doi.org/10.1016/j.marpolbul.2018.05.067, 2018.
Tsiaras, K. P., Petihakis, G., Kourafalou, V. H., and Triantafyllou, G.: Impact of the river nutrient load variability on the North Aegean ecosystem functioning over the last decades, J. Sea Res., 86, 97–109, https://doi.org/10.1016/j.seares.2013.11.007, 2014.
Vahl, O.: Efficiency of particle retention in mytilus edulis L, Ophelia, 10, 17–25, https://doi.org/10.1080/00785326.1972.10430098, 1972.
van Beusekom, J. E. E., Loebl, M., and Martens, P.: Distant riverine nutrient supply and local temperature drive the long-term phytoplankton development in a temperate coastal basin, J. Sea Res., 61, 26–33, https://doi.org/10.1016/j.seares.2008.06.005, 2009.
Van Cauwenberghe, L. and Janssen, C. R.: Microplastics in bivalves cultured for human consumption, Environ. Pollut., 193, 65–70, https://doi.org/10.1016/j.envpol.2014.06.010, 2014.
Van Cauwenberghe, L., Claessens, M., Vandegehuchte, M. B., and Janssen, C. R.: Microplastics are taken up by mussels (Mytilus edulis) and lugworms (Arenicola marina) living in natural habitats, Environ. Pollut., 199, 10–17, https://doi.org/10.1016/j.envpol.2015.01.008, 2015.
van der Veer, H. W., Cardoso, J. F. M. F., and van der Meer, J.: The estimation of DEB parameters for various Northeast Atlantic bivalve species, J. Sea Res., 56, 107–124, https://doi.org/10.1016/j.seares.2006.03.005, 2006.
van Haren, R. J. F., Schepers, H. E., and Kooijman, S. A. L. M.: Dynamic energy budgets affect kinetics of xenobiotics in the marine mussel Mytilus edulis, Chemosphere, 29, 163–189, https://doi.org/10.1016/0045-6535(94)90099-X, 1994.
Van Sebille, E., Wilcox, C., Lebreton, L., Maximenko, N., Hardesty, B. D., Van Franeker, J. A., Eriksen, M., Siegel, D., Galgani, F., and Law, K. L.: A global inventory of small floating plastic debris, Environ. Res. Lett., 10, 124006, https://doi.org/10.1088/1748-9326/10/12/124006, 2015.
Vandermeersch, G., Lourenço, H. M., Alvarez-Muñoz, D., Cunha, S., Diogène, J., Cano-Sancho, G., Sloth, J. J., Kwadijk, C., Barcelo, D., Allegaert, W., Bekaert, K., Fernandes, J. O., Marques, A., and Robbens, J.: Environmental contaminants of emerging concern in seafood – European database on contaminant levels, Environ. Res., 143, 29–45, https://doi.org/10.1016/j.envres.2015.06.011, 2015.
Vlachogianni, T., Anastasopoulou, A., Fortibuoni, T., Ronchi, F., and Zeri, C.: Marine Litter Assessment in the Adriatic and Ionian Seas, IPA-Adriatic DeFishGear Project, MIO-ECSDE, HCMR and ISPRA, 168 pp., ISBN 978-960-6793-25-7, 2017.
Von Moos, N., Burkhardt-Holm, P., and Köhler, A.: Uptake and effects of microplastics on cells and tissue of the blue mussel Mytilus edulis L. after an experimental exposure, Environ. Sci. Technol., 46, 11327–11335, https://doi.org/10.1021/es302332w, 2012.
Ward, J. E. and Kach, D. J.: Marine aggregates facilitate ingestion of nanoparticles by suspension-feeding bivalves, Mar. Environ. Res., 68, 137–142, https://doi.org/10.1016/j.marenvres.2009.05.002, 2009.
Ward, J. E. and Shumway, S. E.: Separating the grain from the chaff: Particle selection in suspension- and deposit-feeding bivalves, J. Exp. Mar. Bio. Ecol., 300(, 83–130, https://doi.org/10.1016/j.jembe.2004.03.002, 2004.
Ward, J. E., Rosa, M., and Shumway, S.: Capture, ingestion, and egestion of microplastics by suspension-feeding bivalves: a 40-year history, Anthr. Coasts, 2, 39–49, https://doi.org/10.1139/anc-2018-0027, 2019a.
Ward, J. E., Zhao, S., Holohan, B. A., Mladinich, K. M., Griffin, T. W., Wozniak, J., and Shumway, S. E.: Selective Ingestion and Egestion of Plastic Particles by the Blue Mussel (Mytilus edulis) and Eastern Oyster (Crassostrea virginica): Implications for Using Bivalves as Bioindicators of Microplastic Pollution, Environ. Sci. Technol., 53, 8776–8784, https://doi.org/10.1021/acs.est.9b02073, 2019b.
Wegner, A., Besseling, E., Foekema, E. M., Kamermans, P., and Koelmans, A. A.: Effects of nanopolystyrene on the feeding behavior of the blue mussel (Mytilus edulis L.), Environ. Toxicol. Chem., 31, 2490–2497, https://doi.org/10.1002/etc.1984, 2012.
Widdows, J., Fieth, P., and Worrall, C. M.: Relationships between seston, available food and feeding activity in the common mussel Mytilus edulis, Mar. Biol., 50, 195–207, https://doi.org/10.1007/BF00394201, 1979.
Wieczorek, A. M., Morrison, L., Croot, P. L., Allcock, A. L., MacLoughlin, E., Savard, O., Brownlow, H., and Doyle, T. K.: Frequency of microplastics in mesopelagic fishes from the Northwest Atlantic, Front. Mar. Sci., 5, https://doi.org/10.3389/fmars.2018.00039, 2018.
Woods, M. N., Stack, M. E., Fields, D. M., Shaw, S. D., and Matrai, P. A.: Microplastic fiber uptake, ingestion, and egestion rates in the blue mussel (Mytilus edulis), Mar. Pollut. Bull., 137, 638–645, https://doi.org/10.1016/j.marpolbul.2018.10.061, 2018.
Zaldivar, J. M.: A general bioaccumulation DEB model for mussels, in: JRC Scientific and Technical Reports, EUR 23626, Office for Official Publications of the European Communities, Luxembourg, ii, 31 pp., ISBN 978-92-79-10943-0, 2008.
Zeri, C., Adamopoulou, A., Bojanić Varezić, D., Fortibuoni, T., Kovač Viršek, M., Kržan, A., Mandic, M., Mazziotti, C., Palatinus, A., Peterlin, M., Prvan, M., Ronchi, F., Siljic, J., Tutman, P., and Vlachogianni, T.: Floating plastics in Adriatic waters (Mediterranean Sea): From the macro- to the micro-scale, Mar. Pollut. Bull., 136, 341–350, https://doi.org/10.1016/j.marpolbul.2018.09.016, 2018.
Zhao, S., Ward, J. E., Danley, M., and Mincer, T. J.: Field-Based Evidence for Microplastic in Marine Aggregates and Mussels: Implications for Trophic Transfer, Environ. Sci. Technol., 52, 11038–11048, https://doi.org/10.1021/acs.est.8b03467, 2018.