the Creative Commons Attribution 4.0 License.
the Creative Commons Attribution 4.0 License.
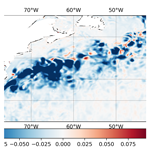
Assessing the impact of future altimeter constellations in the Met Office global ocean forecasting system
Robert R. King
Matthew J. Martin
Lucile Gaultier
Jennifer Waters
Clément Ubelmann
Craig Donlon
Satellite altimeter measurements of sea surface height (SSH) are a crucial component of current operational ocean forecasting systems. The launch of the Surface Water and Ocean Topography (SWOT) wide-swath altimeter (WiSA) mission is bringing a step change in our observing capacity with 2D mesoscale structures now able to be observed over the global ocean. Proposals are now being considered for the make-up of the future altimeter constellation. In this study we use Observing System Simulation Experiments (OSSEs) to compare the impact of additional altimeter observations from two proposed future satellite constellations. We focus on the expected impact on the Met Office operational ocean analysis and forecasting system of assimilating an observation network including either 12 nadir altimeters or 2 wide-swath altimeters.
Here we show that an altimeter constellation of 12 nadir altimeters produces greater reductions in the errors for SSH, surface currents, temperature, and salinity fields compared to a constellation of 2 wide-swath altimeters. The impact is greatest in the dynamic western boundary current (WBC) regions where the nadir altimeters can reduce the SSH RMS (root-mean-square) error by half, while the wide-swath altimeter only reduces this by one-quarter. A comparison of the spatial scales resolved in daily SSH fields also highlights the superiority of the nadir constellation in our forecasting system. We also highlight the detrimental impact spatially correlated errors could have on the immediate use of wide-swath altimeter observations. However, we still achieve promising impacts from the assimilation of wide-swath altimetry, and work is ongoing to develop improved methods to account for spatially correlated observation errors within our data assimilation scheme.
- Article
(10376 KB) - Full-text XML
- BibTeX
- EndNote
The works published in this journal are distributed under the Creative Commons Attribution 4.0 License. This licence does not affect the Crown copyright work, which is re-usable under the Open Government Licence (OGL). The Creative Commons Attribution 4.0 License and the OGL are interoperable and do not conflict with, reduce, or limit each other.
© Crown copyright 2024
Measurements of sea surface height (SSH) from satellite altimeters have been available for more than 30 years and are routinely assimilated into ocean reanalysis and forecasting systems (Le Traon et al., 2017; Davidson et al., 2019). They play a crucial role in constraining the mesoscale ocean dynamics in these systems, allowing estimates of the past, current, and future state of the ocean to be made. Until recently, altimeters only measured along a track directly under the path of the satellites (the nadir track). The nature of these nadir altimeters means that the smallest scales which can be resolved along track are between about 35 and 55 km depending on the altimeter (Pujol et al., 2023). While multiple satellites are available, there are still not enough data to fully constrain the mesoscale dynamics due to the large gaps between tracks on any particular day. Ballarotta et al. (2019) estimated the spatial and temporal scales of maps of sea level anomaly (SLA) created using three nadir altimeters, with the mean effective spatial resolution at mid-latitudes estimated to be about 200 km.
The Surface Water and Ocean Topography (SWOT) satellite mission (Morrow et al., 2019) was launched in December 2022 with some data products already available and near-real-time swath data products expected to be available sometime in 2024. It is the first satellite mission to measure SSH across a 120 km wide swath. There is a 20 km gap in the middle of the swath, in the centre of which a nadir instrument measures the SSH. SWOT is expected to observe the 2D structure of mesoscale ocean processes down to between 15–30 km in wavelength, depending on the sea state, but will have a 21 d repeat time so will only revisit the same ocean features on relatively long timescales. SWOT is an experimental mission to demonstrate the concept of swath altimetry and is expected to provide very useful information which can be assimilated into ocean forecasting systems to improve the accuracy of their analyses and forecasts.
There are plans to include more than one wide-swath altimeter (WiSA) as part of the Sentinel-3 Next Generation (S3-NG) operational mission, which is likely to fly in around 2030. Different combinations of nadir and swath altimeters are being considered by the European Space Agency (ESA) for S3-NG, and the aim of the work described here is to contribute information about the impact of different altimeter constellations on operational ocean forecasts. The two main options which are studied here include a constellation of 12 nadir altimeters and a constellation of 2 wide-swath altimeter satellites.
The impact of potential future observations on data assimilation systems is traditionally assessed by running Observing System Simulation Experiments (OSSEs; Fujii et al., 2019), and that is the approach taken in this study. In an OSSE, a “nature run”, usually a high-resolution model run without data assimilation, is used as a representation of the true ocean. Observations are simulated using the nature run fields for both the existing observing systems and the new data type. This simulation of observations includes a representation of the errors expected from each observation type. The simulated observations from the existing observing systems are assimilated into a model which is usually different to the one used to generate the nature run (with different initial conditions, different surface atmospheric forcing, and sometimes a different resolution), to generate a “control” experiment. Another experiment is run assimilating the simulated observations for the existing observing systems and the new observing system. Both the control run and the experiments assimilating additional observations can be compared to the nature run fields in order to assess the reduction in error expected from assimilating the new data in addition to the existing observing systems. See, for example, Halliwell et al. (2017) for a complete description of the approach.
Recent examples of the application of OSSEs to study the impact of SWOT data include the work of King and Martin (2021) in a regional high-resolution (1.5 km) ocean forecasting system and the work of Benkiran et al. (2021) and Tchonang et al. (2021) in a global °-resolution ocean forecasting system. King and Martin (2021) included experiments showing the impact of including correlated observation errors in the simulated data since real SWOT data are expected to contain spatially correlated errors.
One of the limitations of the OSSE approach is that the results are dependent on the model, the data assimilation scheme, and other aspects of the experimental setup (such as the realism of the simulated observation errors). Additionally, OSSEs using complex, high-resolution ocean forecasting systems are computationally expensive to run, limiting the number of scenarios which can be tested. Also, since operational ocean forecasting systems are continually developed, the impact in today's systems will likely be different to the impact in the systems when data from future missions are actually available. The need for results from multiple systems to address this forecasting system dependence is considered important by the community (see Oke and O'Kane, 2011; Fujii et al., 2019; Martin et al., 2020) in order to provide a more robust assessment of impact for future observing system design. The project to which this work contributes developed a coordinated framework, and OSSEs were run using the Met Office ocean forecasting system (described here) and the Mercator Ocean International ocean forecasting system (described by Benkiran et al., 2024).
The Forecasting Ocean Assimilation Model (FOAM) is the Met Office's ocean forecasting system and consists of global and regional configurations. The global ocean/sea-ice system (Barbosa Aguiar et al., 2024) is run at ° resolution, while a regional system around the UK is run at 1.5 km resolution (Tonani et al., 2019). A lower-resolution (°) version of the global configuration is used as part of the Met Office's coupled numerical weather prediction (NWP) system (Guiavarc'h et al., 2019). This is used to produce ocean reanalyses and as part of the Met Office's coupled seasonal prediction system (GloSea; MacLachlan et al., 2015). These forecasting systems assimilate SSH data from nadir altimeters, sea surface temperature (SST) data from satellites and in situ platforms, in situ profiles of temperature and salinity from various sources including Argo and gliders, and sea-ice concentration (SIC) data from satellites (in the global systems only). These data are assimilated using a 3D-Var version of the NEMOVAR data assimilation software (Waters et al., 2015; Mirouze et al., 2016) together with the NEMO ocean (Madec et al., 2022) and CICE sea-ice models (Hunke and Lipscombe, 2010). In this study we use OSSEs to determine which of two specific future constellations, proposed by ESA, would have the most impact in our global operational forecasting system. This will also allow us to identify issues that will affect the assimilation of real wide-swath altimeter observations and so prepare to make best use of real observations as they become available.
Details of the OSSE framework used in this study are described in Sect. 2, including the nature run, the simulation of observations, the ocean model and data assimilation system used in the assimilation experiments, and details of the experiments. Section 3 presents the results of the OSSEs in terms of the impact of the different altimeter constellations on the sea surface height (SSH), surface currents, temperature, and salinity. It also describes an assessment of the impact on the constrained time and space scales. While the results largely focus on the ° global system, a comparison of the results from the °- and °-resolution versions of the system is also presented. Most of the results are from the assimilation of wide-swath altimeter data without the expected correlated observation errors, but we show some results from experiments which include these correlated errors in the ° system to highlight some of the issues expected from more realistic data. Finally a discussion of the results is given in Sect. 4, and our conclusions are given in Sect. 5.
Observing System Simulation Experiments (OSSEs) require several components: a nature run, observations simulated from the nature run, and additional OSSE runs using a different model setup into which the simulated observations are assimilated (Hoffman and Atlas, 2016; Halliwell et al., 2017). The nature run provides the truth against which other experiments are assessed and from which we sample observations. In this section we describe the nature run used here, the simulation of the observations, the model and data assimilation system used in the OSSEs, and the experimental setup.
2.1 Nature run
To draw useful conclusions about changes to the observing system, the nature run needs to be a realistic representation of the ocean. Additionally, the OSSEs must use an ocean model which differs in enough respects so that there is sufficient error growth between the nature run and OSSEs to emulate the differences between an operational system and the real ocean. Ideally, an OSSE would use a separate lower-resolution ocean model with different parameterisations and different surface boundary inputs and be initialised from a different (though realistic) state.
In this work the nature run was chosen to be a ° global free-running NEMO model (with no data assimilation), the same resolution as the OSSEs, taken from Mercator Ocean and described by Gasparin et al. (2019) and Lellouche et al. (2018). The nature run differs from the OSSE in the version of the NEMO ocean model (version 3.1 for the nature run), encompassing changes to the bottom friction, vertical mixing schemes, and the introduction of a non-linear free surface; the parameter settings used; the sea-ice model (LIM2 for the nature run, CICE for the OSSEs); the surface forcing; and the initial conditions. The nature run was initialised on 11 October 2006 from the EN4 dataset (Good et al., 2013) and was forced at the surface by atmospheric fields from the real-time atmospheric analysis produced at ECMWF (https://www.ecmwf.int/en/forecasts/datasets/set-i, last access: 29 October 2024). Here we consider the period starting in January 2009. The realism of the nature run in terms of large-scale variability was assessed by Gasparin et al. (2018).
2.2 Simulation of observations
2.2.1 Standard observation types
The standard set of observations currently assimilated operationally include in situ and satellite SST data, satellite nadir altimeter along-track SSH data, in situ profiles of temperature and salinity, and satellite sea-ice concentration (SIC) data. Simulated observations were generated for each of these data types using model data from the nature run described above. The positions of the observations were taken from real-world observation coverage representative of a recent period. Realistic observation errors were generated for each type using methods described by Mao et al. (2020) for SST and SIC and by Gasparin et al. (2019) for in situ profiles. Briefly, this involved adding representation errors by randomly selecting the date either 3 d before or after the observation date, then using these time-shifted nature run values in the interpolation process (instead of the correct date). This produces larger errors in regions with higher variability, which is desirable for generating realistic representation errors and is the same method used by Gasparin et al. (2019) for the AtlantOS intercomparison. Uncorrelated instrumental errors were also added to each observation. These were created by randomly sampling from a Gaussian distribution with zero mean and an appropriate standard deviation for each observation type. The standard deviations used for the synthetic SST (0.1–0.5 K) and in situ profiles (0.01–0.05 K, 0.01–0.05 psu) varied with platform type and are detailed fully in Mao et al. (2020) and Table 4 of Gasparin et al. (2019), respectively. For SIC observations, only representation error was added, but this accounts for uncertainties in the marginal ice zone, so the errors are much larger in this area than elsewhere.
2.2.2 Altimeter observations
As mentioned in the introduction, the two main options for S3-NG altimeter constellations considered here are (i) a constellation of 12 nadir altimeters flying in equidistant Sentinel-3A/B-like orbits and (ii) a constellation of 2 wide-swath altimeters (each with its own nadir altimeter). We also include a baseline altimeter constellation consisting of 3 altimeters meant to be representative of the current Sentinel-3 satellites (A and B) and Sentinel-6. The two S3-NG constellations are assimilated in addition to the nadir altimeter data from Sentinel-6. The data assimilated in the different experiments are summarised in Table 1. Other satellite altimeters are also likely to be producing data at the same time as S3-NG and Sentinel-6, but, since we do not know their likely characteristics, we focus on Sentinel-6 in conjunction with either 2 wide-swath or 12 additional nadir altimeters.
Simulated data of SSH were generated for the existing nadir altimeter constellation and for the wide-swath altimeters using the SWOT simulator described by Gaultier et al. (2016). The nature run data described above were fed into the simulator, and it generated observations with realistic errors. The error budget for the nadir altimeters used the error spectrum computed for current altimeter missions (defined in Esteban-Fernandez, 2018) which includes components resulting from instrumental errors, the residual path delay error from the wet-troposphere correction, and the sea-state bias. The error spectrum was computed from level-3 products which already had the typical corrections applied to real altimeter observations (removal of tides, dynamic atmosphere correction, and long-wavelength errors), allowing us to directly simulate level-3-like altimeter data. The resulting (root-mean-square) RMS error (compared to the nature run truth) in our simulated nadir altimeter observations is ∼ 1.4 cm. The nadir altimeter data were generated with a sampling of 6 km, similar to the near-real-time product assimilated in our operational systems. For the constellation of 12 nadir altimeters, data were simulated assuming the same error budget as for the Sentinel-3 satellites and also with a sampling of 6 km.
For the wide-swath altimeters, the error budget was generated using a simulation for each component of the error expected from SWOT, including phase error, roll error, timing error, KaRIn noise, baseline dilation error, and residual path delay error from the wet-troposphere correction (see Gaultier et al., 2016). Each of these components of the error are available separately, so experiments could be run with different error components. In the main OSSEs described below, only the spatially uncorrelated components of the error budget (the KaRIn noise and the residual path delay error) were included in the assimilated data. This is a major simplification, and we discuss the impact of this later in the paper. The simulated wide-swath altimeter data were generated for the 120 km wide swath (with a gap in the middle of 20 km) at 2 km resolution and were averaged to generate “super-observations” at 10 km resolution, similar to the resolution of the model grid. The KaRIn noise was generated using a Gaussian distribution, with the resulting 2×2 km gridded observations having an RMS of 1.7 cm, reducing to 0.3 cm for the 10 km resolution super-observations. The residual path delay error was simulated by generating a random realisation of a wet troposphere following a spectrum derived from the AMSR instrument and then correcting it using the estimation of the wet troposphere on two beams. The RMS of the residual path delay error was 0.4 cm, which was negligibly affected by the observation averaging. The sum of these two components gives a total RMS error (compared to the nature run truth) in the 10 km resolution observations assimilated in our main experiments of ∼ 0.5 cm, compared to 1.4 cm for the nadir altimeter observations. Additionally, representation errors are introduced by the differences between the nature run (used to simulate the observations) and OSSE model fields. Since the wide-swath altimeter data are expected to suffer from larger errors in high wave conditions, observations were removed when the significant wave height (SWH) was greater than 8 m (a wave model was also generated in conjunction with the main nature run which provided additional inputs to the SWOT simulator). For real data it is likely that the accuracy of the data will suffer at lower SWH than this threshold (see Peral et al., 2015). We note that we have chosen to retain the 6 km resolution of the nadir altimeter observations despite generating “super-observations” at 10 km resolution for the wide-swath altimeter observations. This was a deliberate choice to reflect the pragmatic decisions we intend to make with real wide-swath altimeter observations; that is, we are likely to continue to assimilate the nadir observations at the along-track resolution provided but will average the wide-swath data to approximately match the grid scale of our model.
Our control experiment assimilated on average 188 000 altimeter observations per day. With the superobbing applied to the wide-swath altimeter observations, our 2WISA experiment assimilated on average 970 000 altimeter observations per day (including Sentinel-6, the two wide-swath altimeters, and the nadir altimeter component of each wide-swath altimeter). On the other hand, the NADIR experiment with Sentinel-6 and an additional 12 nadir altimeters assimilated on average 831 000 altimeter observations per day. An example of the observational coverage of SSH from the two scenarios studied is shown in Fig. 1. This figure shows that the wide-swath altimeter data increase the coverage of the ocean on each day, and that there are regions within the swath that are measured with high spatial resolution, but that large gaps still exist. While the wide-swath altimeter data measure regions of the ocean with high spatial resolution, there is a trade-off between the two scenarios in terms of spatial and temporal coverage, particularly in the regularity of the coverage in time and space.
2.3 Ocean model and data assimilation scheme
The ocean model used in the OSSEs is NEMO version 3.6 coupled to the sea-ice model CICE version 5.2.1. The configuration of the ocean model is called GO6 and is described in detail by Storkey et al. (2018), while the sea-ice model configuration is called GSI8.1 and is described by Ridley et al. (2018). This ocean/sea-ice model configuration is the version currently used operationally in the global FOAM system. It is available at two different resolutions: one at ° resolution (called ORCA025) and one at ° resolution (called ORCA12). The main results presented in this work are from runs of the ORCA12 system, but some additional experiments have been run using ORCA025 to investigate the impact of correlated errors in the wide-swath altimeter observations.
The data assimilation system used in FOAM is called NEMOVAR (Waters et al., 2015) and is an incremental multivariate 3D-Var with first guess at appropriate time (FGAT) scheme. A key feature of the scheme is that it uses physical balance relationships to transfer information between the different model variables, as described by Weaver et al. (2005). This means that SSH observations are used to estimate corrections to the model's SSH but also affect the subsurface density field and the horizontal velocities. Similarly, observations of the subsurface temperature and salinity are used to adjust the model's density structure and affect the SSH and horizontal velocities. These different observation types are assimilated simultaneously to produce a single analysis for each 1 d assimilation window.
Another aspect of NEMOVAR is that the information at observation locations is spread spatially (horizontally and vertically) to produce corrections to the model fields at surrounding locations. This is done through the so-called background error correlations, and these are modelled efficiently in NEMOVAR using an implicit diffusion operator (Weaver et al., 2016). In the operational FOAM system, the horizontal correlations are specified using a combination of two functions with distinct length scales, as described in Mirouze et al. (2016). The small-scale errors have a length scale which depends on the first baroclinic Rossby radius and varies from 25 to 300 km, while the large-scale errors have a 400 km length scale. Recent work has shown that the altimeter assimilation produces improved results without the longer of these two length scales, and an update to the FOAM system is being prepared which removes the longer length scale for temperature (Mignac et al., 2024). This change is used in the experiments described here.
Satellite observations often suffer from biases, and NEMOVAR includes the facility to correct these observation biases for SST data (as described by While and Martin, 2019) and for SSH (as described by Lea et al., 2008). For SSH data, the bias correction in FOAM consists of two aspects. The first is that the mean dynamic topography (MDT) needed to compare the observations of SSH (relative to the geoid) with the model's SSH contains errors which result in a bias in the observations. In the idealised OSSE setup used here, however, we produce observations of SSH directly without the need for an external MDT. The estimate of this MDT bias term was therefore initialised to zero and will account for differences in the mean SSH in the two versions of ORCA12 (for the nature run and OSSEs) used in the experiments. At the end of the experiments described later, this MDT bias was small in both magnitude and scale, as expected. The second component of the SSH bias correction is designed to account for differences between the modelled and observed SSH due to errors in the representation and removal of high-frequency atmospheric effects, particularly at mid- to high latitudes (poleward of 40°). As the simulated SSH data were produced from a nature run which used a different source of surface forcing compared to the OSSEs, we retained the second bias correction term in these experiments.
The FOAM system uses a 1 d assimilation window, meaning that an analysis is produced daily using observations over a 24 h period. The observation operator in NEMO is used to calculate a model counterpart to every observation at the nearest model time step and is interpolated to the observation location. The innovations (the difference between the observation and the model counterparts) are used by NEMOVAR together with gridded information about the model state for use in estimating the multivariate balance relationships and with information about the background and observation error covariances. Note that we use the same observation and background errors here as in our operational system. The analysis increments generated by NEMOVAR (the corrections to the model state) are then read into another run of NEMO over the same day, during which a fraction of the increments are added in on each time step using incremental analysis updates (IAUs; Bloom et al., 1996).
2.4 Experimental setup
The OSSE period was chosen to be from January through to July 2009. Three main runs were carried out with the FOAM-ORCA12 model and assimilation system described in the previous sub-section. The first is the control experiment which assimilated all the standard observations, including a representative nadir altimeter constellation consisting of Sentinel-3A and 3B and Sentinel-6. The second is an experiment which assimilated data from an additional 12 nadir altimeters, in addition to the Sentinel-6 altimeter and the standard observations assimilated in the control, which we call NADIR. The third is the 2WISA experiment which assimilated the simulated data from two wide-swath altimeters described in Sect. 2.2.2 in addition to the Sentinel-6 altimeter and the standard observations assimilated in the control.
The control, NADIR, and 2WISA experiments were started from the same initial conditions, which were different to those used in the nature run. This came from a previous reanalysis of the FOAM system valid on 1 January 2009. A 3-week spin-up of the system with assimilation of the simulated standard observations was carried out, and the control, NADIR, and 2WISA experiments then started from the same initial conditions on 21 January 2009. These experiments were forced at the surface by atmospheric fields coming from the ERA5 reanalysis produced by ECMWF (Hersbach et al., 2020), which are different to those used in the nature run (which used the real-time ECMWF atmospheric analysis). A summary of the different experiments is given in Table 1.
Table 1Experimental setup. The standard observations include satellite and in situ SST, in situ profiles of temperature and salinity, and sea-ice concentration.

To demonstrate that the OSSE framework used here is representative of our operational system assimilating real observations, we have compared the innovation statistics between our control run and our operational system. While the observational coverage in the two systems is not a perfect match, it was designed to be similar, and this allows a fairer comparison than using innovation statistics from the operational system and the full-field RMSE from the OSSE control experiment.
The control experiment SSH innovations have a globally averaged root-mean-square (RMS) of ∼ 5.8 cm, broadly consistent with an RMS of 6.4 cm in our operational system. Globally averaged innovation RMS for temperature in the control peaks at about 0.75 °C at 100 m depth and decays to around 0.2 °C at 1500 m depth, which is also similar to the operational FOAM system, where the innovation RMS is around 0.7–1.0 °C at 100 m depth and ∼ 0.2 °C at 1500 m depth. The full-field salinity RMSE in the control experiment peaks at the surface at over 1.5 psu, dominated by regions of high salinity variability and which are sparsely sampled by in situ profiles. However, when comparing innovation statistics, globally averaged innovation RMS for salinity in the control peaks at about 0.18 psu at the surface and decays to around 0.025 psu at 1500 m depth, similar to the operational FOAM system where the innovation RMS is around 0.2 psu at the surface and ∼ 0.025 psu at 1500 m depth. The overall innovation errors in the control experiment are consistent with the errors seen in our operational system, so the idealised experimental framework used here can be viewed as representative of the real system.
In this section, we detail the impact of assimilating the simulated observations by comparing each experiment with the truth provided by the full 4D fields from the nature run. This grid-point-by-grid-point comparison allows us to examine the impact of the assimilation over the full domain, unlike in reality, where our knowledge of the true ocean state is limited. We first describe the impact of each experiment on the SSH, the 3D temperature and salinity, and the surface currents. We then describe the impact on the temporal and spatial resolution of SSH features in each experiment and the differences seen when running the experiments with a lower-resolution model. Finally, we also show the impact of the potential large spatially correlated errors in the wide-swath altimetry data.
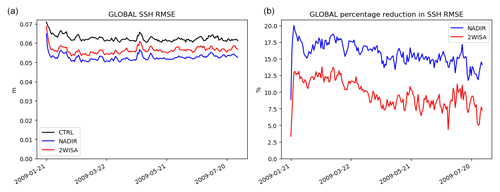
Figure 2Globally averaged SSH RMSE for the ° experiments (a) along with the percentage reduction in the SSH RMSE compared to the control experiment (b).
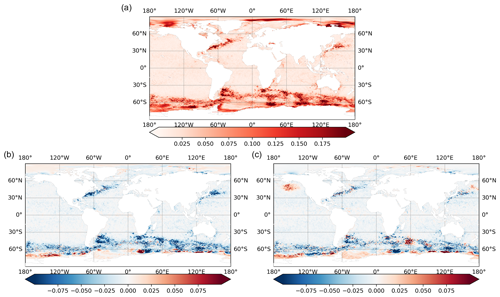
Figure 3Monthly SSH RMSE from July 2009 for the control run (a) and the difference in RMSE compared to the control for the NADIR (b) and 2WISA (c) runs for the ° experiments. Negative values imply a reduction in RMSE for the experiment (NADIR or 2WISA) compared to the control.
3.1 Impact on SSH
The globally averaged impact on SSH of assimilating additional altimeter observations is positive overall in both the NADIR and 2WISA experiments (see Fig. 2). A greater reduction in the SSH RMSE is apparent in the NADIR experiment, where the percentage reduction reaches ∼ 16 % compared to ∼ 10 % in the 2WISA experiment. The western boundary current (WBC) regions dominate the SSH variability and, as shown in Fig. 3, are the regions where the additional altimeter observations have the greatest impact. Broadly, the impact is again greater in the NADIR experiment compared to 2WISA.
Both experiments show regions of degradation under the Antarctic sea-ice. Even though we have no SSH observations in sea-ice-covered areas, the long background error correlation length scale produces changes to the (highly variable) SSH under the sea-ice. While this emulates what happens in our operational system, these experiments have highlighted that we should restrict the spreading of this information under the ice. The 2WISA experiment additionally shows a confined region of degradation in the northeastern Pacific in the SSH RMSE compared to the control. This occurs in a region of very low intrinsic SSH variability (as shown in the map of the monthly RMS of SSH from the nature run in Fig. 4) which is not captured in the background errors in our data assimilation system, resulting in noise being added in this region. Although there are a few other areas with a similarly low SSH variability (north of the Antarctic Circumpolar Current and in the mid-Atlantic), the northeastern Pacific feature aligns with the boundary where we stop applying the full SSH balance (where the temperature stratification is less than 5 K). An inspection of daily SSH increments shows large-length-scale increments between altimeter swaths which align with this boundary in the 2WISA experiment only. It appears that the interaction of very low SSH variability with the transition from applying balanced SSH increments to only barotropic increments leads to spurious SSH changes in this region.
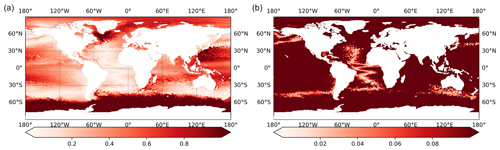
Figure 4RMS of SSH from the nature run over July 2009. The same field is shown in both panels but with a smaller colour range on the right to emphasise the low-variability regions.
Focusing on the Gulf Stream region (Fig. 5), we see that the NADIR experiment shows a large reduction in RMSE (49 % over the region shown) from assimilating observations from the 13 nadir altimeters (Sentinel-6 plus the additional 12 altimeters) compared to the control's 3 nadir altimeters with almost no areas of degradation. In contrast, while the 2WISA experiment reduces the SSH RMSE overall (24 % over the region shown), there are also areas along the main Gulf Stream path and its extension where the SSH RMSE is degraded. This experiment assimilated 1 nadir altimeter in conjunction with the 2 wide-swath altimeters, which results in an uneven spatial sampling on any given day (as shown in Fig. 1).
The daily SSH increments shown in Fig. 6 illustrate the effect of the different spatial sampling in the NADIR and 2WISA experiments. The relatively wide spacing of the altimeter swaths in the 2WISA experiment over our 1 d assimilation window produces short-length-scale increments near the observation locations and longer-length-scale unbalanced SSH increments in the regions between altimeter swaths. In contrast, the relatively close spacing of the altimeter tracks from the 13 nadir altimeters (in the NADIR experiment) over our 1 d assimilation window produces predominantly small-scale SSH increments. The cumulative effect of this is shown by the RMS of the SSH increments over a 21 d period (the repeat cycle of the wide-swath altimeters) in Fig. 6, which indicates that the data assimilation introduces more variability in the 2WISA experiment than in the NADIR experiment. While the wide-swath altimeter data will constrain the SSH in the vicinity of the data on a particular day, there will be a number of days at any given location which are not sampled by the data, during which time the only constraint we have on the SSH comes from the correlations with distant locations. This makes it much harder for the data assimilation to constrain the mesoscale eddy field at all locations with the 2WISA constellation, compared to the constellation of 13 nadir altimeters assimilated in the NADIR experiment, which, while having a less detailed picture of the SSH in particular locations, has a more even sampling on each daily assimilation cycle.
3.2 Impact on subsurface
The assimilation of 13 nadir altimeters (Sentinel-6 plus the additional 12 altimeters) reduces the globally averaged RMSE for temperature profiles to a large extent compared to the control with only 3 nadir altimeters, as shown in Fig. 7. This reduction happens at most depths but is particularly strong between about 100 to 1000 m, with a peak percentage reduction of ∼ 8 % at 400–600 m. In contrast, the reduction in global RMSE for temperature profiles when assimilating the data from 2 wide-swath altimeters plus 1 nadir altimeter is only ∼ 1 %–2 % in the upper 600 m. This is probably due to the regions where we saw degradations in the SSH RMSE (e.g. in the northeastern Pacific and parts of the ACC) also having degradations in the impact on temperature profiles, which offsets any improvements seen elsewhere. In the Gulf Stream region, where there is an overall improvement in SSH RMSE in the 2WISA experiment, there is also an improvement in the temperature profile RMSE at all depths above about 1000 m, peaking at ∼ 8 % at about 600 m depth. The NADIR experiment has an even larger reduction in temperature RMSE in this region, with improvements of over 20 % at the same depth. The balances in our data assimilation scheme allow altimeter observations of the SSH to introduce subsurface changes to the temperature and salinity. However, previous experiments have shown that the assimilation of in situ profiles and altimeter observations can sometimes work against one another (King et al., 2018). With such a large increase in the altimeter observations, the balanced changes applied to subsurface temperature and salinity may dominate over the changes due to the in situ observations leading to degradations in some regions over some depths, such as those seen for temperature below 1000 m in the Gulf Stream region.
The global salinity RMSE is marginally affected by the assimilation of either of the additional altimeter constellations. There is a slight improvement of < 0.25 % in the NADIR experiment relative to the control at most depths but a slight degradation of < 0.25 % in the 2WISA experiment. However, in the Gulf Stream region, the impact of the additional observations is positive at depths of ∼ 300–900 m, with a reduction in salinity RMSE of up to 25 % in the NADIR experiment and ∼ 8 % in the 2WISA experiment.
3.3 Impact on surface currents
The assimilation of altimeter data produces corrections to the geostrophic component of the velocities, and so, given the SSH improvements we saw in Sect. 3.1, we would expect to see improvements to the geostrophic currents but little impact on the ageostrophic component of the velocities. Therefore, here we follow the procedure we have used for the other variables and compare the daily mean surface velocities from the OSSEs to the nature run.
The time series of globally averaged RMSE for the u and v components of the surface current velocity in Fig. 8 shows that the control run velocities take about 4 months to reduce to a stable level, whilst the velocity RMSE in the NADIR and 2WISA experiments reduces to a stable level within about 1 month. The NADIR experiment has a consistently lower RMSE than the 2WISA experiment for both the u- and v-velocity components throughout the experiments. Overall, in the final 2 months of the experiments, the NADIR experiment has 9 % and 11 % reductions in u- and v-velocity RMSE, respectively, while the 2WISA experiment has 4 % and 7 % reductions in u- and v-velocity RMSE, respectively.
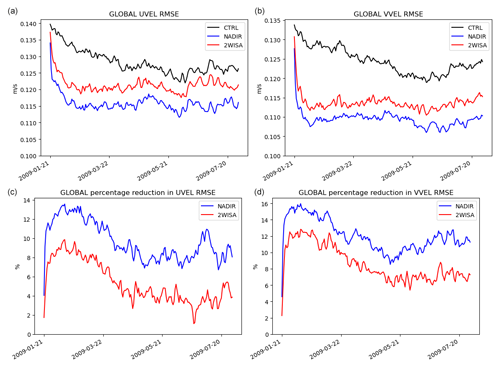
Figure 8Globally averaged time series of the u and v components of surface current velocity RMSE for the control, NADIR, and 2WISA runs (a, b) and the corresponding percentage reduction in RMSE compared to the control (c, d) in the ° system. Note that the y axis in the upper plots does not start from zero to help highlight the differences between the experiments.
Focusing on the last month of the experiments (July 2009), in Fig. 9 we see a similar pattern in the changes in RMSE over the globe for surface currents to the one we saw for SSH, though there are more regions of degradation in the surface currents than for SSH. In the Amazon outflow and Somali Current regions, for instance, there are some degradations in the surface current RMSE. The assimilation scheme used in FOAM does not generate balanced velocity increments near the Equator, so assimilation of extra SSH data in those regions could generate a model response which degrades the velocities. This appears to be the case in both the NADIR and 2WISA experiments, though the regions of degradation are quite small overall.
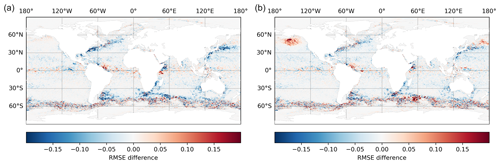
Figure 9Monthly surface current speed RMSE difference from July 2009 compared to the control for the NADIR (a) and 2WISA (b) runs.
Looking in more detail at the Gulf Stream region, the position of the Gulf Stream is noticeably different between the nature run and the control, particularly along the coasts of North and South Carolina (see top panel of Fig. 10). This is improved in the 2WISA run but to a larger extent in the NADIR experiment. The misplacement of the main Gulf Stream path in the control and the differing improvements in the NADIR and 2WISA runs is highlighted by the dipole in the surface current mean error seen in each experiment (see second row of Fig. 10). The magnitude of the dipole reduces in the 2WISA run (compared to that in the control) but is smaller again in the NADIR experiment.
In addition to the change in the position of the main Gulf Stream path, there is the expected misplacement of individual eddies in the control compared to the nature run. For instance, in the nature run, there are two eddies present in the southwestern part of this area to the east of the main Gulf Stream path. However, only one of these eddies is resolved in the control run. Both eddies appear in the 2WISA run but with more diffuse structure than in the nature run, while both eddies are resolved in the NADIR run and are qualitatively similar to the nature run. Similarly, in the northeastern corner of this area, where there is more variability than closer to the coast, it appears that the control run cannot sufficiently initialise the position of mesoscale features. This is somewhat improved in the 2WISA run, but the NADIR run shows a much improved qualitative match to the nature run. It is also clear from the maps of the surface current RMSE and the reduction in the surface current RMSE (see bottom two rows of Fig. 10) that there is an improvement across almost this entire area in the NADIR experiment, while the 2WISA experiment shows regions of slight degradation compared to the control.
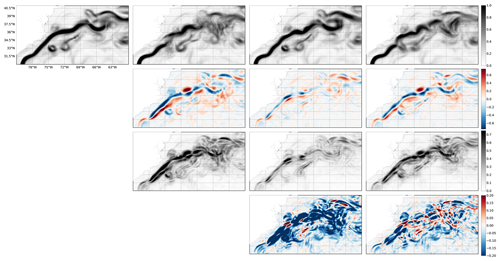
Figure 10Comparison of the monthly (July 2009) mean surface current speed (top row) in the Gulf Stream region near the North American coast for the nature run (top left), control (second column), NADIR (third column), and 2WISA experiments (fourth column). Also shown is the surface current speed mean error (second row) and RMSE (third row) for the control, NADIR, and 2WISA experiments along with the difference in the surface current speed RMSE compared to the control for the NADIR and 2WISA experiments.
3.4 Impact on constrained scales
As mentioned earlier, the wide-swath altimeter satellites will measure the ocean with high resolution within their swath, but the repeat cycle is long (21 d), so their ability to constrain the ocean dynamics on different timescales and space scales is not obvious a priori. To investigate how the assimilation of the different altimeter constellations constrains the model's SSH at different timescales and space scales, we have used two power-spectrum-based metrics which use the ratio of the spectral content of the error (the SSH difference between each experiment and the nature run) and the spectral content of the true signal (from the nature run) to determine a signal-to-noise ratio. Firstly, the frequency–wavenumber 2D power spectrum of the errors in the OSSEs were calculated, with a focus on the Gulf Stream region (defined here as 30–50° N, 40–80° W). This was done using the method of Le Guillou et al. (2021) and the software of Ballarotta et al. (2020) (https://github.com/ocean-data-challenges/2020a_SSH_mapping_NATL60/blob/master/README.md, last access: 29 October 2024) and used the daily SSH fields from the full 6-month duration of the OSSEs.
Figure 11 shows the 2D power spectral density (PSD) score, which compares the power spectrum of the SSH error (i.e. the difference between a specific OSSE and the nature run) with the power spectrum of the nature run SSH for the control, NADIR, and 2WISA experiments. A PSD score of 0.5 corresponds to the space–time scales where the signal is twice the magnitude of the error and is used here to define the boundary between resolved and unresolved space–time scales. All the experiments have large errors at small timescales and short length scales, while the large timescales and space scales are well constrained (have low errors). However, there is a clear improvement in the constrained scales in both NADIR and 2WISA experiments compared to the control. For the larger spatial wavelengths of 4° and above, there is a large improvement in the timescales constrained by assimilating observations from the 12-nadir constellation compared to assimilating observations from the 2 wide-swath altimeter satellites. However, there is an indication that, at spatial wavelengths of 2–4°, the 2WISA experiment constrains the errors at smaller timescales than the NADIR experiment, though the results are rather noisy in this part of the spectrum.
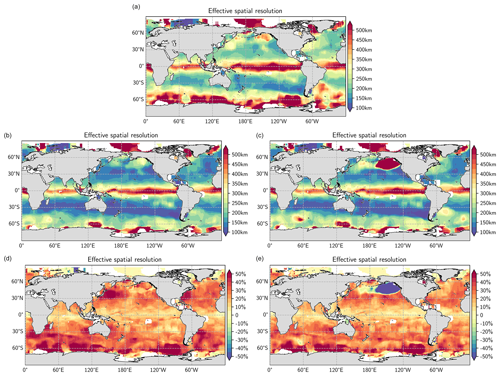
Figure 12Effective spatial resolution of the daily SSH fields from each of the experiments (control run a, NADIR b, 2WISA c) along with the gain in the effective spatial resolution compared to the control for the NADIR (d) and 2WISA (e) runs.
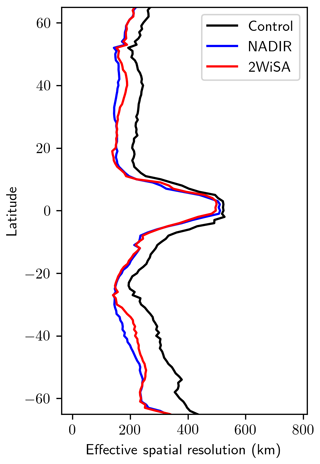
Figure 13Zonal average over the Atlantic basin of the effective spatial resolution of the daily SSH fields from each of the experiments.
To further investigate the ability of the two different altimeter constellations to constrain the ocean dynamics, we used the technique of Ballarotta et al. (2019), which uses the wavenumber power spectrum to calculate an “effective spatial resolution” using the daily mean SSH fields from each day of the experiments. The somewhat arbitrary term of “effective resolution” is useful here to compare similar experiments with the same metrics. However, as discussed by Ballarotta et al. (2019), the scale of resolved ocean features is generally around one-quarter of this “effective resolution”, suggesting that a spatial resolution of 100 km corresponds to resolving features of around 25 km diameter. The method used emulates what can be done with real observations and analysis/forecast fields of SSH. This involves estimating the spatial resolution by calculating the ratio of the power spectral density of the SSH error (the difference between the OSSE and the simulated observation) to the power spectral density of the observations. For the observations, we used the simulated AltiKa observations before any errors were added; i.e. they were samples of the truth from the nature run. The gridded SSH data from each experiment were interpolated to the positions of the AltiKa observations. The along-track and interpolated OSSE data were then split into overlapping 1500 km segments every 300 km. Finally, the globe was segmented into 10°×10° boxes every 1°, and all segments within each box were used to compute the power spectral density. The effective resolution at the centre of each box was then given by the wavelength where the ratio described was equal to 0.5.
The daily effective spatial resolution is shown in Fig. 12 for the control, NADIR, and 2WISA experiments. Also shown is the gain in effective resolution in the NADIR and 2WISA experiments compared to the control. The localised degradation in the northeastern Pacific noted in Sect. 3.1 is again evident here. In fact, the effective resolution highlights the localised nature of this degradation. Despite this, there are still large improvements generally in the 2WISA experiment compared to the control. To assess these without being affected by this region, we computed the zonal average of the effective resolution over the Atlantic basin (shown in Fig. 13). This highlights the difficulty in constraining the ocean dynamics near the Equator in all experiments. The additional observations in the NADIR and 2WISA experiments cannot change this very close to the Equator. However, in both the NADIR and 2WISA experiments, there is a clear improvement in the spatial resolution at mid- to high latitudes, with a gain in resolution of up to around 50 %. As with the earlier results, the NADIR experiment appears superior overall, with a larger gain in the resolution than in the 2WISA experiment across all ocean basins. This superior improvement in the NADIR experiment is particularly evident in all of the western boundary currents.
3.5 Impact of model resolution and correlated errors
To understand the impact in the different operational configurations we run at the Met Office, we repeated the experiments described above (control, NADIR, and 2WISA runs) using our °-resolution ORCA025 system. Similarly to the ORCA12 experiments, these were initialised using a reanalysis of the ORCA025 system valid on 1 January 2009 with a 3-week spin-up where there was assimilation of the standard set of observations. Each experiment was then started from 21 January from the same initial conditions.
The absolute SSH RMSE was higher in each of the ORCA12 experiments compared to the lower-resolution (ORCA025) counterpart, as expected due to the double penalty effect (Rossa et al., 2008). However, the percentage reduction in SSH RMSE shows the NADIR experiment to be superior to the 2WISA experiment in both the high- and low-resolution systems. Although the absolute improvement in RMSE is largest in the low-resolution system, in both systems the percentage reduction is 60 % greater in the NADIR experiment than in the 2WISA experiment. Specifically, while the NADIR and 2WISA experiments reduced the SSH RMSE compared to the control by 16 % and 10 % in the experiments with the ° system detailed above, the reductions were 28 % and 18 %, respectively, in the experiments with the ° system (see Fig. 14).
Unlike for SSH, the velocity RMSE is of similar magnitude in the control run of both the high- and low-resolution systems. Initially, the ORCA025 NADIR experiment is slightly superior to the 2WISA experiment, but, by the final 2 months of the runs, there is a similar impact of 2 % and 7 % reduction in the u and v RMSE, respectively, showing that the higher-resolution system is better able to constrain the surface current RMSE with additional observations and that the NADIR experiment observations allow a greater reduction than the 2WISA observations.
The impacts of assimilating wide-swath observations presented so far have used simulated observations, which included only the uncorrelated KaRIn errors and the residual path delay errors from the wet-troposphere correction but none of the expected correlated errors. To investigate the potential impact of these correlated errors, we have performed three experiments assimilating wide-swath altimeter observations with the (computationally cheaper) °-resolution ORCA025 system. 2WISA assimilates the uncorrelated wide-swath observations, 2WISA_CORR assimilates wide-swath observations which include the full correlated errors, and 2WISA_CORR_TRIM assimilates the observations with correlated errors but discards observations in the outer half of the swath where the correlated errors are largest. No changes were made to our data assimilation scheme for these experiments. For these additional experiments, observations were again simulated using the SWOT simulator tool of Gaultier et al. (2016) and included estimates of the phase, roll, timing, and baseline dilation errors in addition to the KaRIn errors and residual wet-troposphere correction errors described in Sect. 2.2.2 and in King and Martin (2021). The level-2 crossover calibration (as described in Dibarboure et al., 2022) was applied to correct for the simulated roll errors resulting in a small (∼ 2 cm) residual roll error. The total RMS error in the 10 km resolution observations with correlated errors was ∼ 3.9 cm (but with large extrema) compared to 0.5 cm with only the uncorrelated KaRIn errors and the residual path delay errors. Although techniques are being developed to minimise the magnitude of these correlated errors in real data, such methods may be more limited when applied to near-real-time data, which are required for operational ocean prediction. Consequently, these experiments aim to highlight the potential limitations due to correlated errors.
While the assimilation of simulated observations from two wide-swath altimeters without correlated errors (2WISA) reduced the SSH RMSE by 28 %, when the full correlated errors were applied, this reduction was only 5 %. Furthermore, when only observations from the inner half of the swath were used, to discard the largest-magnitude correlated errors, the reduction was still only 6 %. For surface currents, the addition of the correlated errors in the wide-swath altimeter observations results in worse performance and in fact degrades the RMSE compared to the control, even when the observations are restricted to the inner half of the swath. The u and v RMSE was reduced by 2 % and 7 %, respectively, when assimilating observations without correlated errors but increased by 2 % and 1 % when assimilating the restricted observations with correlated errors and increased by 6 % and 8 % for u and v when assimilating the full swath with correlated errors. This highlights the need to develop improved methods to represent correlated observation errors in data assimilation systems such as those of Guillet et al. (2019).
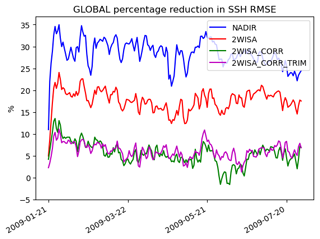
Figure 14Globally averaged percentage reduction in SSH RMSE for the 0.25° experiments compared to the control.
The experiments described here show that a superior impact is found when assimilating the constellation of 12 nadir altimeters. However, similar experiments by the Mercator Ocean International group find the opposite result (Benkiran et al., 2024); that is, in their system, a greater impact is found when assimilating the constellation of 2 wide-swath altimeters. The additional observations assimilated in the two experiments differ in a number of ways which could contribute to the different impacts we have found: the gridding of the observations differs (as explained in Sect. 2.2.2); although both are at slightly higher resolution than the model grid scale, the wide-swath altimeter provides the ability to resolve across-track features, and the spatial and temporal gaps between the tracks/swaths differ markedly. However, an important difference between our system and the Mercator system is the time window used in the data assimilation schemes. The Met Office system uses a 1 d assimilation window (compared to 7 d in the Mercator system), which leads to a less regular spatial sampling of altimeter observations within each analysis window. It appears that a longer assimilation window may be more conducive to initialising mesoscale structures which are relatively static over the window. Whilst such a change could improve results for SSH, this would have to be balanced with the impact on other variables, as in an earlier study we found that a shorter assimilation window improved results for SST with little impact on other variables (Lea et al., 2015). However, given the difference in the daily SSH increments between the NADIR and 2WISA experiments, it may be possible to make better use of the wide-swath altimeter observations with a 1 d assimilation window by altering the balance between the short- and long-length-scale increments.
There was also a specific region of degradation found in the northeastern Pacific affecting the SSH and surface current RMSE fields. This region has very low intrinsic SSH variability which is not accounted for in the background errors in our data assimilation system, resulting in adding noise in this region. This area of degradation also aligns with the boundary where we stop applying the full SSH balance and with large-length-scale SSH increments in the 2WISA experiment. It appears that the interaction of very low SSH variability with the transition from applying balanced SSH increments to only barotropic increments leads to a degradation which is localised to the northeastern Pacific. The comparison of the effective resolution in Fig. 12 further confirms that the effect is localised and affected neither the impact of assimilation in other regions nor the overall order of the impact or our conclusions.
The main experiments reported here were performed without spatially correlated errors included in the simulated wide-swath altimeter observations. However, such correlated errors are expected in real wide-swath data, so additional experiments were run including these error components. The calibration of observations before public release may be able to address major components of these error correlations, but it is not yet clear how effective this will be or whether it will be possible for the near-real-time data products required for assimilation into operational systems. Our experiments showed that, while the assimilation of simulated observations from 2 wide-swath altimeters without correlated errors (2WISA) reduced the SSH RMSE by more then 25 %, when the full correlated errors were applied, this reduction was only 5 %. We further explored the impact of assimilating only the inner portion of the swath where correlated errors are lower, but the effect was minimal, with an SSH RMSE reduction of 6 % compared to the control. The impact of the inclusion of correlated errors was greater for surface currents, where we found the RMSE was reduced when assimilating observations without correlated errors by 2 % and 7 % for the u- and v-velocity components but was increased by 6 % and 8 % when correlated errors were included. In this case, restricting the observations to the inner swath limited the increase in RMSE to 2 % and 1 % for the u and v velocities. We therefore stress the importance of developing improved methods to represent correlated observation errors in data assimilation systems (e.g. Guillet et al., 2019) and testing these with real wide-swath altimeter data. Such data assimilation developments will be a crucial aspect in extracting the full potential of SWOT and other upcoming wide-swath altimeter missions. Additionally, we suggest that the impact of assimilating wide-swath altimetry on the surface currents should be closely monitored, as this is an important physical parameter for many users, which is difficult to verify due to the sparseness of available observations (Aijaz et al., 2023).
With the recent launch of the SWOT wide-swath altimetry mission and the ongoing planning of the future altimeter constellation, we have used an Observing System Simulation Experiment (OSSE) to investigate the potential impact of two proposed next-generation altimeter scenarios in an operational ocean analysis and forecasting system.
In this study, we found that the assimilation of additional altimeter observations has a clear positive impact in both experiments with additional nadir altimeters (NADIR) and additional wide-swath altimeters (2WISA). The SSH RMSE is reduced by 10 % in our 2WISA experiment compared to the control, while the NADIR experiment has a superior reduction in SSH RMSE of 16 %. The greatest impact from the additional observations is seen in dynamic regions such as the western boundary currents; for example, in the Gulf Stream region, the SSH RMSE is reduced by 24 % in the 2WISA experiment and by 49 % in the NADIR experiment. A similar impact is seen on the temperature and salinity fields, where the NADIR experiment is generally superior to 2WISA. While the NADIR experiment shows a reduction in the global temperature RMSE of ∼ 8 % at around 500 m, the reduction is only 1 %–2 % in the 2WISA experiment. The global salinity RMSE was only marginally affected in both the NADIR and 2WISA experiments. However, the impacts were again more pronounced in the western boundary currents, with reductions in the Gulf Stream region of up to 8 % and 20 % for temperature RMSE and up to 8 % and 25 % for salinity RMSE in the 2WISA and NADIR experiments, respectively.
The global surface current fields again show a similar impact, with the NADIR experiment having a consistently lower RMSE for both the u and v components of surface velocity throughout the experiments. By the final 2 months, the NADIR experiment has 9 % and 11 % reductions in the u- and v-velocity RMSE, while the 2WISA experiment has 4 % and 7 % reductions in u- and v-velocity RMSE, respectively. A comparison between the monthly mean surface current fields in the control, NADIR, and 2WISA experiments and those from the nature run showed that the NADIR experiment better corrected the Gulf Stream path and better initialised the positions and strengths of individual eddies both closer to the North American coast and in the Gulf Stream extension region further from the coast. The improvement in the initialisation of mesoscale features is an important aspect expected from the increased observation coverage from either altimeter constellation. Knowledge of the surface currents is important for a number of users of operational ocean forecasts, but there is a significant gap in our ability to observe and constrain this field. Until there are satellite observations of the total surface current vectors, altimetry will remain our most important tool for constraining surface currents in our forecasting systems.
A comparison of the spatial and temporal scales resolved in each of the experiments highlighted a clear improvement in both the NADIR and 2WISA experiments compared to the control. The temporal resolution of features appears superior in the NADIR experiment for length scales greater than 4°, while, at smaller spatial scales, the 2WISA experiment appears to constrain the errors at shorter timescales than the NADIR experiment. Focusing on the spatial resolution of daily SSH fields showed a clear improvement over the control at mid- to high latitudes with a gain in resolution of up to around 50 %, with the NADIR experiment again superior overall with a larger gain in the resolution compared to 2WISA, which was particularly evident in the western boundary current regions.
The European Space Agency (ESA) is planning its future altimeter constellation through the Sentinel-3 Next-Generation Topography mission and has chosen to develop a solution involving both nadir and wide-swath altimeters. While the experiments detailed here show a superior impact from an additional 12 nadir altimeters compared to 2 wide-swath altimeters, we still show promising impacts from the new wide-swath altimeters. It must also be noted that the results from OSSEs provide an estimate of the impact in the specific system tested. These results do not reflect the best achievable impact but rather an estimate of the impact in our current system. Future model and assimilation changes tailored to the observation network are expected to lead to a greater impact. Work is now underway to prepare our operational systems to assimilate real data from the SWOT wide-swath altimeter mission, including accounting for correlated errors in the assimilation scheme, which will test our ability to make good use of wide-swath altimeter data to deliver improved ocean forecasts to users.
The nature of the 4D data generated in running the model experiments requires a large tape storage facility. These data are of the order of 300 TB (terabytes). The model data can be made available upon request from the authors.
RRK, MJM, LG, JW, CU, and CD contributed to the design of the project. RRK, MJM, and JW worked on the experimental design; RRK ran the experiments; LG simulated the observations; RRK, MJM, JW, and CU contributed to the analysis; and the article writing was led by RRK and MJM. All authors have read and agreed to the published version of the article.
The contact author has declared that none of the authors has any competing interests.
Publisher’s note: Copernicus Publications remains neutral with regard to jurisdictional claims made in the text, published maps, institutional affiliations, or any other geographical representation in this paper. While Copernicus Publications makes every effort to include appropriate place names, the final responsibility lies with the authors.
Funding support from the European Space Agency is gratefully acknowledged. We also acknowledge our collaborators in developing NEMOVAR at CERFACS, INRIA, and ECMWF. We would like to thank G. C. Smith and another anonymous reviewer for their time and effort in reviewing our article, which helped us to revise and improve it.
This research has been supported by the European Space Agency (grant no. 4000130863/20/NL/IA).
This paper was edited by Mehmet Ilicak and reviewed by G. C. Smith and one anonymous referee.
Aijaz, S., Brassington, G. B., Divakaran, P., Regnier, C., Drevillon, M., Maksymczuk, J., and Peterson, K. A.: Verification and intercomparison of global ocean Eulerian near-surface currents, Ocean Model., 186, 102241, https://doi.org/10.1016/j.ocemod.2023.102241, 2023. a
Ballarotta, M., Ubelmann, C., Pujol, M.-I., Taburet, G., Fournier, F., Legeais, J.-F., Faugère, Y., Delepoulle, A., Chelton, D., Dibarboure, G., and Picot, N.: On the resolutions of ocean altimetry maps, Ocean Sci., 15, 1091–1109, https://doi.org/10.5194/os-15-1091-2019, 2019. a, b, c
Ballarotta, M., Albert, A., Ajayi, A., Beauchamp, M., Cosme, E., Le Sommer, J., and Metref, S.: Ocean Data Challenge 2020a_SSH_mapping_NATL60, GitHub [code], https://github.com/ocean-data-challenges/2020a_SSH_mapping_NATL60 (last access: 9 December 2024), 2020.
Barbosa Aguiar, A., Bell, M. J., Blockley, E., Calvert, D., Crocker, R., Inverarity, G., King, R., Lea, D. J., Maksymczuk, J., Martin, M. J., Price, M. R., Siddorn, J., Smout-Day, K., Waters, J., and While, J.: The Met Office Forecast Ocean Assimilation Model (FOAM) using a 1/12-degree grid for global forecasts, Q. J. Roy. Meteorol. Soc., 150, 3827–3852, https://doi.org/10.1002/qj.4798, 2024. a
Benkiran, M., Ruggiero, G., Greiner, E., Le Traon, P.-Y., Remy, E., Lellouche, J. M., Bourdalle-Badie, R., Drillet, Y., and Tchonang, B.: Assessing the Impact of the Assimilation of SWOT Observations in a Global High-Resolution Analysis and Forecasting System – Part 1: Methods, Front. Mar. Sci., 8, 691955, https://doi.org/10.3389/fmars.2021.691955, 2021. a
Benkiran, M., Le Traon, P.-Y., Rémy, E., and Drillet, Y.: Impact of two high resolution altimetry mission concepts for ocean forecasting, EGUsphere [preprint], https://doi.org/10.5194/egusphere-2024-420, 2024. a, b
Bloom, S. C., Takacs, L. L., da Silva, A. M., and Ledvina, D.: Data Assimilation Using Incremental Analysis Updates, Mon. Wea. Rev., 124, 1256–1271, https://doi.org/10.1175/1520-0493(1996)124<1256:DAUIAU>2.0.CO;2, 1996. a
Davidson, F., Alvera-Azcárate, A., Barth, A., Brassington, G. B., Chassignet, E. P., Clementi, E., De Mey-Frémaux, P., Divakaran, P., Harris, C., Hernandez, F., Hogan, P., Hole, L. R., Holt, J., Liu, G., Lu, Y., Lorente, P., Maksymczuk, J., Martin, M., Mehra, A., Melsom, A., Mo, H., Moore, A., Oddo, P., Pascual, A., Pequignet, A.-C., Kourafalou, V., Ryan, A., Siddorn, J., Smith, G., Spindler, D., Spindler, T., Stanev, E. V., Staneva, J., Storto, A., Tanajura, C., Vinayachandran, P. N., Wan, L., Wang, H., Zhang, Y., Zhu, X., and Zu, Z.: Synergies in Operational Oceanography: The Intrinsic Need for Sustained Ocean Observations, Front. Mar. Sci., 6, 450, https://doi.org/10.3389/fmars.2019.00450, 2019. a
Dibarboure, G., Ubelmann, C., Flamant, B., Briol, F., Peral, E., Bracher, G., Vergara, O., Faugère, Y., Soulat, F., and Picot, N.: Data-driven calibration algorithm and pre-launch performance simulations for the swot mission, Remote Sens., 14, 6070, https://doi.org/10.3390/rs14236070, 2022. a
Esteban-Fernandez, D.: Swot Mission Performance and Error Budget, IGARSS 2018 – 2018 IEEE International Geoscience and Remote Sensing Symposium, Valencia, Spain, 2018, 8625–8628, https://doi.org/10.1109/IGARSS.2018.8517385, 2018. a
Fujii, Y., Rémy, E., Zuo, H., Oke, P., Halliwell, G., Gasparin, F., Benkiran, M., Loose, N., Cummings, J., Xie, J., Xue, Y., Masuda, S., Smith, G., Balmaseda, M., Germineaud, C., Lea, D., Larnicol, G., Bertino, L., Bonaduce, A., Brasseur, P., Donlon, C., Heimbach, P., Kim, Y., Kourafalou, V., Le Traon, P.-Y., Martin, M., Paturi, S., Tranchant, B., and Usui, N.: Observing system evaluation based on ocean data assimilation and prediction systems: On-going challenges and a future vision for designing and supporting ocean observational networks, Front. Mar. Sci., 6, 417, https://doi.org/10.3389/fmars.2019.00417, 2019. a, b
Gasparin, F., Greiner, E., Lellouche, J.-M., Legalloudec, O., Garric, G., Drillet, Y., Bourdallé-Badie, R., Traon, P.-Y. L., Rémy, E., and Drévillon, M.: A large-scale view of oceanic variability from 2007 to 2015 in the global high resolution monitoring and forecasting system at Mercator Océan, J. Mar. Syst., 187, 260–276, https://doi.org/10.1016/j.jmarsys.2018.06.015, 2018. a
Gasparin, F., Guinehut, S., Mao, C., Mirouze, I., Rémy, E., King, R. R., Hamon, M., Reid, R., Storto, A., Le Traon, P.-Y., and Martin, M.: Requirements for an integrated in situ Atlantic Ocean observing system from coordinated observing system simulation experiments, Front. Mar. Sci., 6, 83, https://doi.org/10.3389/fmars.2019.00083, 2019. a, b, c, d
Gaultier, L., Ubelmann, C., and Fu, L.-L.: The challenge of using future SWOT data for oceanic field reconstruction, J. Atmos. Ocean. Technol., 33, 119–126, https://doi.org/10.1175/JTECH-D-15-0160.1, 2016. a, b, c
Good, S. A., Martin, M. J., and Rayner, N. A.: EN4: Quality controlled ocean temperature and salinity profiles and monthly objective analyses with uncertainty estimates, J. Geophys. Res.-Ocean., 118, 6704–6716, https://doi.org/10.1002/2013JC009067, 2013. a
Guiavarc'h, C., Roberts-Jones, J., Harris, C., Lea, D. J., Ryan, A., and Ascione, I.: Assessment of ocean analysis and forecast from an atmosphere–ocean coupled data assimilation operational system, Ocean Sci., 15, 1307–1326, https://doi.org/10.5194/os-15-1307-2019, 2019. a
Guillet, O., Weaver, A. T., Vasseur, X., Michel, Y., Gratton, S., and Gürol, S.: Modelling spatially correlated observation errors in variational data assimilation using a diffusion operator on an unstructured mesh, Q. J. Roy. Meteorol. Soc., 145, 1947–1967, https://doi.org/10.1002/qj.3537, 2019. a, b
Halliwell, G. R., Mehari, M. F., Le Hénaff, M., Kourafalou, V. H., Androulidakis, I. S., Kang, H. S., and Atlas, R.: North Atlantic Ocean OSSE system: Evaluation of operational ocean observing system components and supplemental seasonal observations for potentially improving tropical cyclone prediction in coupled systems, J. Oper. Oceanogr., 10, 154–175, https://doi.org/10.1080/1755876X.2017.1322770, 2017. a, b
Hersbach, H., Bell, B., Berrisford, P., Hirahara, S., Horányi, A., Muñoz-Sabater, J., Nicolas, J., Peubey, C., Radu, R., Schepers, D., Simmons, A., Soci, C., Abdalla, S., Abellan, X., Balsamo, G., Bechtold, P., Biavati, G., Bidlot, J., Bonavita, M., De Chiara, G., Dahlgren, P., Dee, D., Diamantakis, M., Dragani, R., Flemming, J., Forbes, R., Fuentes, M., Geer, A., Haimberger, L., Healy, S., Hogan, R. J., Hólm, E., Janisková, M., Keeley, S., Laloyaux, P., Lopez, P., Lupu, C., Radnoti, G., de Rosnay, P., Rozum, I., Vamborg, F., Villaume, S., and Thépaut, J.-N.: The ERA5 global reanalysis, Q. J. Roy. Meteorol. Soc., 146, 1999–2049, https://doi.org/10.1002/qj.3803, 2020. a
Hoffman, R. N. and Atlas, R.: Future observing system simulation experiments, Bull. Am. Meteorol. Soc., 97, 1601–1616, https://doi.org/10.1175/BAMS-D-15-00200.1, 2016. a
Hunke, E. C. and Lipscombe, W. H.: CICE: The Los Alamos sea ice model, Documentation and software users manual, Version 4.1 (LA-CC-06012), T-3 Fluid Dynamics Group, Los Alamos National Laboratory, Los Alamos, US, https://csdms.colorado.edu/w/images/CICE_documentation_and_software_user's_manual.pdf (last access: 9 December 2024), 2010. a
King, R. R. and Martin, M. J.: Assimilating realistically simulated wide-swath altimeter observations in a high-resolution shelf-seas forecasting system, Ocean Sci., 17, 1791–1813, https://doi.org/10.5194/os-17-1791-2021, 2021. a, b, c
King, R. R., While, J., Martin, M. J., Lea, D. J., Lemieux-Dudon, B., Waters, J., and O'Dea, E.: Improving the initialisation of the Met Office operational shelf-seas model, Ocean Model., 130, 1–14, https://doi.org/10.1016/j.ocemod.2018.07.004, 2018. a
Le Guillou, F., Metref, S., Cosme, E., Ubelmann, C., Ballarotta, M., Le Sommer, J., and Verron, J.: Mapping altimetry in the forthcoming swot era by back-and-forth nudging a one-layer quasigeostrophic model, J. Atmos. Ocean. Technol., 38, 697–710, https://doi.org/10.1175/JTECH-D-20-0104.1, 2021. a
Le Traon, P.-Y., Dibarboure, G., Jacobs, G., Martin, M., Rémy, E., and Schiller, A.: Use of satellite altimetry for operational oceanography, in: Satellite altimetry over oceans and land surfaces, 581–608, CRC Press, https://doi.org/10.1201/9781315151779-18, 2017. a
Lea, D., Drecourt, J.-P., Haines, K., and Martin, M.: Ocean altimeter assimilation with observational-and model-bias correction, Quarterly J. Roy. Meteorol. Soc., 134, 1761–1774, https://doi.org/10.1002/qj.320, 2008. a
Lea, D. J., Mirouze, I., Martin, M. J., King, R. R., Hines, A., Walters, D., and Thurlow, M.: Assessing a New Coupled Data Assimilation System Based on the Met Office Coupled Atmosphere-Land-Ocean-Sea Ice Model, Mon. Weather Rev., 143, 4678–4694, https://doi.org/10.1175/MWR-D-15-0174.1, 2015. a
Lellouche, J.-M., Greiner, E., Le Galloudec, O., Garric, G., Regnier, C., Drevillon, M., Benkiran, M., Testut, C.-E., Bourdalle-Badie, R., Gasparin, F., Olga Hernandez, O., Levier, B., Drillet, Y., Remy, E., and Le Traon, P.-Y.: Recent updates to the Copernicus Marine Service global ocean monitoring and forecasting real-time ° high-resolution system, Ocean Sci., 14, 1093–1126, https://doi.org/10.5194/os-14-1093-2018, 2018. a
MacLachlan, C., Arribas, A., Peterson, K. A., Maidens, A., Fereday, D., Scaife, A. A., Gordon, M., Vellinga, M., Williams, A., Comer, R. E., Camp, J., Xavier, P., and Madec, G.: Global Seasonal forecast system version 5 (GloSea5): a high-resolution seasonal forecast system, Q. J. Roy. Meteorol. Soc., 141, 1072–1084, https://doi.org/10.1002/qj.2396, 2015. a
Madec, G., Bourdallé-Badie, R., Chanut, J., Clementi, E., Coward, A., Ethé, C., Iovino, D., Lea, D., Lévy, C., Lovato, T., Martin, N., Masson, S., Mocavero, S., Rousset, C., Storkey, D., Müeller, S., Nurser, G., Bell, M., Samson, G., Mathiot, P., Mele, F., and Moulin, A.: NEMO ocean engine, Zenodo, https://doi.org/10.5281/zenodo.6334656, 2022. a
Mao, C., King, R. R., Reid, R., Martin, M. J., and Good, S. A.: Assessing the Potential Impact of Changes to the Argo and Moored Buoy Arrays in an Operational Ocean Analysis System, Front. Mar. Sci., 7, 588267, https://doi.org/10.3389/fmars.2020.588267, 2020. a, b
Martin, M. J., Remy, E., Tranchant, B., King, R. R., Greiner, E., and Donlon, C.: Observation impact statement on satellite sea surface salinity data from two operational global ocean forecasting systems, J. Oper. Oceanogr., 15, 87–103, https://doi.org/10.1080/1755876X.2020.1771815, 2020. a
Mignac, D., Waters, J., Lea, D. J., Martin, M. J., While, J., Weaver, A. T., Vidard, A., Guiavarc’h, C., Storkey, D., Ford, D., Blockley, E. W., Baker, J., Haines, K., Price, M. R., Bell, M. J., and Renshaw, R.: Updates to the Met Office’s global ocean-sea ice forecasting system including model and data assimilation changes, EGUsphere [preprint], https://doi.org/10.5194/egusphere-2024-3143, 2024. a
Mirouze, I., Blockley, E. W., Lea, D. J., Martin, M. J., and Bell, M. J.: A multiple length scale correlation operator for ocean data assimilation, Tellus A, 68, 29744, https://doi.org/10.3402/tellusa.v68.29744, 2016. a, b
Morrow, R., Fu, L.-L., Ardhuin, F., Benkiran, M., Chapron, B., Cosme, E., d’Ovidio, F., Farrar, J. T., Gille, S. T., Lapeyre, G., Le Traon, P.-Y., Pascual, A., Ponte, A., Qiu, B., Rascle, N., Ubelmann, C., Wang, J., and Zaron, E. D.: Global Observations of Fine-Scale Ocean Surface Topography With the Surface Water and Ocean Topography (SWOT) Mission, Front. Mar. Sci., 6, 232, https://doi.org/10.3389/fmars.2019.00232, 2019. a
Oke, P. R. and O'Kane, T. J.: Observing system design and assessment, Operational Oceanography in the 21st Century, Springer, 123–151, https://doi.org/10.1007/978-94-007-0332-2_5, 2011. a
Peral, E., Rodríguez, E., and Esteban-Fernández, D.: Impact of surface waves on SWOT's projected ocean accuracy, Remote Sens., 7, 14509–14529, https://doi.org/10.3390/rs71114509, 2015. a
Pujol, M.-I., Dupuy, S., Vergara, O., Sánchez Román, A., Faugère, Y., Prandi, P., Dabat, M.-L., Dagneaux, Q., Lievin, M., Cadier, E., Dibarboure, G., and Picot, N.: Refining the Resolution of DUACS Along-Track Level-3 Sea Level Altimetry Products, Remote Sens., 15, 793, https://doi.org/10.3390/rs15030793, 2023. a
Ridley, J. K., Blockley, E. W., Keen, A. B., Rae, J. G., West, A. E., and Schroeder, D.: The sea ice model component of HadGEM3-GC3. 1, Geosci. Model Dev., 11, 713–723, https://doi.org/10.5194/gmd-11-713-2018, 2018. a
Rossa, A., Nurmi, P., and Ebert, E.: Overview of methods for the verification of quantitative precipitation forecasts, in: Precipitation: Advances in measurement, estimation and prediction, Springer, 419–452, https://doi.org/10.1007/978-3-540-77655-0_16, 2008. a
Storkey, D., Blaker, A. T., Mathiot, P., Megann, A., Aksenov, Y., Blockley, E. W., Calvert, D., Graham, T., Hewitt, H. T., Hyder, P., Kuhlbrodt, T., Rae, J. G. L., and Sinha, B.: UK Global Ocean GO6 and GO7: a traceable hierarchy of model resolutions, Geosci. Model Dev., 11, 3187–3213, https://doi.org/10.5194/gmd-11-3187-2018, 2018. a
Tchonang, B. C., Benkiran, M., Le Traon, P.-Y., Jan van Gennip, S., Lellouche, J. M., and Ruggiero, G.: Assessing the Impact of the Assimilation of SWOT Observations in a Global High-Resolution Analysis and Forecasting System 2̆013 Part 2: Results, Front. Mar. Sci., 8, 1208, https://doi.org/10.3389/fmars.2021.687414, 2021. a
Tonani, M., Sykes, P., King, R. R., McConnell, N., Péquignet, A.-C., O'Dea, E., Graham, J. A., Polton, J., and Siddorn, J.: The impact of a new high-resolution ocean model on the Met Office North-West European Shelf forecasting system, Ocean Sci., 15, 1133–1158, https://doi.org/10.5194/os-15-1133-2019, 2019. a
Waters, J., Lea, D. J., Martin, M. J., Mirouze, I., Weaver, A., and While, J.: Implementing a variational data assimilation system in an operational ° global ocean model, Q. J. Roy. Meteorol. Soc., 141, 333–349, https://doi.org/10.1002/qj.2388, 2015. a, b
Weaver, A. T., Deltel, C., Machu, É., Ricci, S., and Daget, N.: A multivariate balance operator for variational ocean data assimilation, Quarterly Journal of the Royal Meteorological Society: A journal of the atmospheric sciences, Appl. Meteorol. Phys. Oceanogr., 131, 3605–3625, https://doi.org/10.1256/qj.05.119, 2005. a
Weaver, A. T., Tshimanga, J., and Piacentini, A.: Correlation operators based on an implicitly formulated diffusion equation solved with the Chebyshev iteration, Q. J. Roy. Meteorol. Soc., 142, 455–471, https://doi.org/10.1002/qj.2664, 2016. a
While, J. and Martin, M. J.: Variational bias correction of satellite sea-surface temperature data incorporating observations of the bias, Q. J. Roy. Meteorol. Soc., 145, 2733–2754, https://doi.org/10.1002/qj.3590, 2019. a