the Creative Commons Attribution 4.0 License.
the Creative Commons Attribution 4.0 License.
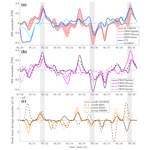
Influence of river runoff and precipitation on the seasonal and interannual variability of sea surface salinity in the eastern North Tropical Atlantic
Clovis Thouvenin-Masson
Vincent Échevin
Alban Lazar
Jean-Luc Vergely
In tropical regions, the freshwater flux entering the ocean originates primarily from precipitation and, to a lesser extent when considering basin-scale averages, from continental rivers. Nevertheless, at the regional scale, river flows can have a significant impact on the surface ocean dynamics. Riverine freshwater modifies salinity and, therefore, density, stratification, and circulation. With its particular coastline and high cumulative river discharge, as well as its being in the vicinity of the intertropical convergence zone (ITCZ), the eastern part of the North Tropical Atlantic (e-NTA) region off northwestern Africa is a particularly interesting location to study the linkage between precipitation, river outflow, and sea surface salinity (SSS). Here, we focus on the regional e-NTA SSS seasonal cycle and interannual variability and on the impact of using various river runoff and precipitation forcing data sets to simulate SSS with a regional model. The simulated SSS values are compared with the Climate Change Initiative (CCI) satellite SSS values; in situ SSS values from Argo floats, ships, and a coastal mooring; and the GLORYS reanalysis SSS values. An analysis of the mixed-layer salinity budget is then conducted. Overall, the simulations reproduce the seasonal cycle and interannual variability well despite a positive mean model bias north of 15° N. The seasonal cycle is impacted by the phasing of the different runoff products. The mixed-layer SSS decrease during the rainy season is mainly driven by precipitation followed by runoff by means of horizontal advection and is partly compensated for by vertical mixing. In terms of interannual anomalies, river runoff has a more direct impact on SSS than precipitation. This study highlights the importance of properly constraining river runoff and precipitation to simulate realistic SSS values and the importance of observing SSS in coastal regions to validate such constraints.
- Article
(8702 KB) - Full-text XML
-
Supplement
(1469 KB) - BibTeX
- EndNote
The upper layer of the ocean is where exchanges between the ocean and the atmosphere take place. Air–sea forcing (e.g., wind, heat flux) generates turbulence in the surface layer, leading to the formation of a surface mixed layer from a few meters to hundreds of meters thick, with homogeneous characteristics (e.g., temperature and salinity) and whose bottom is characterized by a marked density gradient, the pycnocline. This layer receives various freshwater flows, such as precipitation or river discharge. The input of these low-salinity waters lowers the density of the surface waters, which can lead to an increase in the density gradient between the surface and subsurface waters. Freshwater inputs can also generate significant salinity gradients within the mixed layer (Mignot et al., 2007), leading to the formation of intermediate layers known as barrier layers. The latter isolate the surface layer from the deep ocean, inhibiting heat exchange between the ocean surface and subsurface (Vialard and Delecluse, 1998). Such ocean–atmosphere interactions might impact the formation of water masses and their evolution, as well as air–sea exchanges of heat and gases. Since the 1980s, the quality and availability of in situ river discharge measurements have declined due to a lack of funding and an unwillingness by institutions to share these data with the general public (Chandanpurkar et al., 2017; Durand et al., 2019). As a result, current ocean general circulation models (OGCMs) such as those used to generate the GLORYS reanalysis (Lellouche et al., 2021) typically utilize climatological river discharge products (Dai et al., 2009), which have not been updated for more than a decade. However, it has been shown that river discharges tend to vary strongly interannually. Gévaudan et al. (2022) found that the Amazon River runoff anomalies can reach values of the order of 50 000 m3 s−1 (25 % of the climatological value) and that these anomalies have a significant influence on the surface salinity of the tropical Atlantic Ocean. Chandanpurkar et al. (2022) studied the influence of river discharge interannual variability on salinity at the mouths of the world's major rivers. They found that river discharge interannual variability is responsible for a standard deviation of 1.3 to 3 pss of salinity and that models that take interannual variations of river discharge into account simulate sea surface salinity (SSS) values that are closer to those of satellite observations. At the scale of the global ocean, a recent study (Fournier et al., 2023) demonstrates that SSS variability, averaged over estuarine regions of major river plumes, is strongly correlated with the global water cycle variability, particularly in relation to the El Niño–Southern Oscillation (ENSO) phenomenon.
In this paper, we focus on the eastern North Tropical Atlantic (e-NTA; 10–17° N, 20–12° W; Fig. 1), a region subject to high river discharge forcings, resulting from rainfall over the high mountain plateaus of Guinea. There is a geographical disparity in the river flows: to the north of Dakar (14.7° N), only the Senegal River has an average outflow of over 500 m3 s−1 (Roudier et al., 2014), whereas, to the south of Dakar, freshwater discharge takes place through numerous rivers along the coast, with the Gambia River being the most significant. River flow in the e-NTA is highly seasonal, with rivers that run almost dry during boreal summer and peak in autumn after the rainy season. While their interannual variations are not well known due to a lack of data, they are expected to be strongly influenced by the West African monsoon, with large interannual variations that are expected to increase by 10 % to 28 % with climate change (Akinsanola, 2020). Moreover, studies based on climate models (Ardoin-Bardin et al., 2009) predict a long-term decreasing trend for these river flows of up to 27 % for the Senegal River and up to 37 % for the Gambia River by 2080.
Cumulating all the river discharges of Senegal and Guinea (12–17° N) leads to an average monthly outflow of ∼ 30 000 m3 s−1 at its annual maximum in September. In comparison, the largest Amazon outflow in May is 276 000 m3 s−1, and the largest outflow of the Congo River is 56 000 m3 s−1 in December (Wohl and Lininger, 2022). However, the e-NTA region is of particular interest because it is subject to both river discharge and intense precipitation linked to the meridional displacements of the intertropical convergence zone (ITCZ). Furthermore, this region hosts the strong Senegalese coastal upwelling, a region where human populations are highly dependent on small pelagic fisheries as a source of protein (Failler et al., 2014).
The aforementioned studies demonstrate the usefulness of salinity as a tracer for variations in the water cycle from the perspective of the seasonal cycle and interannual variability near major rivers. Concerning the impact of freshwater fluxes on the salinity in the e-NTA region, only the seasonal variations and their driving physical processes have been studied by Camara et al. (2015) using the Nucleus for European Modelling of the Ocean (NEMO) ocean model. They found that runoff and precipitation were the main contributors to the freshening in the e-NTA and that poleward advection of low-salinity waters along the coasts was partly compensated for by vertical diffusion of salinity. However, to our knowledge, no study has yet focused on interannual variability in this region or on the sensitivity of the simulated salinity to the runoff and precipitation forcing data sets.
These are the goals of this study, in which we aim to (i) differentiate the effects of precipitation from those of river discharge on coastal salinity in the e-NTA region and (ii) contrast the effects of different precipitation and runoff data sets on the simulated salinity. To achieve these goals, the surface ocean dynamics are simulated by the Coastal and Regional Ocean Community (CROCO) model, with various configurations of climatological or interannual forcings. The model results are compared with Mercator's GLORYS reanalysis, satellite, and in situ SSS measurements (e.g., merchant ships, Argo floats, buoys). We estimate the seasonal cycle and interannual variation in salinity for each configuration and intercompare these different configurations. Using a mixed-layer salinity balance, we identify the mechanisms through which river runoff and precipitation alter the simulated SSS, employing a methodology similar to that of Camara et al. (2015).
Section 2 presents the data and the methods used. Section 3 is dedicated to the results and includes a validation of the modeled SSS, an analysis of the observed and modeled SSS anomalies, and a study of the modeled SSS sensitivity to changes in freshwater flux forcings. Finally, a few points are raised for discussion and conclusions are given in Sect. 4.
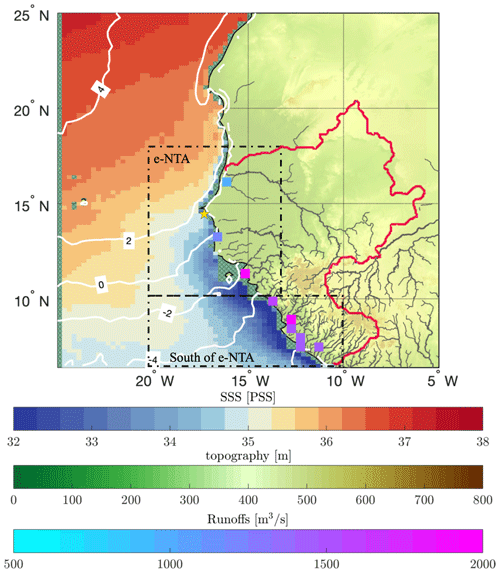
Figure 1CCI satellite SSS values (color) averaged over October–November–December of the years 2010 to 2019 (over ocean); white contours indicate the averaged ERA5 E−P (evaporation minus precipitation) rate (in mm d−1) over this period. Topography (color scale) is shown on land. Along the coast, colored squares indicate averaged ISBA river runoff over September–October–November (color scale). The area delimited by the red line corresponds to the merged catchment areas of the rivers flowing into the area of study, extracted from the HydroSHEDS database (hydrological data and maps are based on SHuttle Elevation Derivatives). Dotted black boxes delimit the e-NTA and the south of the e-NTA region. The yellow star represents the position of the Melax buoy.
The region we focus on is identical to the one studied in Camara et al. (2015) for the purpose of comparing the results obtained. We refer to it as the eastern North Tropical Atlantic (e-NTA). This region is strongly impacted by river water outflows as it includes major rivers (Senegal, Gambia, Casamance, the Big and Little Scarcies; see Fig. 1). In the following, we study the salinity and freshwater forcing variables averaged over this region. We focus on the longest common period for which all salinity and forcing products are available: from 1 January 2010, before which satellite salinity products are not available, to 19 June 2019, after which the ISBA-CTRIP product is no longer available.
2.1 Salinity data
2.1.1 Satellite maps
Three L-band radiometric satellite missions have measured SSS from space: SMOS (2010–present), SMAP (2015–present), and Aquarius (2012–2015). Version 3.2 of the SSS product generated as part of the Climate Change Initiative (CCI) project is used here, covering a period from 2010 to 2021 (Boutin et al., 2021a). These data are generated with a temporal optimal interpolation of the three satellite measurements, as described for version 2 of the CCI+SSS project in Boutin et al. (2021b). Developments between version 2 and version 3 are described in detail in Thouvenin-Masson et al. (2022). SSS fields are available on a 25 km Equal-Area Scalable Earth Grid (EASE-Grid2), and they are used here at a weekly temporal resolution. Due to the spatial resolution of satellite SSS measurements, data obtained less than ∼ 40 km from the coast are flagged as they must be considered with caution due to land contamination (e.g., Zine et al., 2008). This flag filtering is applied in the present study. In this satellite product, a correction is applied to remove the instantaneous effect of rain on the top surface satellite measurements to remain consistent with the bulk salinity recorded by most in situ instruments (Supply et al., 2020).
2.1.2 GLORYS reanalysis
The GLORYS12V1 product is a Copernicus Marine Environment Monitoring Service (CMEMS) global ocean eddy-resolving reanalysis available at a daily resolution from 1993 to 2023. This reanalysis is based on the NEMO ocean model forced by ERA5 data and by climatological river runoff at the surface. Satellite sea level anomalies, sea surface temperature (SST), sea ice concentration, in situ temperature, and salinity vertical profiles (but not satellite SSS) are assimilated using a reduced-order Kalman filter derived from a singular evolutive extended Kalman (SEEK) filter with a three-dimensional multivariate background error covariance matrix and a 7 d assimilation cycle (Lellouche et al., 2018, 2021). Model reanalysis output is available at a daily temporal resolution on a regular ° grid for 50 vertical levels. See Lellouche et al. (2021) for a complete description of the model.
2.1.3 In situ data
In situ data are used to evaluate the CROCO simulations. This involves measurements from thermosalinographs (TSGs) installed on merchant and research vessels, from Argo floats near the surface, and from the Melax mooring.
With regard to TSGs, the delayed-mode data set from Laboratoire d'Etudes en Géophysique et Océanographie Spatiales (TSG-LEGOS-DM) is used. It is derived from voluntarily observing ships, which are collected, validated, archived, and made freely available by the French Sea Surface Salinity Observation Service (Alory et al., 2015). Only adjusted values, when available, and TSG data with quality flags of 1 and 2 (“good” or “probably good”) are selected. TSG data are available from 1993 to present at depths of between 5 to 15 m, and we use the hourly resolution product.
The Argo project is a set of about 4000 profilers moving in the global ocean. These instruments provide around 100 000 temperature and salinity measurements annually over the global ocean, with an average spacing of 3° between measurements (Argo, 2023). These data are collected and made freely available by the international Argo project and the national programs that contribute to it. The Argo SSS data gathered in the Salinity Pilot-Mission Exploitation Platform (Pi-MEP) database (Guimbard et al., 2021) are used. In this database, Argo data from the Global Data Assembly Centre (GDAC) database (Argo, 2023) with a quality index of 1 or 2 are selected. Argo measurements between 10 and 0 m depth are considered to be surface data (most of the Argo data resulting from this selection are obtained from a depth of about 5 m).
Finally, the Melax mooring is equipped with oceanographic and atmospheric sensors. Moored at 36 m depth, it is located 30 km from the coast. It measures the physical and biogeochemical parameters over the Senegalese shelf (14°20′ N, 17°14′ W), south of the city of Dakar (Tall et al., 2021, Fig. 1). The mooring captured surface salinity almost continuously from mid-February 2015 to August 2016. We use the Melax measurements averaged daily over this time period.
2.2 Regional simulations: the CROCO model
The ocean model CROCO (https://www.CROCO-ocean.org/, last access: 18 February 2023, Hilt et al., 2020) is used to simulate salinity variations in the e-NTA region. CROCO has vertical sigma coordinates, which are well suited for coastal studies. The slow mode and the fast barotropic mode are computed separately using a time-splitting algorithm (Shchepetkin and McWilliams, 2009), improving the consistency, accuracy, and stability of the simulations. High-order numerical schemes enable the representation of small-scale structures such as mesoscale eddies and filaments. The AGRIF (adaptive refinement of the horizontal grid; Debreu et al., 2008) module is utilized, enabling the embedment of a sub-domain in which small scales are more finely resolved. In the configuration used here, the parent grid covering 7–35° N and 30–10° W has a resolution of 10 km, and the child grid used in the Senegal region (12–18° N, 20–15° W) has a resolution of 2 km. More details on the model configuration can be found in Ndoye et al. (2018). Daily outputs from the Mercator model output at ° resolution (GLOBAL_ANALYSISFORECAST_PHY_001_024; downloaded from http://marine.copernicus.eu/, last access: 13 March 2023) are used to force physical properties (temperature, salinity, velocity, and sea level) as open boundary conditions (OBCs) of the parent grid.
Hourly atmospheric forcings (air temperature, relative humidity, 10 m wind, radiative fluxes) from the ERA5 reanalysis (see below) are used in all simulations. No surface salinity restoring with climatological observations (e.g., Ndoye et al., 2018) is used.
In order to estimate the relative importance of interannual variations in each of the freshwater flux forcings, five CROCO simulations are performed with different rain rate and river runoff forcings. The other hourly forcing terms (air temperature, wind, radiative flux, etc.) are kept identical for all simulations. Three simulations are forced with synoptic freshwater flux forcings, including interannual variations. These simulations are called CROCOglofas, CROCOisba, and CROCOimerg. Two simulations are forced with climatological rain rates or climatological river outflows. These are named CROCOprclm and CROCOroclm, respectively. The forcings of each simulation are summarized in Table 1.
2.2.1 Precipitation and runoff forcings
ERA-5 is a reanalysis produced by the European Centre for Medium-Range Weather Forecasts (ECMWF), which provides comprehensive modeling of atmospheric, continental surface, and ocean wave variables (Hersbach et al., 2020). Based on the Cycle 41r2 Integrated Forecast System (IFS), ERA5 hourly fields are available over the period 1950–2023 at a horizontal resolution of 31 km.
The precipitation value used in the CROCO simulations is composed of the convective precipitation field (cp) produced by the IFS convection scheme, which represents precipitation at sub-grid scales, and the stratiform precipitation field (sp) produced by the IFS cloud model, which represents the formation and dissipation of clouds and large-scale precipitation due to changes in atmospheric variables such as pressure, temperature, and humidity.
Integrated Multi-satellitE Retrievals for Global Precipitation Measurement (IMERG) is a rain rate product based on satellite precipitation measurements. It combines information from the Global Precipitation Measurement (GPM) satellite constellation with infrared (IR) satellite data obtained by geostationary satellites to estimate precipitation over the majority of the Earth's surface at a frequency of 30 min. The algorithm is based on the Climate Prediction Center morphing (CMORPH; Joyce et al., 2004) method and takes advantage of the high repetition rate of IR satellites to track the movement of less frequent but more accurate microwave- and radar-detected rainfall cells. IMERG data are available from 2000 to present at a resolution of 0.1° every half-hour.
Over the ocean, these two precipitation products are consistent in terms of mean values and have similar climatologies and anomalies (Figs. 6c, 3b) after integration over the e-NTA. Nevertheless, IMERG rain rates are more variable locally and extend over a larger range of values than ERA5 rain rates (see Figs. S1 and S3 in the Supplement, blue and red curves).
The Global Flood Awareness System (GloFAS; http://www.globalfloods.eu/, last access: 12 January 2023; Harrigan et al., 2020) is one of the components of the Copernicus Emergency Management Service (CEMS). This system is designed to help prevent flooding on a global scale, notably by providing water level forecasts for river basins. It is based on satellite data, on soil temperature and humidity, on precipitation from ERA5, and on in situ data. These data are integrated into the Hydrology in the Tiled ECMWF Scheme for Surface Exchanges over Land (HTESSEL) continental surface model, which is part of the ECMWF's integrated forecasting system (IFS 41r2), via a terrestrial data assimilation system explained in de Rosnay et al. (2012). The resulting runoff is then integrated into the LISFLOOD runoff routing model. The GloFAS hydrological model simulations are available from 1979 to present at a daily and 0.1° resolution.
ISBA-CTRIP river discharge estimation is used in this study. ISBA-CTRIP combines two models: the Interaction Soil-Biosphere-Atmosphere (ISBA; https://www.umr-cnrm.fr/isbadoc/model.html, last access: 13 January 2023) hydrological model developed by the Centre National de Recherches Météorologiques (CNRM) within the framework of the IPCC (see Decharme et al., 2019, for a full description of the model) and the CTRIP (CNRM version of Total Runoff Integrating Pathways) model, which is an improved version of the TRIP model used to simulate river runoff to the ocean from the total runoff calculated by ISBA. In the configuration used here, this model uses tier-2 water resources reanalysis (WRR2) precipitation at 0.25° resolution from the E2O project as forcing. The E2O data set is directly based on the 3-hourly ERA-Interim reanalysis (https://www.ecmwf.int/en/forecasts/datasets/reanalysis-datasets/era-interim, last access: 14 March 2023) over the 1979–2014 period. Precipitation has been hybridized with observations using the Multi-Source Weighted-Ensemble Precipitation (MSWEP; http://www.gloh2o.org, last access: 5 December 2022) data set (Beck et al., 2017). ISBA-CTRIP data are available daily from 1979 to 9 June 2019 at a 0.5° resolution.
GloFAS runoff and ISBA runoff, after summing the individual outflows for the region studied, have similar climatologies (maximum difference of 1×108 m3 d−1; see Fig. 3b). The simulated river runoff exhibits strong interannual anomalies in this area (Fig. 2). These river runoff anomalies are strongly correlated with African monsoon variations, as shown in Fig. 2, where interannual anomalies of modeled runoff closely mirror the interannual anomalies of precipitation over the watershed, used as forcing in these models. These interannual anomalies can reach 8×108 m3 d−1, i.e., almost 40 % of the seasonal variation (Fig. 3b). They are sometimes of opposite signs between the two products, with differences reaching 1×109 m3 d−1 (Fig. 2). These differences and their origins are discussed in Sect. 4.
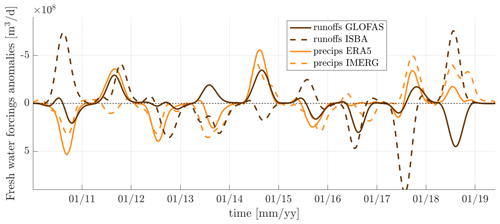
Figure 2Runoff anomalies (brown) and watershed precipitation anomalies (orange) for GloFAS and ERA5 (solid lines) and ISBA and IMERG (dashed lines) over the catchment areas of the rivers flowing through the study region (see Fig. 1 for catchment delimitation). Note that the y axis has been reversed.
2.2.2 Processes controlling the mixed-layer salinity budget
Diagnostics implemented in CROCO make it possible to isolate the various terms involved in the salinity balance of the mixed layer to identify the dynamical processes that modify salinity. In CROCO, the temporal variation of the salinity, ∂tS, is expressed as follows for each layer of the water column:
with t being the time dimension; x, y, and z being the zonal, meridional, and vertical dimensions, respectively; u, v, and w being the current in the x, y, and z dimensions, respectively; S being the salinity; and Kz being the vertical diffusion coefficient.
The boundary conditions on salinity fluxes are as follows:
-
At the surface (z=0), .
-
At the ocean bottom , (no exchanges through the bottom).
Over the mixed layer of height h, the salinity budget is computed as follows:
Note that Sm is the depth-averaged salinity in the mixed layer: .
The left-hand side can be expressed as the sum of the time variation of Sm and the entrainment term:
The last term (related to vertical diffusion) is equal to
CROCO computes (for each time step) Sm and ∂tS online so that it can compute the entrainment term as a residual:
Thus, the final equation is
In this study, the mixing terms were found to be negligible compared to the other terms, and the horizontal and vertical advection terms were found to largely offset each other. Thus, in the following, the so-called advection term comprises the sum of the zonal, meridional, and vertical advection terms. The runoff forcing is introduced via the zonal advection term as rivers are introduced as westward zonal flows at different locations of the West African coastline.
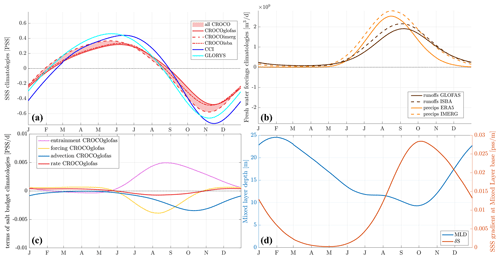
Figure 3(a) Seasonal cycle of SSS in the e-NTA region: CCI (blue), GLORYS (cyan), and various CROCO simulations. The red area represents the range between the minimum and maximum values of the simulated SSS. (b) ERA5 and IMERG precipitation (orange, in m3 d−1) and GloFAS and ISBA runoff (black, in m3 d−1) climatologies. (c) Trends of the salinity balance equation (in pss d−1). The yellow line represents the effect of the atmospheric forcing, the blue line represents the lumped advection term (horizontal and vertical, including runoff forcing), and the pink line represents the entrainment term at the base of the mixed layer. The red line is the SSS rate term, which corresponds approximately to the sum of the other three terms (vertical and horizontal diffusion are negligible). (d) Climatologies of the MLD (in m, blue) and the salinity gradient at the base of the mixed layer (in pss m−1, red) for the CROCOglofas simulation.
2.3 Comparison between SSS data sets and simulations
2.3.1 Analysis of cross-correlations
To study the relationship between the different variables involved in the salinity budget averaged over the e-NTA, we correlate forcing terms with salinities or other terms (such as mixed-layer depth (MLD)) using the determination coefficient (r2). To identify cause-and-effect relationships that can take weeks to establish, the correlation maximum that results from allowing a time delay (up to ± 90 d) between the variables is used.
2.3.2 Climatological and interannual variability of the salinity budget
The different variables linked to the salinity budget are spatially averaged over the e-NTA, and the resulting time series are analyzed from January 2010 to July 2019, the time period over which the ISBA runoff was available at the time of this study. The averaged seasonal and interannual signals are then extracted from the original signal as outlined below.
Seasonal signal. A two-stage method is used to calculate a climatological seasonal variation. A daily climatology is first calculated by averaging data available on each day of the year between 2010 and 2019. To eliminate short-term fluctuations, the daily climatology is then smoothed using a 1-month moving-average filter.
Interannual signal. To remove the seasonal variations, the monthly climatology is subtracted from the original daily time series. A 3-month moving average is then applied to filter intraseasonal variability in order to focus on interannual variability at seasonal timescales.
2.3.3 Colocation methodologies when comparing in situ and gridded data
When comparing gridded SSS values and Melax mooring or Argo float SSS values, a spatial bilinear interpolation of the gridded product and a selection of the nearest neighbors in time are used to collocate products represented on a grid with local data from Argo floats or the Melax buoy. When comparing gridded SSS and TSG SSS, we first perform a smoothing of in situ data with a Gaussian window along the ship track to a resolution comparable to gridded data sets (e.g., model or satellite data type), given the high spatio-temporal sampling of TSG measurements. The standard deviation of this filter is set to one-quarter of the spatial resolution of the model grid (95 % of the weight in a radius of half the spatial resolution). The resulting smoothed TSG data are compared to the nearest corresponding satellite or model pixel in time. Only the SSS values in pixels that are common to modeled and satellite SSS are considered when comparing results obtained with both data sets.
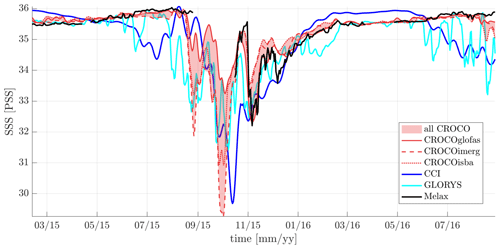
Figure 4Surface salinity between March 2015 and August 2016 at the Melax mooring (black line) from CROCO simulations (red lines; red shading shows the range between the maximum and minimum simulated values), from satellite CCI data (blue), and from GLORYS data (cyan). Notice that the nearest satellite data pixel is further than 30 km away from the mooring position and further than 50 km away from the coast.
3.1 Analysis and validation of the seasonal cycle of salinity
A study of the salinity balance resulting from the different CROCO simulations was carried out to analyze the dynamical processes at the origin of the SSS seasonal cycle (Fig. 3c). The SSS climatological variations are governed by the effect of precipitation during the summer rainy season. Rainfall accumulates on the continent during this period, generating intense river runoff 1–2 months later. The peak of river runoff is reached in September–October. This drives a continuous drop in salinity from the start of the rainy season onwards. These two effects also generate a strong vertical salinity gradient at the base of the mixed layer and a decrease in the MLD (Fig. 3d). The ocean transfers this freshwater input towards the ocean interior through vertical advection (not shown) and entrainment. During the dry season (January–May), the atmospheric forcing is slightly positive (due to evaporation being larger than precipitation) and is associated with a deeper mixed layer. There is also a strong vertical inflow of salt in the mixed layer by advection (not shown), which corresponds to the coastal upwelling that is particularly marked in March. Relatively salty upwelled waters are then redistributed by horizontal advection, notably by westward Ekman transport. This analysis is in line with that of Camara et al. (2015).
Although similar in shape and of the same order of magnitude, the seasonal cycles of the various SSS products present noticeable differences (Fig. 3a): CROCO SSS values have a seasonal cycle of smaller amplitude than those of CCI and GLORYS, and CCI SSS is in phase with CROCOglofas. On the other hand, CCI SSS lags behind CROCO SSS forced by ISBA runoff (CROCOimerg and CROCOisba) and behind GLORYS SSS by ∼ 2 weeks. Between the CROCO simulations, there is a difference of the order of 0.1 pss in amplitude, which may stem from a difference in the amplitude of the seasonal cycle of the precipitation products that were used, with the amplitude of the IMERG seasonal cycle being 3.108 m3 d−1 larger than the one of ERA5 (Fig. 3b).
3.2 Evaluation of the CROCO simulations using in situ measurements
3.2.1 Coastal SSS from the Melax mooring off southern Senegal
Salinity measurements at the Melax mooring provide a useful time series for the evaluation of the simulation near the Senegalese coast. During its first 2 years of deployment, the mooring recorded an almost continuous time series, with an SSS oscillation of ∼ 2–3 pss in amplitude in November 2015 (Fig. 4). CROCO SSS values agree well with in situ SSS during the dry seasons (March–September 2015 and March–July 2016) and at the time of the very strong oscillation in November. GLORYS also gives consistent results but underestimates the amplitude of the first observed oscillation of SSS (mid-October–early November 2015). GLORYS SSS is also highly oscillatory and too low over the periods when in situ SSS is stable (before September 2015 and after May 2016). The CCI SSS is further from in situ SSS, which is expected as the pixel collocated with the mooring is 30 km offshore of the mooring (and 55 km from coast) due to the application of the coastal flag and the land contamination close to the coast. In addition, in such a coastal area with unresolved satellite SSS variability, an uncertainty arises from the sampling difference between a pointwise in situ measurements and a satellite measurement integrated over ∼ 50km (Thouvenin-Masson, et al., 2022), which is greater than the GLORYS and CROCO horizontal resolutions.
The salinity balance is used to explain the origin of the strong oscillation detected in mid-2015 (see Fig. S5). Freshening is initiated in August 2015 by an event of intense precipitation and is amplified by advection of freshwater from the coastal regions south of the mooring, which collect a strong river runoff until November 2015. The observed oscillation in mid-November 2015 is caused by an oscillation of the zonal advection term, leading to an intensified westward (eastward) transport of relatively low-salinity (high-salinity) waters (not shown).
3.2.2 Argo and TSG
Although most of the in situ measurements are taken at a depth between 5 and 10 m, we have chosen to compare them with the salinity in the top layer of the model in order to be able to analyze these validations in the light of comparisons with satellite measurements taken in the first centimeter of the ocean (also note that there are no strong vertical salinity gradients in the top 5 m of the model water column).
Among the three types of gridded products, satellite observations show the closest alignment with in situ data, with r2 values of 0.94 and 0.89 when compared to Argo and TSG data, respectively (Table 2). The observed differences generally remain within 0.2 pss in absolute value (Fig. 5b, f), except for a few instances involving in situ measurements taken very close to the coast.
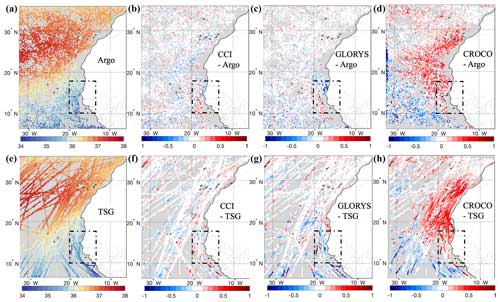
Figure 5(Top) Argo SSS (a) used as reference. Difference between Argo SSS and various SSS fields (CCI (b), GLORYS (c), and CROCOimerg (d)). (Bottom) TSG SSS (e) used as reference. Difference between TSG SSS and various SSS fields (CCI (f), GLORYS (g), and CROCOimerg (h)). Comparisons were averaged over 0.2° boxes to ease visualization.
Since the GLORYS reanalysis assimilates Argo data, the statistics of the comparisons with this data set are very good, as expected. The r2 values are 0.91 when comparing to Argo and 0.86 when comparing to TSG, which is only slightly lower than the values obtained with CCI (Table 2). However, there is a negative bias of −0.8 to −1 pss with respect to Argo and TSG measurements taken on the continental shelf at the mouth of the Senegal River (16° N). These Argo data were taken at the end of 2012, which corresponds to a period when river outflows were particularly high (Fig. 6c). It is therefore likely that these differences can be explained by the use of climatological runoff in GLORYS (Fig. 5c,g).
When CROCO SSS values are compared with in situ SSS, a significant bias of up to +0.5 pss is observed, which is fairly systematic near the coast and north of 14.7° N. This positive bias is observed with respect to both Argo and TSG data and with all CROCO simulations and thus seems to be robust. South of 14.7° N and far from the coast (30–20° W), a negative bias of the order of −0.2 pss is observed, while the few TSG data available on the continental shelf show a positive bias (Fig. 5d, h). There is a stronger bias in CROCOprclm (Table 2), suggesting that the climatological precipitation field strongly reduces the effect of rainfall on SSS. Comparison statistics between the other simulations and in situ data are very close (with maximum absolute differences in terms of r2 of the order of 0.01), with slightly better results for CROCOimerg.
The seasonal variability of the CROCO SSS bias with respect to CCI shows similar patterns (positive bias near the north Senegalese–Mauritanian coast), regardless of the year (not shown). Therefore, in the following, these simulations are analyzed on a relative basis after removing the annual mean bias of SSS. The figures shown below are based on fields from which the mean SSS has been removed. The origin of the systematic SSS bias in CROCO is discussed in Sect. 4.
The statistics calculated for the global and e-NTA regions are provided for reference in Table 2. There are significantly fewer co-located points in the e-NTA, lower dynamics of SSS, and a higher proportion of points close to the coast compared to the global region. Consequently, the statistics are consistently less favorable, with r2 values reaching 0.43 (0.67) compared to Argo floats (TSG).
Table 2Summary statistics of comparisons between various salinity products and in situ data over the full model domain and the e-NTA area (see maps in Fig. 5). “Std. diff.' stands for “standard difference”.
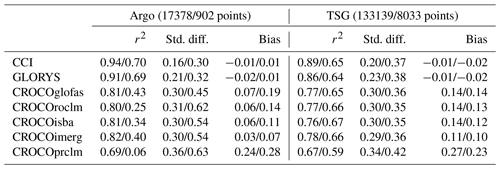
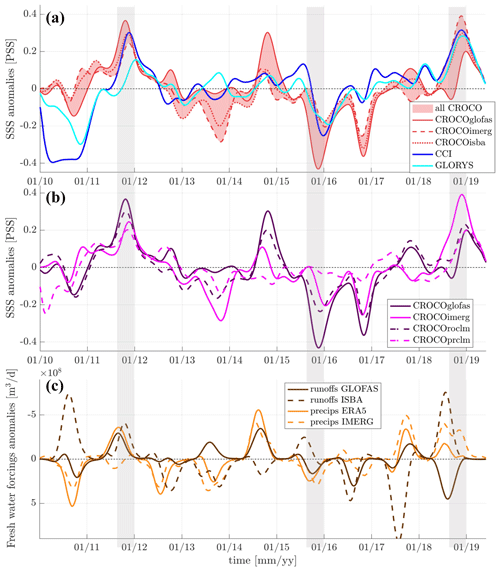
Figure 6Band-pass-filtered SSS anomalies for (a) CCI (blue), GLORYS (cyan), and the three interannual CROCO simulations (red; the red shading corresponds to the range between the minimum and maximum of the simulated SSS); (b) CROCO simulation with interannual runoff (solid purple line) and climatological runoff (dotted purple line) and CROCO simulation with interannual rain rate (solid magenta line) and climatological rain rate (dotted magenta line). Only pixels where CCI data are available have been considered in generating these curves. (c) Anomalies of the different forcings, runoff (brown, GloFAS plain line, ISBA dashed line) and precipitation (orange, ERA5 plain line, IMERG dashed line). The y axis has been reversed for easier comparison with SSS anomalies. Periods in gray shading correspond to large SSS anomalies, for which a detailed analysis is given below.
3.3 Origin of the main interannual variations
The interannual anomalies calculated for the different CROCO simulations are now compared to the interannual anomalies of the CCI and GLORYS products, used as references given their good agreement with in situ data (Table 2).
Interannual variations of SSS in the e-NTA are significant, oscillating between −0.4 and 0.4 pss and therefore being of the same order of magnitude as the seasonal cycle (Fig. 3). Overall, the different SSS estimates are in relatively good agreement. There is no long-term trend in the anomalies (Fig. 5): for each year, anomalies are close to zero during the first half of the year (late winter–summer) and reach their extrema at the end of the year (autumn–early winter), lagging behind the anomalies of rainfall and runoff by a few months (Fig. 6c). The interannual variability of SSS derived from the CROCO simulations (Fig. 6a) correctly represents the main variability compared to CCI and GLORYS. There are differences between CROCO simulations, which can reach 0.2–0.3 pss during the rainy season at the end of the year (e.g., 2014, 2015). For the rest of the year, the differences remain negligible. The strongest CROCO SSS anomalies are generally produced by CROCOglofas.
In 2011, 2015, and 2018, strong SSS anomalies were observed in the CCI and GLORYS products (see gray shading in Fig. 6) and were well represented by the CROCO simulations (Fig. 6a). The spatial distributions of SSS simulated by CROCOimerg and those of CCI SSS over the region for these 3 years are very similar during these time periods (Fig. 7) and are now studied in more detail.
There is a significant disparity in the anomalies of the two river discharge forcing products, with anomalies sometimes having opposite signs (Fig. 6c). This disparity is explored in the “Conclusions and discussion” section (Sect. 4.2).
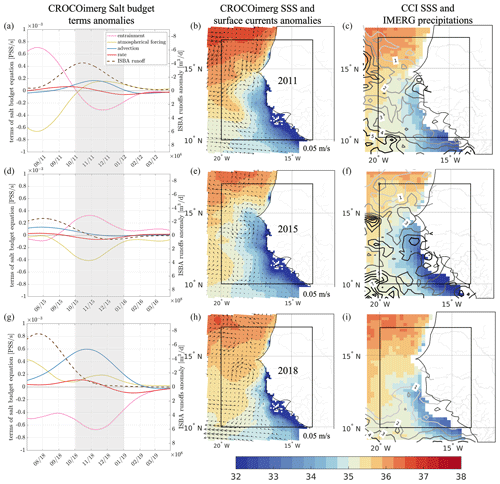
Figure 7SSS anomalies over the three analyzed periods: late 2011 (a, b, c), late 2015 (d, e, f), and late 2018 (g, h, i). Left column (a, d, g): anomalies of the terms in the salinity balance equation (in pss d−1) for CROCOimerg. The color code used is the same as that used in Fig. 3a. Only pixels where CCI data are available have been considered in generating these curves. The dotted black line is the ISBA runoff anomaly (the y axis has been reversed). The gray shading indicates time periods of strong salinity variations. Central column (b, e, h): simulated SSS maps (in pss) averaged over 3 months for CROCOimerg. Arrows show the surface current anomalies. Right column (c, f, i): CCI SSS maps (in pss) averaged over 3 months. Gray contours depict IMERG precipitation (in mm d−1; contour spacing is 1 mm d−1, and darker gray corresponds to higher precipitation).
3.3.1 Positive SSS anomaly in 2011
Around mid-2011, CROCO simulates a steep SSS increase and a positive anomaly, which is in good agreement with the CCI anomaly (Fig. 6a). The GLORYS anomaly displays the same variation, albeit with a lower magnitude. Although all CROCO simulations reproduce the SSS increase, the CROCOisba anomaly is the closest to the CCI anomaly.
The fact that the SSS increase also appears in the simulation with climatological precipitation (CROCOprclm) and the fact that the IMERG and ERA5 precipitation anomalies are of opposite signs (Fig. 6c) suggest that this anomaly does not result from a precipitation anomaly. Furthermore, the SSS anomaly is also present in the simulation with climatological runoff (CROCOroclm), and the changes in runoff forcing only generate second-order differences in the SSS anomalies (Fig. 6b), indicating that the runoff anomaly is not the primary cause of the salinity anomaly. Consequently, the SSS increase must arise mainly from the ocean circulation. The salinity balance (Fig. 6a) confirms that a positive anomaly in entrainment in summer (July–August), overcompensating for a negative anomaly in atmospheric forcing, triggers the SSS increase, which is reinforced by a positive anomaly in advection in autumn–early winter (September–January) in the case of CROCOimerg. A closer analysis of the advection anomaly indicates that this is mainly due to the anomaly of currents (V′<S>; see Fig. S6, blue curve) related to an increase in the southward wind-driven coastal current (coastal jet) through the climatological poleward gradient of salinity (Fig. 7b).
3.3.2 Negative SSS anomaly in 2015
Starting in mid-2015, most CROCO simulations show a significant freshening (from −0.4 pss for CROCOglofas to −0.2 pss for the other simulations), which is in good agreement with CCI and GLORYS (Fig. 6a). In contrast, simulations forced by climatological precipitation (CROCOprclm) display no anomaly, while simulations forced by climatological (CROCOroclm) and ISBA (CROCOimerg) runoff display a freshening that is 50 % weaker than with GloFAS runoff (CROCOglofas) (Fig. 6b). This suggests that this freshening is initially due to the precipitation anomaly, followed by the subsequent runoff anomaly (Fig. 6c). The analysis of the salinity balance equation confirms this hypothesis (Fig. 7d): the freshening is firstly due to the rain intensification (October–December 2015, yellow curve). It is then reinforced slightly by the runoff in December–January (blue curve) and by an anomalous salty-water outflow at the northern boundary of the e-NTA region (Fig. S6, blue curve). This anomalous advection of freshwater is amplified by the higher GloFAS runoff in CROCOglofas. This effect is discussed in more detail in Sect. 4.
3.3.3 Positive SSS anomaly in 2018
In mid-2018, the SSS anomalies reach ∼ 0.3 pss (Fig. 6a). As in 2011, all CROCO simulations, including those with climatological forcing (Fig. 6b), reproduce this positive anomaly, which cannot be attributed to one particular forcing anomaly. Analysis of the salinity balance for CROCOimerg (Fig. 7g) reveals that the salty anomaly initially results from a strong positive atmospheric forcing anomaly (i.e., rain deficit) in IMERG (Fig. 7i), also found in the ERA5 product (Fig. 6c). The greater impact of the IMERG precipitation anomaly on CROCOimerg SSS compared to the impact of the ERA5 precipitation anomaly on CROCOisba SSS (Fig. 6a) could be due to a more localized and more intense precipitation anomaly in IMERG than in ERA5 (see Fig. S1). This precipitation anomaly is accompanied by a large negative ISBA runoff anomaly (Figs. 6c, 7g, dashed black curve), increasing SSS by means of a very large positive advection (Fig. 7g, blue curve). This runoff anomaly explains why CROCOprclm (also forced by ISBA, Fig. 6b) also simulates the positive SSS anomaly (albeit more weakly than in CROCOimerg) without a precipitation anomaly. Because the GloFAS runoff anomaly has an opposite sign (i.e., larger runoff), the CROCOglofas simulation displays a weaker SSS anomaly than the simulations forced by ISBA runoff (CROCOimerg and CROCOisba; Fig. 6b). In the case of CROCOimerg (Fig. 7g), the anomaly is due to a negative anomaly in both rainfall and runoff (yellow and blue curves).
In conclusion, the 2018 SSS anomaly is due to the combined effects of precipitation anomalies and river discharges. It is primarily caused by a strong negative precipitation anomaly (observed in both forcing data sets), which is not entirely compensated for by entrainment (Fig. 7g). This is then accompanied by a negative (positive) river discharge anomaly of ISBA (GloFAS) runoff, thereby accentuating (mitigating) the salinity anomaly through advection. This runoff anomaly explains the CROCOprclm SSS anomaly. The large GloFAS runoff is surprising as it is opposite to the rain deficit over the oceanic region during this period (Fig. 6c).
3.4 SSS sensitivity to the freshwater forcings
In this section, we investigate more thoroughly the sensitivity of the simulated SSS to a modulation of runoff forcing, with all other model forcings being kept identical. We analyze the temporal variability of simulated salinity over the whole simulated period. Three test cases are set up using the simulations described in Table 1: the differences between simulations with a climatological runoff and a synoptic runoff (the GloFAS product) are first analyzed with regard to the interannual variability of forcing, and then the difference in SSS induced by the use of the ISBA or GloFAS synoptic runoff products is analyzed. Lastly, the effects of SSS modulation by precipitation changes are presented.
The panels in Fig. 8 depict these case studies. For each case, the difference in SSS between the studied simulations is shown in red, the differences in forcings in the e-NTA region are shown with solid blue lines, and those occurring south of the e-NTA region (5–10° N, 10–20° W; see Fig. 1) are shown with dashed lines. The region south of the e-NTA is indeed the site of strong freshwater influx, and the general oceanic circulation tends to advect these waters northward into the e-NTA region. The maximum of the cross-correlation function (r2) and the corresponding temporal lag are determined over the e-NTA region and the region south of the e-NTA, as explained in Sect. 2.3. See Fig. S4 for more information about the cross-correlations and the lags between forcing differences and their effect on salinity.
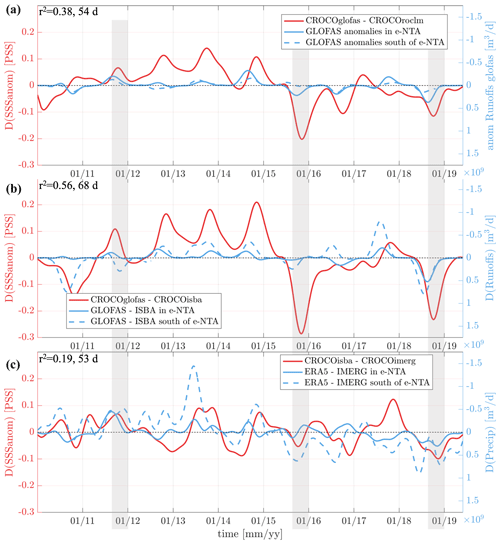
Figure 8(a) GloFAS runoff anomalies (blue) and difference between SSS anomalies (red) (CROCOglofas−CROCOroclm). (b) Differences between GloFAS and ISBA runoff anomalies (blue) and differences between SSS anomalies (red) (CROCOglofas−CROCOisba). Note that CROCOglofas, CROCOroclm, and CROCOisba are forced by the same ERA5 precipitation fields. In (a) and (b), blue lines show the sum of the runoff anomalies of rivers flowing directly into the e-NTA (local runoff, solid line) and south of the e-NTA (dashed line). (c) Differences between IMERG and ERA5 precipitation anomalies (blue line) in the e-NTA (solid line) and south of the e-NTA (dashed line) and differences between SSS anomalies in the e-NTA (red line) from simulations using IMERG (CROCOimerg) and ERA5 (CROCOisba) with the same ISBA runoff forcing. To ease the reading of the figure, the axes of precipitation and runoff anomalies have been reversed. The maximum correlation (r2) and time lag between the time series of SSS anomalies and of the sum of the freshwater flux in and south of the e-NTA region are indicated in the top left of the panels.
3.4.1 SSS sensitivity to interannual versus climatological runoff
The influence of GloFAS interannual runoff variability on salinity is investigated by comparing the CROCOroclm SSS to the CROCOglofas SSS. The other forcings (i.e., ERA5) are kept identical between the two simulations (Table 1) so that the differences observed in SSS are mainly the consequence of the difference in river outflow forcing and its effect on nearshore ocean dynamics. Note that SSS differences can also arise from differences in mesoscale circulation due to dynamical (chaotic) nonlinearities or intrinsic variability unrelated to the forcings.
Interannual runoff variability has a significant effect on SSS (Fig. 8a). The GloFAS runoff interannual variability is indeed correlated with the difference between CROCOglofas SSS and CROCOroclm SSS (r2 of 0.38 for a lag of 54 d; Fig. 8a). Differences in SSS may be due to both a local and a remote runoff anomaly (i.e., south of the e-NTA) (Fig. 8a, dotted line).
The salinity balance for CROCOglofas and CROCOroclm (not shown) indicates that, as for the climatological cycle (Fig. 3c), a difference in runoff is partly compensated for by a difference in entrainments of opposite sign. There is indeed a correlation of 0.98 between the interannual runoff anomaly and the entrainment term difference, with 75 % of the runoff anomalies being compensated for by entrainment on average. The lag time (54 d) between runoff anomalies and SSS difference is likely to be due to a localized (nearshore) effect of the runoff taking time to spread offshore and to modify the ocean surface layer over the entire e-NTA.
3.4.2 SSS sensitivity to a change in runoff interannual variability
The differences between ISBA and GloFAS runoff anomalies (Fig. 8b) are greater than the anomalies of either runoff measured independently as the anomalies are frequently of opposite sign (Fig. 6c). Consequently, the impact of the total runoff difference on salinity is larger than in the previous case study, with differences reaching 0.3 pss. An r2 of 0.56 is obtained with regard to the difference in runoff and the difference in SSS, with a time lag of 68 d; the difference in runoff is also offset by the entrainment at the bottom of the mixed layer: indeed, runoff difference and entrainment difference have a correlation of 0.98, and entrainment compensates for, on average, 84 % of the runoff difference, with a time lag of 13 d.
In these two case studies, the effect of runoff is variable: small deviations from the climatology can generate significant differences in SSS, as in 2015 (Fig. 8a, b), while large runoff anomalies sometimes have a limited effect, as in 2017 or 2018. These differences in behavior can be explained by surface current anomalies: in 2015, a northwesterly current transports the SSS anomaly linked to river flow so that it has a greater impact on the mean SSS of the e-NTA region (Fig. 7e). Thus, averaged SSS is particularly affected by a small change in runoff. Conversely, in 2018 (Fig. 8b), the large difference (1.2×109 m3 d−1) between the two runoff forcings with anomalies of opposite signs (Fig. 6c) coincided with a northerly wind anomaly (not shown). The SSS anomaly produced by runoff in 2018 is therefore confined to the coasts south of the e-NTA; it does not spread northward, and it has relatively little impact (0.22 pss) on the mean salinity of the area (Fig. 7h).
3.4.3 SSS sensitivity to a change in rain rate interannual variability
The effect of a change in precipitation on the simulated salinity – more specifically, on the difference induced by ERA5 (CROCOisba) and IMERG (CROCOimerg) synoptic precipitation products – is shown in Fig. 8c.
The differences between the IMERG and ERA5 mean precipitation fields are small in comparison to the amplitude of their climatologies, (Figs. 6c, 3b), but the aggregated freshwater fluxes over the e-NTA are of the same order as the differences between runoff forcings. The differences in SSS resulting from this difference in rain rate (Fig. 8c) are weaker than those associated with runoff differences and are not strongly driven by the direct effect of rain in contrast to the effect of river discharge (Sect. 3.4.2), as shown by the low correlation (r2=0.19). Note, however, that differences in SSS seem to be linked to differences in precipitation over certain periods (e.g., mid-2011, mid-2013, late 2014). As for runoff, the salinity balance of CROCOimerg shows that an anomaly in the rain rate forcing term is nearly totally compensated for by an anomaly in the entrainment term (Fig. 7a, d, g). This adjustment is almost exactly correlated with the rainfall difference (r2=0.99), and the entrainment difference compensates for, on average, 98 % of the forcing-term difference. The weaker correlation with precipitation anomalies than with runoff anomalies could be explained by the fact that precipitation anomalies are weaker locally but spread over a larger region than runoff. The influx of freshwater from rivers also occurs in coastal regions in the continental shelf region, where the mixed layer occupies the entire water column down to the bottom. This prevents the freshwater from being expelled by entrainment, forcing it to spread by advection, thus reducing the salinity of the mixing layer.
SSS values are simulated over the period 2010–2019 using an ocean circulation regional model (CROCO) off the West African region, forced by various precipitation and river runoff products. The simulated SSS values are compared to various local and global data sets: the CCI satellite product, the GLORYS reanalysis, the ARGO float database, a coastal mooring, and TSG measurements. The comparisons show that modeled SSS is systematically too high north of 15° N but is quite consistent with observations in terms of anomalies. Moreover, comparison with a coastal mooring 30 km off the coast of southern Senegal shows an excellent agreement. The simulation forced by IMERG precipitation and ISBA runoff is, on average, slightly closer to the observations than the other simulations over the period of study.
The simulated SSS values are analyzed in terms of seasonal cycle and interannual anomalies averaged over the eastern North Tropical Atlantic (e-NTA). At the first order, the amplitude and phase of the SSS seasonal cycle are modified only slightly by the different precipitation and runoff products used as model forcing. However, there is a time lag of about 2 weeks between the simulations, which corresponds to a shift in the seasonal cycles of the two runoff products. There is also a difference in amplitude of 0.1 pss, which may be due to a difference in precipitation forcing. The seasonal cycles of the CCI satellite data and the GLORYS reanalysis are also out of phase by about 2 weeks, which could originate from the climatological runoff used in the GLORYS reanalysis. Analysis of the modeled mixed-layer SSS budget indicates that the SSS decrease during the rainy season is driven, initially, by precipitation and, a few weeks later, by river runoff by means of horizontal advection of low-salinity coastal waters. These negative trends are partly (nearly fully) compensated for by runoff (precipitation) by entrainment of relatively saline subsurface water into the mixed layer. This can be explained as follows: when precipitation occurs, surface waters become less saline, and a vertical gradient of salinity is formed in the surface layer. As the mixed-layer depth deepens during the nighttime, saline subsurface water is incorporated (entrained) into the mixed layers, leading to an increased mixed-layer salinity. This diurnal salinization of the mixed layer occurs even when the mixed layer tends to decrease at a seasonal timescale (Fig. 3d). Thus, the larger the precipitation, the larger the salinity vertical gradient, and the larger the entrainment and compensation by salinization of the mixed layer.
Despite the systematic model bias, modeled and observed SSS interannual variations are, overall, in good agreement. Large SSS anomalies are often correlated with large precipitation or runoff anomalies within the e-NTA and from neighboring regions whose surface waters are then advected by surface currents into the e-NTA. However, a propagation of the river plume is not systematic and depends, in particular, on the wind-driven surface circulation patterns.
A study of the sensitivity of SSS to precipitation and runoff interannual variability shows a different response of the surface ocean to the two types of forcing. A difference in precipitation is almost totally compensated for by entrainment, while a difference in runoff is compensated for by between 75 % and 84 % on average. For a change in forcing of an equivalent order in terms of mean freshwater input, surface salinity is therefore more impacted by river runoff than by precipitation.
4.1 Uncertainties in SSS interannual variability
Over several time periods (e.g., 2010, 2013, 2014, and 2016; Fig. 6a), the CROCO SSS anomalies are markedly different from those of the reference products (GLORYS and CCI). In 2010, the CROCO anomalies appear to be underestimated compared with GLORYS and CCI. The latest shows a very strong freshening (∼ −0.4 pss) during most of the year (Fig. 6a), which is likely to be exaggerated. In 2010, the CCI data set relies only on the SMOS data set, the absolute calibration of which in 2010 is questionable (Boutin et al., 2021a). The GLORYS product also shows a freshening (−0.15 pss), but this is weaker than in CCI. The CROCOglofas simulation is the closest in 2010 to GLORYS. In 2013, there is a large disparity in SSS values (up to 0.3 pss) between the various CROCO simulations. The CROCOglofas SSS displays a very weak anomaly, similarly to CCI, while all the other CROCO simulations show a moderate freshening (−0.15 to −0.28 pss). These discrepancies could be explained by the relatively large difference in runoff during this period (Fig. 6c). CROCOglofas is less biased than CROCOisba with respect to CCI and GLORYS, suggesting that the anomalously low runoff of GloFAS in 2013 is more realistic. In contrast, in 2014, CROCOglofas shows an unrealistic, high positive SSS anomaly not found in the other simulations. This strong anomaly is associated with an anomalously low GloFAS runoff in contrast to the anomalously high runoff of ISBA driving the more realistic SSS anomaly in CROCOisba. In 2016, the CROCO simulations are in relatively good agreement with one another but display a much stronger freshening than what is found in both reference products (Fig. 6a). Only CROCOprclm simulates the moderate freshening correctly (Fig. 6b; see dotted magenta line). Analysis of the salinity balance shows that advection drives the overly strong freshening (not shown), which may result from unrealistic surface currents in this period. Intrinsic variability may also play a role as all simulations, including CROCOprclm, are forced by the same ERA5 winds.
4.2 Uncertainties in freshwater forcings
4.2.1 Disparity between river discharge forcing interannual variability
This study highlights the disparity between two river discharge products available for West Africa. The GloFAS and the ISBA products are rather consistent in terms of seasonal cycle amplitude, with a maximum difference between seasonal cycles of 3×108 m3 d−1 (Fig. 3b), but the phasing of the cycle is shifted by about 2 weeks, and interannual anomalies are frequently of opposite signs (Fig. 6c). This is in line with results from Decharme et al. (2019) showing that runoff values simulated by different hydrological models driven by various precipitation products have a significant disparity in terms of the intensities and phases of seasonal runoff cycles (e.g., see Fig. 14 of Decharme et al., 2019).
To understand the origin of these disparities, the runoff anomalies of each product are compared with the rainfall anomalies over the catchment areas of the rivers flowing through the study region (see Fig. 1 for catchment delimitation). The runoff anomalies of each product are strongly correlated with the rainfall anomalies used in the hydrological models (Fig. 2): GloFAS runoff anomalies are correlated with the ERA5 rainfall anomalies over the catchment area with an r2 of 0.87 and with a time lag of 22 d, and ISBA runoff anomalies are correlated with the IMERG rainfall anomalies over the catchment area with an r2 of 0.56 and with a time lag of 33 d. This suggests that the quality of runoff estimation is highly dependent on the quality of the estimation of rainfall on land. Comparisons of modeled SSS with in situ SSS over the entire period (2010–2019) show slightly better results in simulations forced by ISBA but using the GloFAS (respectively, ISBA) product leads to more accurate modeled SSS at the beginning (respectively, end) of the studied period. In conclusion, the present study presents an indirect evaluation of runoff interannual variabilities by analyzing their impact on SSS.
4.2.2 Impact of a monthly climatological precipitation forcing
The CROCOprclm simulation was designed to suppress the effect of interannual precipitation variability on salinity. However, the calculation of a monthly climatological precipitation field by averaging monthly precipitation rates from various years drastically changes the distribution of precipitation (Figs. S2 and S3) by smoothing and attenuating the highly localized precipitation phenomena. Climatological values do not exceed m d−1, which is too low for synoptic fields. So, rather than highlighting the effect of temporal precipitation anomalies, this simulation highlights the effect of a reduction of spatial rainfall variability. To reproduce a commonly observed forcing, it would be better to design a daily climatological field consisting of a succession of typical years with a realistic precipitation distribution but scaled to a climatology in terms of total precipitation quantities. Such experiments are planned for future studies.
4.3 CROCO SSS biases
As seen in Sect. 3.2.2, the CROCO SSS values in all simulations were too high with respect to observations, mainly north of Cape Verde (15° N). This SSS bias is, in fact, associated with a positive temperature bias of ∼ 0.5–1.5 °C (Fig. S7 and text below). Such an SST bias is estimated to lead to an excess of evaporation that could explain about one-third of the SSS bias (see histograms in Fig. S8). The remaining SSS biases could be due to a salinity bias in the subsurface waters transported to the surface layer by coastal upwelling and then offshore by Ekman currents.
Other processes neglected or misrepresented in the regional model may impact the SSS bias. First, the salinity of the river inflows (i.e., the runoff salinity), set to 15 pss in our study, may have an impact. This choice is debatable as it is expected that the salinity from rivers would be closer to 0 pss. For example, salinity gradually increases from ∼ 0 pss at ∼ 7 km from the coast to 10 pss at the estuary mouth of the Suwannee River in western Florida (Laurel-Castillo and Valle-Levinson, 2023).
Most West African river mouths have the particularity of being located near very flat coasts, which promotes the formation of large estuaries despite their relatively low runoff (Descroix et al., 2020). These large estuaries allow the intrusion of seawater inland and facilitate water evaporation. Salinity in the Senegal River reaches a minimum of 10 pss in October, at the peak of the flow, and 35 pss in winter (Mikhailov and Isupova, 2008). The Sine-Saloum and Casamance rivers even have inverse estuaries, with estuarine salinity higher than that of the ocean (Pagès and Citeau, 1990; Descroix et al., 2020).
The value of 15 pss was chosen considering mixing between river waters and seawater, as well as evaporation inside the estuary. However, the effect of a change in CROCO runoff salinity can impact SSS in the freshwater plume: a sensitivity study shows that setting the runoff salinity to 1 pss instead of 15 pss can lead to a decrease in SSS of about 1 pss in regions traversed by the plume (Fig. S9). Future studies should consider the seasonal variability of runoff salinity when data are available. It should be noted that this effect on SSS as a result of a reduction in runoff salinity has little impact offshore of the Senegal River (Fig. S9) and thus does not explain the positive SSS bias north of 15° N (Fig. 5).
Lastly, tidal effects were not considered in our simulations, mainly to reduce computing time (a short model time step is required in the presence of strong tidal currents). However, it has been shown in the Amazon plume region that tides can impact plume propagation (Ruault et al., 2020) and, conversely, that river flows can enhance tidal elevation (Durand et al., 2022). A sensitivity study of SSS in relation to tides shows that tides can, in some cases, cause an increase in average SSS in the e-NTA region of about 1 pss due to increased vertical mixing (Fig. S10). Thus, including this effect would not reduce the positive bias north of 15° N, and the impact on our results is likely to be weak. However, a more detailed evaluation of the tidal effect on river plume propagation in our region of interest would be needed to confirm these results.
TSG data are available at https://doi.org/10.6096/SSS-LEGOS (Delcroix et al., 2002), and Argo data have been downloaded from the Pi-MEP database at https://pimep.ifremer.fr/diffusion/data/cci-l4-esa-merged-oi-v3.2-7dr/argo/ (Guimbard et al., 2021). Melax data are available at https://doi.org/10.5281/zenodo.4095436 (Lazar et al., 2020). CCI data are available from the Centre for Environmental Data Analysis (CEDA) (https://doi.org/10.5285/5920a2c77e3c45339477acd31ce62c3c, Boutin et al., 2021b). GLORYS reanalysis data are available at https://doi.org/10.48670/moi-00021 (EU Copernicus Marine Service Product, 2023), and the ERA5 data set provided by the ECMWF is available at https://doi.org/10.24381/cds.143582cf (Hersbach et al., 2017). CROCO model information is available at https://www.CROCO-ocean.org/ (Hilt et al., 2020). Given the large size of the modeling experiment outputs (∼ 1.6 TB for each simulation), the data set is not stored online and can be shared upon request to the corresponding authors. The ISBA-CTRIP data are available from the authors (Decharme et al., 2019). IMERG data are available at https://doi.org/10.5067/GPM/IMERGDF/DAY/07 (Huffman et al., 2023), and GloFAS data can be downloaded through the ECMWF Meteorological Archival and Retrieval System (MARS) https://confluence.ecmwf.int/display/CEMS/MARS (Harrigan et al., 2020).
The supplement related to this article is available online at: https://doi.org/10.5194/os-20-1547-2024-supplement.
CTM conducted the study and wrote the initial version of the paper. CTM, VE, and JB designed the simulations. CTM prepared the forcing fields. VE performed the simulations, and CTM analyzed them. CTM, JB, and JLV performed the satellite data processing and analysis. AL collected the Melax in situ data. CTM, JB, VE, and AL participated in the discussion of the results. All the authors have read, improved upon, and agreed with the content of the paper.
The contact author has declared that none of the authors has any competing interests.
Publisher’s note: Copernicus Publications remains neutral with regard to jurisdictional claims made in the text, published maps, institutional affiliations, or any other geographical representation in this paper. While Copernicus Publications makes every effort to include appropriate place names, the final responsibility lies with the authors.
This work is part of the PhD of Clovis Thouvenin-Masson and of the ESA CCI+SSS project. CROCO modeling experiments were conducted on the IDRIS high-performance computing facility Jean Zay. GLORYS global ocean model outputs were provided by the Copernicus Marine Environment Monitoring Service (CMEMS). We thank Xavier Perrot for the discussions about rain rate products; Manon Gevaudan, Julien Jouanno, and Sylvain Biancamaria for providing the precious information about ISBA-CTRIP; and Patrick Marchesiello for the insightful discussions about the CROCO model.
This research has been supported by the Centre national d’études spatiales (grant no. TOSCA SMOS-OCEAN), CNES and ACRI-st (PhD allocation no. 3233), the European Space Agency (grant no. 4000123663/18/I-NB), and DARI project nos. A0110101140 and A0130101140.
This paper was edited by Aida Alvera-Azcárate and reviewed by Sreelekha Jarugula and two anonymous referees.
Akinsanola, A. A., Zhou, W., Zhou, T., and Keenlyside, N.: Amplification of synoptic to annual variability of West African summer monsoon rainfall under global warming, npj Clim. Atmos. Sci., 3, 21, https://doi.org/10.1038/s41612-020-0125-1, 2020.
Alory, G., Delcroix, T., Téchiné, P., Diverrès, D., Varillon, D., Cravatte, S., Gouriou, Y., Grelet, J., Jacquin, S., Kestenare, E., Maes, C., Morrow, R., Perrier, J., Reverdin, G., and Roubaud, F.: The French contribution to the voluntary observing ships network of sea surface salinity, Deep-Sea Res. Pt. I, 105, 1–18, https://doi.org/10.1016/j.dsr.2015.08.005, 2015.
Ardoin-Bardin, S., Dezetter, A., Servat, E., Paturel, J.-E., Mahe, G., Niel, H., and Dieulin, C.: Using general circulation model outputs to assess impacts of climate change on runoff for large hydrological catchments in West Africa, Hydrolog. Sci. J., 54, 77–89, https://doi.org/10.1623/hysj.54.1.77, 2009.
Argo: Argo float data and metadata from Global Data Assembly Centre (Argo GDAC), https://doi.org/10.17882/42182, 2023.
Beck, H. E., Van Dijk, A. I. J. M., Levizzani, V., Schellekens, J., Miralles, D. G., Martens, B., and De Roo, A.: MSWEP: 3-hourly 0.25° global gridded precipitation (1979–2015) by merging gauge, satellite, and reanalysis data, Hydrol. Earth Syst. Sci., 21, 589–615, https://doi.org/10.5194/hess-21-589-2017, 2017.
Boutin, J., Reul, N., Koehler, J., Martin, A., Catany, R., Guimbard, S., Rouffi, F., Vergely, J.-L., Arias, M., Chakroun, M., Corato, G., Estella-Perez, V., Hasson, A., Josey, S., Khvorostyanov, D., Kolodziejczyk, N., Mignot, J., Olivier, L., Reverdin, G., Stammer, D., Supply, A., Thouvenin-Masson, C., Turiel, A., Vialard, J., Cipollini, P., Donlon, C., Sabia, R., and Mecklenburg, S.: Satellite-Based Sea Surface Salinity Designed for Ocean and Climate Studies, J. Geophys. Res.-Ocean., 126, e2021JC017676, https://doi.org/10.1029/2021JC017676, 2021a.
Boutin, J., Vergely, J.-L., Reul, N., Catany, R., Koehler, J., Martin, A., Rouffi, F., Arias, M., Chakroun, M., Corato, G., Estella-Perez, V., Guimbard, S., Hasson, A., Josey, S., Khvorostyanov, D., Kolodziejczyk, N., Mignot, J., Olivier, L., Reverdin, G., Stammer, D., Supply, A., Thouvenin-Masson, C., Turiel, A., Vialard, J., Cipollini, P., and Donlon, C.: ESA Sea Surface Salinity Climate Change Initiative (Sea_Surface_Salinity_cci): weekly and monthly sea surface salinity products, v03.21, for 2010 to 2020, NERC EDS Centre for Environmental Data Analysis [data set], https://doi.org/10.5285/5920a2c77e3c45339477acd31ce62c3c, 2021b.
Camara, I., Kolodziejczyk, N., Mignot, J., Lazar, A., and Gaye, A. T.: On the seasonal variations of salinity of the tropical Atlantic mixed layer, J. Geophys. Res.-Ocean., 120, 4441–4462, https://doi.org/10.1002/2015JC010865, 2015.
Chandanpurkar, H. A., Lee, T., Wang, X., Zhang, H., Fournier, S., Fenty, I., Fukumori, I., Menemenlis, D., Piecuch, C. G., Reager, J. T., Wang, O., and Worden, J.: Influence of Nonseasonal River Discharge on Sea Surface Salinity and Height, J. Adv. Model. Earth Syst., 14, e2021MS002715, https://doi.org/10.1029/2021MS002715, 2022.
Chandanpurkar, H. A., Reager, J. T., Famiglietti, J. S., and Syed, T. H.: Satellite-and reanalysis-based mass balance estimates of global continental discharge (1993–2015), J. Clim., 30, 8481–8495, https://doi.org/10.1175/JCLI-D-16-0708.1, 2017.
Dai, A., Qian, T., Trenberth, K. E., and Milliman, J. D.: Changes in Continental Freshwater Discharge from 1948 to 2004, J. Clim., 22, 2773–2792, https://doi.org/10.1175/2008JCLI2592.1, 2009.
de Rosnay, P., Balsamo, G., Albergel, C., Muñoz-Sabater, J., and Isaksen, L.: Initialisation of Land Surface Variables for Numerical Weather Prediction, Surv. Geophys., 35, 607–621, https://doi.org/10.1007/s10712-012-9207-x, 2012.
Debreu, L., Vouland, C., and Blayo, E.: AGRIF: Adaptive grid refinement in Fortran, Comput. Geosci., 34, 8–13, https://doi.org/10.1016/j.cageo.2007.01.009, 2008.
Decharme, B., Delire, C., Minvielle, M., Colin, J., Vergnes, J. P., Alias, A., Saint-Martin, D., Séférian, R., Sénési, S., and Voldoire, A.: Recent Changes in the ISBA-CTRIP Land Surface System for Use in the CNRM-CM6 Climate Model and in Global Off-Line Hydrological Applications, J. Adv. Model. Earth Syst., 11, 1207–1252, https://doi.org/10.1029/2018MS001545, 2019.
Delcroix, T., Alory, G., Téchiné, P., Diverrès, D., Varillon, D., Cravatte, S., Gouriou, Y., Grelet, J., Jacquin, S., Kestenare, E., Maes, C., Morrow, R., Perrier, J., Reverdin, G., and Roubaud, F.: Sea Surface Salinity data from Voluntary Observing Ships Network, Odatis [dataset], https://doi.org/10.6096/SSS-LEGOS, 2002.
Descroix, L., Sané, Y., Thior, M., Manga, S.-P., Ba, B. D., Mingou, J., Mendy, V., Coly, S., Dièye, A., Badiane, A., Senghor, M.-J., Diedhiou, A.-B., Sow, D., Bouaita, Y., Soumaré, S., Diop, A., Faty, B., Sow, B. A., Machu, E., Montoroi, J.-P., Andrieu, J., and Vandervaere, J.-P.: Inverse Estuaries in West Africa: Evidence of the Rainfall Recovery?, Water, 12, 647, https://doi.org/10.3390/w12030647, 2020.
Durand, F., Piecuch, C. G., Becker, M., Papa, F., Raju, S. V., Khan, J. U., and Ponte, R. M.: Impact of Continental Freshwater Runoff on Coastal Sea Level, Surv. Geophys., 40, 1437–1466, https://doi.org/10.1007/s10712-019-09536-w, 2019.
Durand, F., Testut, L., Jouanno, J., and Fassoni-Andrade, A. C.: Role of the amazon outflow on the barotropic tide on the amazonian shelf, Cont. Shelf Res., 238, 104695, https://doi.org/10.1016/j.csr.2022.104695, 2022.
EU Copernicus Marine Service Product: Global Ocean Physics Reanalysis, Mercator Ocean International [data set], https://doi.org/10.48670/moi-00021, 2023.
Failler, P., El Ayoubi, H., and Konan, A.: Industrie des pêches et de l'aquaculture en Côte d'Ivoire, https://doi.org/10.13140/RG.2.1.2919, 2014.
Fournier, S., Reager, J. T., Chandanpurkar, H. A., Pascolini-Campbell, M., and Jarugula, S.: The Salinity of Coastal Waters as a Bellwether for Global Water Cycle Changes, Geophys. Res. Lett., 50, e2023GL106684, https://doi.org/10.1029/2023GL106684, 2023.
Gévaudan, M., Durand, F., and Jouanno, J.: Influence of the Amazon-Orinoco Discharge Interannual Variability on the Western Tropical Atlantic Salinity and Temperature, J. Geophys. Res.-Ocean., 127, e2022JC018495, https://doi.org/10.1029/2022JC018495, 2022.
Guimbard, S., Reul, N., Sabia, R., Herlédan, S., Khoury Hanna, Z. E., Piollé, J.-F., Paul, F., Lee, T., Schanze, J. J., Bingham, F. M., Le Vine, D., Vinogradova-Shiffer, N., Mecklenburg, S., Scipal, K., and Laur, H.: The Salinity Pilot-Mission Exploitation Platform (Pi-MEP): A Hub for Validation and Exploitation of Satellite Sea Surface Salinity Data, Remote Sens., 13, 4600, https://doi.org/10.3390/rs13224600, 2021 (data available at: https://pimep.ifremer.fr/diffusion/data/cci-l4-esa-merged-oi-v3.2-7dr/argo/, last access: 26 November 2024).
Harrigan, S., Zsoter, E., Alfieri, L., Prudhomme, C., Salamon, P., Wetterhall, F., Barnard, C., Cloke, H., and Pappenberger, F.: GloFAS-ERA5 operational global river discharge reanalysis 1979–present, Earth Syst. Sci. Data, 12, 2043–2060, https://doi.org/10.5194/essd-12-2043-2020, 2020 (data available at: https://confluence.ecmwf.int/display/CEMS/MARS, last access: 26 November 2024).
Hersbach, H., Bell, B., Berrisford, P., Hirahara, S., Horányi, A., Muñoz-Sabater, J., Nicolas, J., Peubey, C., Radu, R., Schepers, D., Simmons, A., Soci, C., Abdalla, S., Abellan, X., Balsamo, G., Bechtold, P., Biavati, G., Bidlot, J., Bonavita, M., De Chiara, G., Dahlgren, P., Dee, D., Diamantakis, M., Dragani, R., Flemming, J., Forbes, R., Fuentes, M., Geer, A., Haimberger, L., Healy, S., Hogan, R. J., Hólm, E., Janisková, M., Keeley, S., Laloyaux, P., Lopez, P., Lupu, C., Radnoti, G., de Rosnay, P., Rozum, I., Vamborg, F., Villaume, S., and Thépaut, J.-N.: Complete ERA5 from 1940: Fifth generation of ECMWF atmospheric reanalyses of the global climate, Copernicus Climate Change Service (C3S) Data Store (CDS) [data set], https://doi.org/10.24381/cds.143582cf, 2017.
Hersbach, H., Bell, B., Berrisford, P., Hirahara, S., Horányi, A., Muñoz-Sabater, J., Nicolas, J., Peubey, C., Radu, R., and Schepers, D.: The ERA5 global reanalysis, Q. J. R. Meteorol. Soc., 146, 1999–2049, https://doi.org/10.1002/qj.3803, 2020.
Hilt, M., Auclair, F., Benshila, R., Bordois, L., Capet, X., Debreu, L., Dumas, F., Jullien, S., Lemarié, F., Marchesiello, P., Nguyen, C., and Roblou, L.: Numerical modelling of hydraulic control, solitary waves and primary instabilities in the Strait of Gibraltar, Ocean Model., 151, 101642, https://doi.org/10.1016/j.ocemod.2020.101642, 2020.
Huffman, G. J., Stocker, E. F., Bolvin, D. T., Nelkin, E. J., and Tan, J.: GPM IMERG Final Precipitation L3 1 day 0.1 degree x 0.1 degree V07, edited by: Savtchenko, A., Greenbelt, MD, Goddard Earth Sciences Data and Information Services Center (GES DISC) [data set], https://doi.org/10.5067/GPM/IMERGDF/DAY/07, 2023.
Joyce, R. J., Janowiak, J. E., Arkin, P. A., and Xie, P.: CMORPH: A method that produces global precipitation estimates from passive microwave and infrared data at high spatial and temporal resolution, J. Hydrometeorol., 5, 487–503, https://doi.org/10.1175/1525-7541(2004)005<0487:CAMTPG>2.0.CO;2, 2004.
Laurel-Castillo, J. A., and Valle-Levinson, A.: A Statistics-Based Analytical Framework for Intratidal and Tidally Averaged Salinity Distribution in Estuaries, J. Geophys. Res.-Ocean., 128, e2023JC019813, https://doi.org/10.1029/2023JC019813, 2023.
Lazar, A., Machu, E., Tall A. W., Bodichon, R., Capet, X., Échevin, V., Pietri, A., and Corréa, K.: Supporting datasets used in the Geophysical Research – Oceans entitled “Variability of dissolved oxygen in the bottom layer of the southern Senegalese shelf”, Zenodo [data set], https://doi.org/10.5281/zenodo.4095436, 2020.
Lellouche, J.-M., Greiner, E., Le Galloudec, O., Garric, G., Regnier, C., Drevillon, M., Benkiran, M., Testut, C.-E., Bourdalle-Badie, R., Gasparin, F., Hernandez, O., Levier, B., Drillet, Y., Remy, E., and Le Traon, P.-Y.: Recent updates to the Copernicus Marine Service global ocean monitoring and forecasting real-time ° high-resolution system, Ocean Sci., 14, 1093–1126, https://doi.org/10.5194/os-14-1093-2018, 2018.
Lellouche, J.-M., Greiner, E., Bourdalle-Badie, R., Garric, G., Regnier, C., Drevillon, M., Benkiran, M., Hernandez, O., and Le Traon, P.-Y.: The Copernicus Global ° Oceanic and Sea Ice GLORYS12 Reanalysis, Front. Earth Sci., 9, 698876, https://doi.org/10.3389/feart.2021.698876, 2021.
Mignot, J., de Boyer Montégut, C., Lazar, A., and Cravatte, S.: Control of salinity on the mixed layer depth in the world ocean: 2. Tropical areas, J. Geophys. Res.-Ocean., 112, C10010, https://doi.org/10.1029/2006JC003954, 2007.
Mikhailov, V. N., and Isupova, M. V.: Hypersalinization of river estuaries in West Africa, Water Resour., 35, 367–385, https://doi.org/10.1134/S0097807808040015, 2008.
Ndoye, S., Capet, X., Estrade, P., Machu, E., Kounta, L., Sow, B., Diakhaté, M., and Gaye, A. T.: A numerical modeling study of the Southern Senegal upwelling shelf: Circulation and upwelling source waters, Afr. J. Environ. Sci. Technol., 12, 487–500, https://doi.org/10.5897/AJEST2018.2572, 2018.
Pagès, J., and Citeau, J.: Rainfall and salinity of a Sahelian estuary between 1927 and 1987, J. Hydrol., 113, 325–341, https://doi.org/10.1016/0022-1694(90)90182-W, 1990.
Roudier, P., Ducharne, A., and Feyen, L.: Climate change impacts on runoff in West Africa: A review, Hydrol. Earth Syst. Sci., 18, 2789–2801, https://doi.org/10.5194/hess-18-2789-2014, 2014.
Ruault, V., Jouanno, J., Durand, F., Chanut, J., and Benshila, R.: Role of the Tide on the Structure of the Amazon Plume: A Numerical Modeling Approach, J. Geophys. Res.-Ocean., 125, e2019JC015841, https://doi.org/10.1029/2019JC015495, 2020.
Shchepetkin, A. F. and McWilliams, J. C.: Correction and commentary for “Ocean forecasting in terrain-following coordinates: Formulation and skill assessment of the regional ocean modeling system” by Haidvogel et al., J. Comp. Phys., 228, 8985–9000, https://doi.org/10.1016/j.jcp.2009.09.002, 2009.
Supply, A., Boutin, J., Reverdin, G., Vergely, J.-L., and Bellenger, H.: Variability of Satellite Sea Surface Salinity Under Rainfall, in: Satellite Precipitation Measurement, Vol. 2, edited by: Levizzani, V., Kidd, C., Kirschbaum, D., Kummerow, C., Nakamura, K., and Turk, F., Springer Int. Publ., Cham, 1155–1176, https://doi.org/10.1007/978-3-030-35798-6_34, 2020.
Tall, A. W., Machu, E., Echevin, V., Capet, X., Pietri, A., Corréa, K., Sall, S. M., and Lazar, A.: Variability of Dissolved Oxygen in the Bottom Layer of the Southern Senegalese Shelf, J. Geophys. Res.-Ocean., 126, e2020JC016633, https://doi.org/10.1029/2020JC016854, 2021.
Thouvenin-Masson, C., Boutin, J., Vergely, J.-L., Reverdin, G., Martin, A. C., Guimbard, S., Reul, N., Sabia, R., Catany, R., and Fanton-d'Andon, O. H.: Satellite and in situ sampling mismatches: Consequences for the estimation of satellite sea surface salinity uncertainties, Remote Sens., 14, 1878, https://doi.org/10.3390/rs14081878, 2022.
Vialard, J. and Delecluse, P.: An OGCM Study for the TOGA Decade. Part I: Role of the Salinity in the Physics of the Western Pacific Fresh Pool, J. Phys. Oceanogr., 28, 1071–1088, https://doi.org/10.1175/1520-0485(1998)028<1071:AOSFTT>2.0.CO;2, 1998.
Wohl, E., and Lininger, K. B.: Hydrology and discharge, in: Large Rivers: Geomorphology and Management, 2nd edn., edited by: Gupta, A., Wiley, Hoboken, 42–75, https://doi.org/10.1002/9781119412632.ch3, 2022.
Zine, S., Boutin, J., Font, J., Reul, N., Waldteufel, P., Gabarro, C., Tenerelli, J., Petitcolin, F., Vergely, J.-L., Talone, M., and Delwart, S.: Overview of the SMOS Sea Surface Salinity Prototype Processor, IEEE Trans. Geosci. Remote Sens., 46, 621–645, https://doi.org/10.1109/TGRS.2008.915543, 2008.