the Creative Commons Attribution 4.0 License.
the Creative Commons Attribution 4.0 License.
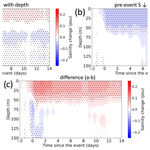
Upper-ocean changes with hurricane-strength wind events: a study using Argo profiles and an ocean reanalysis
Donata Giglio
Addison Hu
Mikael Kuusela
Kimberly M. Wood
Ann B. Lee
As the Earth's climate warms, the intensity and rain rate of tropical cyclones (TCs) is projected to increase. TCs intensify by extracting heat energy from the ocean; hence, a better understanding of upper-ocean changes with the TC passage is helpful to improve our understanding of air–sea interactions during and after the event. This work uses Argo float observations and the HYbrid Coordinate Ocean Model (HYCOM) ocean reanalysis to describe characteristics of upper-ocean changes with hurricane-strength wind events. We study the association of these changes with the vertical structure of the salinity profile before the event, i.e., increasing versus decreasing with depth. We also study the contribution of changes in salinity to upper-ocean density changes in each case. Results show that in regions where pre-event salinity increases with depth there is a corresponding statistically significant increase in upper-ocean salinity; vice versa, we observe a significant decrease in upper-ocean salinity in regions where pre-event salinity decreases with depth. Consistent with previous studies, temperature decreases in both regions. As near-surface temperature decreases, upper-ocean density increases, and the increase is larger where pre-event salinity increases with depth. Changes in upper-ocean properties from Argo and HYCOM are overall consistent with wind-driven vertical mixing of near-surface waters with colder and higher-salinity (or lower-salinity) waters below. Resulting changes in ocean stratification have implications for air–sea interactions during and after the event, with potential impacts on weather events that follow.
- Article
(7829 KB) - Full-text XML
-
Supplement
(9657 KB) - BibTeX
- EndNote
Tropical cyclones (TCs) represent a severe threat for both the human population and the fragile ecosystems that live in the tropical ocean (Greening et al., 2006; Orr and Ogden, 1992), causing thousands of fatalities and billions of US dollars in damage globally each year (Emanuel, 2003; Klotzbach et al., 2018; Mendelsohn et al., 2012).
The number of hurricanes decreased during 1990–2021, likely due to a more La Niña-like base state favoring TC activity in the North Atlantic and suppressing it in the Pacific (Klotzbach et al., 2022); yet TC intensification rates and the frequency of rapid TC intensification have increased in the last few years (Balaguru et al., 2018; Bhatia et al., 2019; Kishtawal et al., 2012). As TCs are becoming more powerful (IPCC, 2022), it is important to improve our understanding of the physical mechanisms that may regulate air–sea interactions during and after the TC passage (Cione et al., 2013), e.g., to improve the prediction of TC intensification and mitigate the TC-related destructive potential. TCs intensify by extracting energy from the ocean in the form of latent heat (e.g., Chan, 2005; Emanuel, 1999), leading to a substantial ocean cooling (Shen and Ginis, 2003; Han, 2023). Hence, the underlying sea surface temperatures (SSTs) are a key factor in determining how TCs evolve in time. Also, the strong winds of tropical cyclones induce upper-ocean mixing of cold water from deeper in the ocean with the warm surface waters – a process called upwelling (Webb and Suginohara, 2001; Prasad and Hogan, 2007; Han et al., 2024). This mixing entrains colder water from below the mixed layer and decreases SST (Cui et al., 2023; Elsberry et al., 1976; Fisher, 1958; Karnauskas et al., 2021; Leipper, 1967; Price, 1981), resulting in a negative feedback on the cyclone's intensity via the impact of SST cooling on air–sea enthalpy fluxes (e.g., Balaguru et al., 2012; Bender and Ginis, 2000; Cione and Uhlhorn, 2003; Karnauskas et al., 2021; Lin et al., 2005; Liu et al., 2007; Lloyd and Vecchi, 2011; Prasad and Hogan, 2007; Schade and Emanuel, 1999; Shay et al., 2000). Wind-driven vertical mixing is an important mechanism regulating upper-ocean changes for all high-wind events, including those that are not associated with tropical cyclones (Cardona and Bracco, 2012; Kuang et al., 2011; Large et al., 1994; Meng et al., 2020).
While several studies have investigated cyclone-induced changes in upper-ocean temperature and the role the ocean thermal structure plays in the intensification of cyclones (e.g., Zhang et al., 2021), cyclone-induced changes in upper-ocean salinity and the role of the vertical structure of the salinity profile in regulating TC-induced changes in upper-ocean stratification are not as well understood. This limits our understanding of air–sea interactions during and after the TC passage, as the degree of stratification of the water column is related to vertical gradients of both temperature and salinity, and it regulates air–sea exchanges of heat (e.g., Reul et al., 2014b; Vincent et al., 2014) and vertical mixing induced by atmospheric disturbances, including hurricanes (Holyer et al., 1987; Neetu et al., 2012). As an example, Balaguru et al. (2020) show how taking into account both temperature and salinity improves the prediction of TCs' rapid intensification. Satellite-based observations of sea surface salinity show salinification (a salty wake) after TC passage (Chaudhuri et al., 2019; Grodsky et al., 2012; Liu et al., 2020; Reul et al., 2014b, a, 2021; Bulusu et al., 2005; Vinayachandran and Mathew, 2003; Zhang et al., 2016), especially for stronger, slowly moving TCs (Reul et al., 2021), with greater effects on the right-hand side of the storms in the Northern Hemisphere (Sun et al., 2021) and the left-hand side of the storms in the Southern Hemisphere. The TC-induced salty wake has also been described based on in situ observations, generally for a single TC or for TCs over a specific region (Bond et al., 2011; Domingues et al., 2015; Sanford et al., 1987; Venkatesan et al., 2014; Zhang et al., 2018). A salty wake is consistent with TC-induced vertical mixing in regions where pre-event salinity increases with depth, i.e., as fresher water near the surface mixes with saltier water at depth.
A better understanding of freshwater redistribution in the upper ocean and its impacts on ocean stratification, during and after a weather event of interest, requires temperature and salinity measurements within the water column, such as those provided by Argo profiling floats. While Argo provides an unprecedented spatial and temporal coverage of the global ocean, most of the floats take measurements on a 10 d cycle (Roemmich et al., 2003, 2009); hence, a composite approach of all the weather events of interest where Argo profiles are available is helpful (Lin et al., 2017). Using Argo observations and a composite approach, Steffen and Bourassa (2018) quantify barrier layer development due to tropical cyclones. A barrier layer is the salinity-stratified isothermal layer situated between the base of the mixed layer and the top of the thermocline (Godfrey and Lindstrom, 1989) in some regions of the ocean, and it acts as a barrier to the turbulent entrainment of cold thermocline water into the surface mixed layer (Cronin and McPhaden, 2002). Steffen and Bourassa (2018) show a statistically significant increase in barrier layer thickness and barrier layer potential energy in the Atlantic basin with the TC passage, consistent with an increase in the post-TC isothermal layer depth due to vertical mixing. On the other hand, the eastern Pacific basin shows no significant changes to any barrier layer characteristic, likely due to a shallow and highly stratified pycnocline, while the central Pacific has a statistically significant freshening in the upper 20–30 m, which increases upper-ocean stratification by about 35 %. Tropical cyclones with different characteristics, such as intensity and forward speed, change the characteristics of the barrier layer (BL) thickness in different ways (Zhang et al., 2022): strong and slowly moving TCs have more impact on the upper-ocean properties, with pronounced increase in the barrier layer's thickness. Finally, Balaguru et al. (2012) show that the TC intensification rate is almost 50 % higher over regions with barrier layers compared to regions without. The presence of a preexisting ocean barrier layer can limit the effects of wind-driven vertical mixing and the near-surface cooling response (Wang et al., 2011). This is the case as while salinity increases from the bottom of the density-based mixed layer to the bottom of the barrier layer, temperature does not change much in the barrier layer, resulting in a more favorable ocean state for, e.g., the maintenance of a tropical cyclone, as the mixed layer and thermocline are decoupled. A long-term freshening of the upper ocean also tends to intensify the strongest TCs of the western North Pacific, as the increase in stratification reduces their ability to cool the upper ocean (Balaguru et al., 2016).
In this work, we use Argo float observations (Argo, 2000; Roemmich et al., 2003, 2009) to study TC-induced changes in upper-ocean properties, focusing on hurricane-strength TCs, i.e., cyclones with maximum sustained winds greater or equal to 64 kn (32.9 m s−1). We describe these changes and their uncertainties using the method by Hu et al. (2024) and compare cases where the pre-event salinity profile increases versus decreases with depth; i.e., we compare two different initial conditions. Our goal is to describe the association between the increasing versus decreasing vertical structures of the pre-event salinity profile and changes in upper-ocean salinity and density during hurricane-strength wind events, which has potential implications for upper-ocean stratification and air–sea exchanges during and after the event. If pre-event salinity increases with depth, wind-induced vertical mixing will result in an increase in near-surface salinity, as saltier waters from below are mixed in (Fig. 1b); if pre-event salinity decreases with depth, wind-induced vertical mixing will result in a decrease in near-surface salinity, as fresher waters from below are mixed in (Fig. 1d). For a given depth reached by the vertical mixing, the salinity change will be larger for pre-event salinity profiles with larger vertical gradients. The two different types of pre-event salinity profiles (Fig. 1b and d; Fig. S1a in the Supplement) and associated near-surface salinity changes cannot be captured when analyzing the presence versus absence of a pre-event barrier layer, and the composite pre-event profiles with and without a barrier layer both show a vertical structure of salinity that increases with depth (Fig. S1b). Differences in near-surface salinity changes for the increasing and decreasing case result in opposite contributions to the density changes with the weather event. As part of this study, we compare Argo-based results for hurricane-strength TCs with the upper-ocean response to hurricane-strength wind events in the HYCOM ocean reanalysis (Chassignet et al., 2007). The HYCOM reanalysis has been used in the past to investigate upper-ocean physical and biological processes during hurricane-strength wind events (e.g., Gierach et al., 2009; Prasad and Hogan, 2007; Zamudio and Hogan, 2008) and complements our analysis as it provides time series for each event of interest, instead of sparse pairs of oceanic profiles before and after weather events, like in the case of Argo observations. We find that results from both Argo and HYCOM are consistent with the vertical mixing of salinity playing a role in how upper-ocean stratification changes with the TC passage. This is also the case for hurricane-strength wind events in general, as shown using HYCOM to investigate composites from only hurricane-strength wind events that are not co-located with observed tropical cyclones. Finally, we show that our results for hurricane-strength wind events do not change when we consider only pre-event increasing profiles with or without a barrier layer.
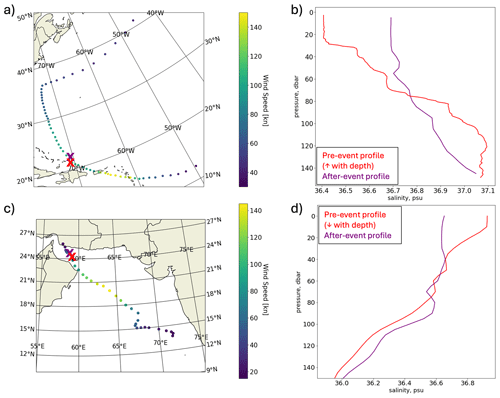
Figure 1(a, c) Paths of two tropical cyclones and location of Argo profiles close in space and time to the TC tracks and collected before (red marker) and after (purple marker) the TC passage. (b, d) Comparison between salinity profiles collected before (red line) versus after (purple line) the TC passage. One of the pre-event salinity profiles increases with depth (b), and the other decreases with depth (d).
2.1 Tropical cyclone track data
Tropical cyclone track data are available globally by combining products from the National Hurricane Center (NHC) and the United States Navy Joint Typhoon Warning Center (JTWC). The National Hurricane Center Data Archive (HURDAT2) database (Landsea and Franklin, 2013) includes TC track data for the North Atlantic and the eastern Pacific basins. The JTWC database (Chu et al., 2002) provides TC track records for the western Pacific Ocean, the Southern Hemisphere, and the north Indian Ocean.
Data are reported at 6-hourly resolution, since 1851 for the Atlantic hurricane database (HURDAT2), since 1949 for the northeast and north central Pacific (HURDAT2), and since 1945 for the JTWC best track data. In the following, we use TCs' spatiotemporal information and along-track wind speed during 2004–2020, i.e., during a time period when both TC track data and ocean temperature and salinity observations from Argo floats were available at the time of analysis.
2.2 HYCOM
The HYbrid Coordinate Ocean Model (HYCOM) reanalysis product is produced by the Naval Research Laboratory. In the following, we use output from the Global Ocean Forecast System (GOFS) 3.1 (experiment 53.X), 41-layer HYCOM + NCODA (Navy Coupled Ocean Data Assimilation) Global ° Reanalysis version of the product (Bleck, 2002; HYCOM Consortium, 2019). The vertical levels of HYCOM are different from those in other models as the vertical coordinates remain isopycnic in the open stratified ocean, smoothly transitioning to z-level coordinates in the weakly stratified upper-ocean mixed layer to terrain-following sigma coordinates in shallow water regions and back to z-level coordinates in very shallow water.
In our analysis, we focus on the time period 2011–2015, which overlaps with Argo observations, and when the model is forced by the National Centers for Environmental Prediction (NCEP) Climate Forecast System version 2 (CFSv2; Saha et al., 2014). We use HYCOM temperature and salinity fields (available at 3-hourly temporal resolution), as well as the wind forcing (available hourly). From the HYCOM temperature and salinity fields, potential density is estimated using the TEOS-10 oceanographic toolbox (McDougall and Barker, 2011). Mixed layer properties are estimated using the methodology described in Holte and Talley (2009), i.e., a hybrid algorithm that models the general shape of each profile, searches for physical features in the profile, and calculates threshold and gradient MLDs to assemble a suite of possible MLD values, before selecting a final MLD estimate. This method has been shown to also work well in regions where the mixed layer exhibits great variability and the estimate of the MLD is overall challenging, e.g., winter mixed layers north of the Subantarctic Front, which can reach depths of 500 m and blend into deeper waters and remnant mixed layers (Holte and Talley, 2009).
2.3 Argo
Argo floats provide an unprecedented coverage in space and time of global ocean temperature and salinity fields in the upper 2000 dbar (Argo, 2000; Roemmich et al., 2009), with extensions of the core array measuring ocean biogeochemical properties and at depths below 2000 dbar.
In the following, we use Argo temperature and salinity profiles during 2004–2020, a time period that overlaps with available TC tracks at the time of analysis. Potential density, conservative temperature, and absolute salinity are estimated from Argo-measured temperature, salinity, and pressure using the TEOS-10 oceanographic toolbox (McDougall and Barker, 2011). As for HYCOM, mixed layer properties are estimated using the methodology described in Holte and Talley (2009).
3.1 HYCOM
We use the HYCOM output with high resolution in space and time to investigate upper-ocean changes in the more general case of weather events with hurricane-strength winds (i.e., wind speed equal to or greater than 64 kn (32.9 m s−1)), before exploring upper-ocean changes for the specific case of hurricane-strength TCs using sparse ocean observations. In the following we focus on seven regions that include most of the events of interest between 32° S and 50° N (red boxes in Fig. 2a and b). For each region, we locate the events of interest starting from the maximum hurricane-strength wind event over the ocean, and we exclude, from the remaining data, any other event within ±2° and 7 d before and after the one selected, to ensure selected events are independent. We then proceed in selecting subsequent events, continuing with the strongest hurricane-strength wind event in the remaining wind data in the region: using this method, selected events are further than 7 d and ±2° from one another. We find that 32 % of the 1238 events identified here are not co-located with an observed tropical cyclone, i.e., are not within a 200 km radius and ±1 d of an observed TC (henceforth referred to as non-TC events); most of these non-TC events are found in the North Atlantic and Pacific, with 35 % in the North Atlantic box (in red in Fig. 2a and b) and 56 % in the northernmost box in the Pacific (in red in Fig. 2a and b).
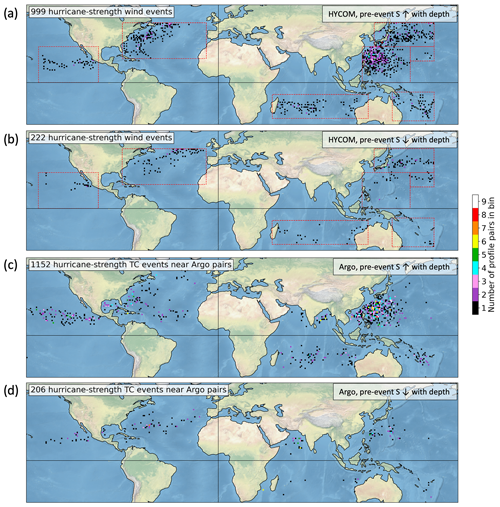
Figure 2(a, b) Number of weather events included in the HYCOM analysis, in 1°×1° bins, i.e., weather events with hurricane-strength winds during 2011–2015 and within the regions outlined in red. (c, d) Number of Argo profile pairs included in the Argo analysis, in 1°×1° bins, i.e., Argo profile pairs co-located with hurricane-strength TCs during 2004–2020. Panels (a) and (c) are for locations where pre-event upper-ocean salinity increases with depth. Panels (b) and (d) are for locations where pre-event upper-ocean salinity decreases with depth.
At the location of each selected hurricane-strength wind event, we store the time series of oceanic properties of interest, estimate the seasonal cycle, and remove it from the data to isolate the impact of hurricane-strength wind events on the upper ocean. To characterize this event-related signal, we analyze changes in oceanic properties (e.g., absolute salinity and potential density) compared to 2 d before the event and focus on how the upper ocean evolves in the 14 d after the event. We use the profile 2 d before the event for the pre-event state to hedge against storm effects prior to the event, consistent with Hu et al. (2024), Cheng et al. (2015), and with the method used for Argo in the next subsection.
We group selected events based on the pre-event vertical structure of salinity from the HYCOM reanalysis, i.e., the vertical structure 2 d before the event: increasing events are located where pre-event absolute salinity increases between the density-based mixed layer and 50 m below the mixed layer (e.g., Fig. 1b); decreasing events are located where salinity decreases between the mixed layer and 50 m below (e.g., Fig. 1d). The number of increasing events is much larger than the number of decreasing events, with the latter mostly located in the Northern Hemisphere (Fig. 2). We use 50 m for the thickness of the layer considered below the mixed layer, as it captures the 90th percentile (across events) of the observed mixed layer deepening (not shown). We find our conclusions in the following do not change with the thickness of the layer considered below the mixed layer, e.g., if we consider 20 to 70 m below the mixed layer.
Composites of seasonally adjusted differences between profiles at each time step and the profile 2 d before the event are created for the increasing and decreasing group, and the two groups are compared; see Figs. 3 and 4. For each composite, we compute the standard error and use it to identify the significant signal (95 % confidence level). As the two groups of events are independent by construction, the variance of the difference (between increasing and decreasing) is estimated as the sum of the variances.
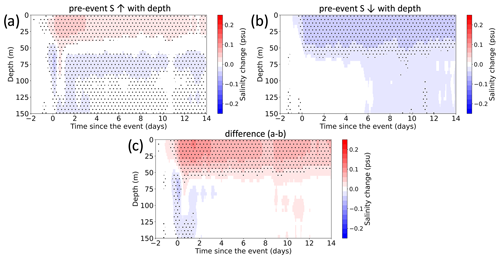
Figure 3Upper-ocean salinity changes during hurricane-strength wind events, based on the HYCOM reanalysis. Composite changes are shown for regions where pre-event upper-ocean salinity increases (a) vs. decreases (b) with depth. Panel (c) shows the difference between the increasing case (i.e., panel a) and the decreasing case (i.e., panel b). Dots indicate statistically significant values (95 % confidence limit).
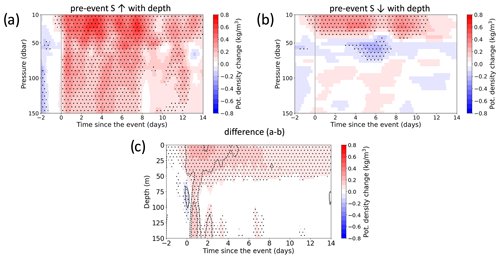
Figure 4As in Fig. 3 but for potential density. Black contours in panel (c) indicate differences in temperature change between the increasing and decreasing case and are shown only when significant, as in Fig. S2c. A continuous line indicates positive values; a dashed line indicates negative values.
3.2 Argo
Our analysis of TC-related upper-ocean changes from (sparse) Argo observations is based on the differences between post-TC and pre-TC Argo profiles, as described in Hu et al. (2024). Hu et al. (2024) introduced a framework that, for the first time, (1) accounts for seasonal effects to isolate the signal of interest, (2) models space–time covariances to provide rigorous uncertainty quantification for results from sparse observations, and (3) treats time as a continuous variable (rather than producing estimates that are binned in time, as other studies). In the following, we provide a summary of the method in Hu et al. (2024) and include a description of differences in our implementation.
3.2.1 Defining a TC-centric coordinate system
Given the scattered nature, in space and time, of the Argo data, we define a TC-centric coordinate system to characterize the ocean response to TCs' passage in the continuous time and space realms (Hu et al., 2024). One coordinate is the time difference between the post-TC Argo profile and the TC passage. The other coordinate is the cross-track angle, which is determined by calculating the angle between line segments from the sphere's center to two surface points, equivalent to the haversine distance. When longitude is constant, this angle is the absolute difference in latitude. When latitude is constant, it is the absolute difference in longitude, adjusted by the cosine of the latitude. The cross-track angle aligns with the great circle distance and is used here as it provides a more accurate spherical model for measuring the distance from the TC track, compared to, e.g., using distance in kilometers.
3.2.2 Selecting Argo profile pairs to characterize changes with TC passage
To investigate TC-related upper-ocean changes, we select TC profiles that are within 12 d before and 20 d after a TC event and within a cross-track angle of ±2° of a TC event, i.e., ±2° of a TC track data point; as indicated earlier, we only consider TC track data points that correspond to hurricane-strength TCs. All the other Argo profiles, which represent the vast majority, are classified as non-TC profiles.
TC profiles are used to create pairs of Argo profiles: each pair includes one profile before a TC and one profile after, and their difference is used to assess the ocean response to TCs. To be paired, two Argo profiles need to be both incidental to a TC track (within ±2° in cross-track angle) and co-located in space (within ±0.2°), with a time separation of 32 d or less. As in Hu et al. (2024), the pre-TC Argo profile, which we can also call the baseline profile, needs to be between 12 and 2 d before the TC passage; the post-TC Argo profile, which we also call the signal profile, needs to be between 2 d before and 20 d after the TC passage to hedge against storm effects prior to the event, as described in Hu et al. (2024) and consistent with Cheng et al. (2015).
3.2.3 Classification of profiles based on the pre-event vertical structure of salinity
As for HYCOM and differently from Hu et al. (2024), we group Argo profile pairs based on the vertical structure of the Argo salinity profile before the weather event: increasing pairs include Argo pre-TC profiles with upper-ocean salinity increasing with depth (e.g., Fig. 1b); decreasing pairs include Argo pre-TC profiles with salinity decreasing with depth (e.g., Fig. 1d). For each of the two groups, we estimate upper-ocean changes with the TC passage and compare them to one another to characterize differences associated with the pre-event vertical structure of salinity. As for HYCOM, there are many more instances for the increasing case compared to the decreasing case (Fig. 2c and d). We note that, while the total counts in Fig. 2 (white box on the top left of each map) are comparable between HYCOM and Argo, the numbers have different meanings. For HYCOM, the number indicates the count of all selected weather events, hence the number of continuous time series of ocean temperature/salinity available for the analysis, as the model output is available at the location of the weather event at all times of the model simulation. For Argo, the number indicates the count of all available profile pairs to estimate the upper-ocean response to the TC passage, as we only have sparse observations from Argo floats.
3.2.4 Estimating and removing effects of the seasonal cycle
To isolate the effect of hurricane-strength TCs on upper-ocean properties based on differences between post-event and pre-event profiles, the seasonal cycle needs to be removed from the data. For example, as observed in Hu et al. (2024), the TC season is usually associated with a seasonal warming, which therefore needs to be removed from the data to study TC-related upper-ocean changes. As in Hu et al. (2024), we estimate the seasonal cycle using the local linear regression method in Roemmich and Gilson (2009) and all the Argo profiles that were classified as non-TC. First, we calculate the local linear regression on a 1°×1° grid; then, each TC profile is matched to the closest grid point to remove the seasonal cycle from that profile; i.e., we subtract the seasonal mean field estimate from the pre-TC and post-TC Argo profiles to then analyze seasonally adjusted differences.
3.2.5 Determining the statistically significant, smoothed results
In addition to the TC-related signal of interest, seasonally adjusted variables from TC-profiles include effects of ocean variability that is unrelated to TCs, i.e., variability that represents noise in our analysis. As in Hu et al. (2024), we characterize this variability by fitting the Gaussian process model described in Kuusela and Stein (2018) to non-TC Argo profiles. Once fitted, the model is used to provide information about ocean variability at the time and locations of the TC profiles and estimate self-variances and cross-covariances of seasonally adjusted variables. As in Hu et al. (2024), only the non-TC activity is represented here as a random process; hence, the variance of the before and after profiles is the same under this model. The estimate of self-variances and cross-covariances of seasonally adjusted variables allows us to account for cross-observation dependence and point-wise variance when smoothing seasonally adjusted differences between the post-TC profile and the pre-TC profile, to characterize the upper-ocean response to the passage of hurricane-strength TCs as a continuous function of cross-track angle and time, for different vertical levels. Notably, this allows us to obtain point-wise confidence intervals on our estimates (α=0.05, i.e., to obtain 95 % coverage) and thus to assess the significance of each individual pixel in our plots, as shown in Figs. 5 and 6. We smooth seasonally adjusted differences using a fixed-knot thin plate spline smoother (Duchon, 1977; Green and Silverman, 1993; Nychka, 2000; Wahba, 1980, 1990; Wood, 2017).
4.1 Upper-ocean changes during hurricane-strength wind events in HYCOM
Consistent with previous studies (Black and Dickey, 2008; Han et al., 2024; Hu et al., 2024; Korty et al., 2008; Price, 1981; Zhang et al., 2021), the analysis of HYCOM fields shows that, during hurricane-strength wind events, upper-ocean temperature decreases (Fig. S2a and b in the Supplement). Several factors can, however, impact the magnitude of such change. As an example, the presence or absence of a strong barrier layer can significantly influence the heat exchange processes at the air–sea interface and affect, e.g., the intensity and track of tropical cyclones (Balaguru et al., 2012; Reul et al., 2021; Wang et al., 2011). A strong barrier layer reduces the cooling of the upper ocean during the passage of a TC (e.g., Reul et al., 2021), as it can limit the vertical mixing of cold waters from below the mixed layer, allowing the storm to maintain and even intensify its strength (Balaguru et al., 2012). Also, with a pre-event barrier layer, when vertical mixing reaches below the density-based mixed layer, it may only mix in waters with similar temperature, as temperature within the barrier layer is similar to the one above by definition. On the other hand, in the absence of a pre-event barrier layer, the upper-ocean cooling with the TC passage is larger, which may weaken the storm. While not the focus of our analysis, the comparison of HYCOM temperature composites based on the presence or absence of a barrier layer before a hurricane-strength wind event yields results that are consistent with previous TC studies; i.e., the cooling after the event is overall larger in the absence of a barrier layer (not shown). A difference in cooling is also observed when comparing HYCOM composites based on the vertical structure of salinity before the event, i.e., pre-event salinity increasing versus decreasing (instead of pre-event barrier layer present versus not present). In particular, the cooling is larger for the increasing case (Fig. S2a) than for the decreasing case (Figs. S2b and 4c). The increasing case includes most of the events, with ≈30 % of these events in regions with a barrier layer. If we compare increasing vs. decreasing composites for only events with no pre-event barrier layer, we still find a larger cooling of the top ≈15 m for the increasing case in the days after the event (Fig. S3d–f in the Supplement), yet the difference is much smaller and limited to day 2 to 5 after the event (Fig. S2f). Also, upper-ocean temperature differences between the increasing and decreasing case are not significant when only considering non-TC hurricane-strength wind events (Fig. S4d–f in the Supplement). We note that factors such as wind strength and duration of the strongest winds are key to understanding the upper-ocean temperature response to weather events, i.e., storm intensity, size, and translation speed affect the characteristics of the upper-ocean temperature response to the event (Anthes and Chang, 1978; Emanuel and Nolan, 2004; Lin et al., 2017; Samson et al., 2014; Wang et al., 2016; Zhu and Zhang, 2006). Isolating the difference in cooling between the increasing and decreasing case from other relevant factors may be difficult with a limited number of events; hence, it is not a focus here. The discussion of temperature is included as relevant to better understand results for density presented later in this section.
Consistent with wind-induced vertical mixing, near-surface salinity increases in regions where pre-event salinity increases with depth, due to fresher waters mixing with saltier waters below (Fig. 3a); vice versa, near-surface salinity decreases where pre-event salinity decreases with depth, as saltier waters mix with fresher water below (Fig. 3b). More specifically, for the increasing composite, ocean salinity increases by over 0.05 psu in the top 40 m after hurricane-strength wind events (Fig. 3a). This positive anomaly weakens in time, yet it is still detectable after 14 d. For the decreasing composite, a decrease by over 0.05 psu is seen instead (Fig. 3b). The difference between the two composites is statistically significant (Fig. 3c). This is also seen when only locations with no pre-event barrier layer are considered (Fig. S3a–c). Additionally, the same result is found when only non-TC hurricane-strength wind events are included in the composites (Fig. S4a–c). For the increasing composite, salinity decreases between 50 and 100 m, yet the signal is weaker compared to the increase near the surface: this difference in the sign of salinity changes at different depths is consistent with wind-induced vertical mixing acting on regions with a pre-event salinity that increases with depth; i.e., near-surface waters get saltier as they mix with saltier waters at depth; deeper layers get fresher as they mix with lower-salinity waters above. Yet, while an increase in salinity near the surface is common to most profiles in the composite, where the decrease happens in the water column may depend on the details of the stratification at different locations, and a switch in sign of the observed changes with depth may not have appeared in the composite; e.g., for the decreasing case, no significant salinity increase is detected in the top 150 m (Fig. 3b). As for temperature, results for salinity in this study are consistent with previous research reporting sea surface salinity (SSS) salinification in the trail of TCs (e.g., Bond et al., 2011; Chaudhuri et al., 2019; Domingues et al., 2015; Grodsky et al., 2012; Lin et al., 2017; Liu et al., 2020; McPhaden et al., 2009; Price, 1981; Reul et al., 2014b; Sanford et al., 1987; Steffen and Bourassa, 2018; Venkatesan et al., 2014; Vinayachandran and Mathew, 2003; Zhang et al., 2016, 2018). This SSS salinification, observed from satellites particularly for slow-moving TCs, is consistent with the importance of vertical mixing during hurricane-strength wind events and the fact that subsurface water is on average saltier than surface water in convective regions associated with TC activity (Jourdain et al., 2013); hence, not differentiating between the increasing versus decreasing case as in the present study will yield a result closer to the increasing case. Also, as described in Reul et al. (2021) and found here in HYCOM composites (not shown), barrier layers lead to storm wakes that are saltier compared to wakes over barrier-layer-free areas.
Finally, consistent with the described cooling, for both increasing and decreasing composites, upper-ocean potential density increases with the passage of hurricane-strength wind events (Fig. 4a and b). The increase in density is larger for the increasing case, as both the cooling is stronger and upper-ocean salinity increases, and the difference with the decreasing case is statistically significant (Fig. 4c). While both temperature and salinity contribute to the stronger increase in density for the increasing case, an estimate of the temperature versus salinity contribution to the difference between the increasing versus decreasing composites for density indicates salinity contributes around 35 %–40 % (not shown). As for temperature and salinity, similar results are found for the difference between increasing and decreasing composites for density when only locations with no pre-event barrier layer are considered (Fig. S3g–i). This is also the case when only non-TC hurricane-strength wind events are included in the composites (Fig. S4a–c). While results here are overall consistent with previous studies, our analysis shows the importance of an increasing versus decreasing vertical structure of pre-event salinity for how density changes in the upper ocean with hurricane-strength wind events, which can modulate air–sea interactions during and after the events through changes in stratification.
4.2 Upper-ocean changes observed by Argo floats during hurricane-strength tropical cyclones
While HYCOM assimilates observations to provide a model representation of relevant processes at high resolution in space and time, the question remains of how results in Sect. 4.1 compare with in situ measurements during relevant weather events. In the following, we discuss differences and similarities between the Argo- versus HYCOM-based results, and we refer to the previous section for some of the context from previous studies.
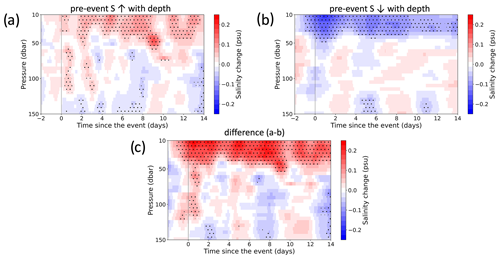
Figure 5Upper-ocean salinity changes within 0.5° in cross-track angle from the TC track during hurricane-strength TCs, based on Argo observations. Changes are shown for regions where pre-event upper-ocean salinity increases (a) vs. decreases (b) with depth. Panel (c) shows the difference between the increasing case (i.e., panel a) and the decreasing case (i.e., panel b). In all panels, a point-wise α=0.05 hypothesis test is performed and used to indicate (with dots) where the null hypothesis is rejected.
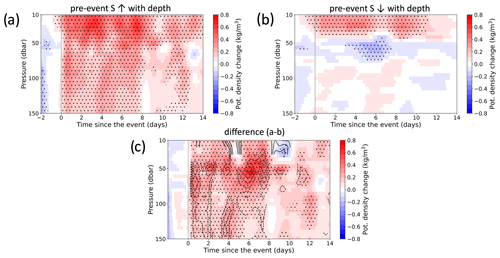
Figure 6As in Fig. 5 but for potential density. Black contours in panel (c) indicate differences in temperature change between the increasing and decreasing case and are shown only when significant, as in Fig. S5c. A continuous line indicates positive values; a dashed line indicates negative values.
Consistent with results in Sect. 4.1 and several previous studies (including analyses based on Argo data, e.g., Liu et al., 2007; Cheng et al., 2015), Argo observations show upper-ocean cooling as hurricane-strength TCs move over the ocean, for both the increasing case and the decreasing case (Fig. S2a). Different from HYCOM, observed cooling for the increasing case in Argo is detected as deep as 150 dbar for the 2 weeks after the event (Fig. S5a in the Supplement), i.e., deeper than what is seen in HYCOM fields for hurricane-strength wind events. This difference may be related to, e.g., how vertical mixing processes are represented in the HYCOM model and in particular how deep the wind-induced mixing reaches with the event; the details of the vertical structure of pre-event upper-ocean properties in the model versus observations, i.e., the initial condition of events of interest; and the availability of sparse ocean observations co-located with events of interest compared to the continuous time series the HYCOM model provides in its spatial domain. Also, for the decreasing case in Argo, a warming is observed between 50 and 70 dbar (Fig. S5b). Analogous to what was discussed for Fig. 3a, vertical mixing of the water column may result in the observed warming, as warmer waters above are mixed downward. However, it is not surprising that the same vertical pattern of changes, with different signs at different depths, is not seen in Fig. S5a. While the near-surface cooling is common across locations used to estimate ocean changes for the increasing versus decreasing composite, the depth of the warming signal and its vertical extent vary (analogous to that discussed for Fig. 3a). The challenges described in Sect. 4.1 to characterize the differences in temperature changes between the increasing and decreasing case apply also to results from sparse Argo observations. While the cooling below 50 dbar is stronger for the increasing case compared to decreasing case, the cooling in the top 50 dbar is stronger for the decreasing case or not statistically different between the two groups (Figs. 6 and S5c). As for HYCOM, differences in temperature changes between the increasing case and the decreasing case are not the focus of our analysis, yet Figs. 6 and S5c are helpful to interpret differences in density changes later in this section.
Upper-ocean salinity and density changes observed by Argo during hurricane-strength TCs are overall consistent with HYCOM-based results (Sect. 4.1). Consistent with wind-induced vertical mixing, salinity increases with the TC passage for the increasing case, and it decreases for the decreasing case (Fig. 5). Hence, salinity enhances or reduces the upper-ocean increase in density that occurs as the upper ocean cools with the TC passage (Fig. 6). Even if overall changes in salinity and density are consistent between the Argo and HYCOM analyses, some of the details may be different. As an example, the vertical structure of Argo-based density changes for the decreasing case reflects the vertical structure of changes in temperature described earlier in this subsection, i.e., a switch in sign between shallower and deeper layers; hence, it is different from HYCOM. Also, different from that discussed for HYCOM at the end of the previous subsection, in Argo, the differences in upper-ocean density changes between the increasing and decreasing case largely reflect differences in salinity changes (Fig. 5c, top 50 dbar), rather than both differences in temperature changes (Fig. S5c) and salinity changes: the stronger increase in density for the increasing case is associated with salinity increasing where pre-event salinity increases with depth, as wind-induced mixing brings up saltier water, and is observed despite a weaker cooling for the increasing case compared to the decreasing case.
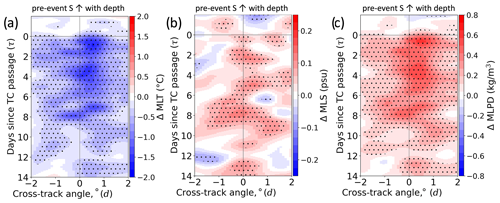
Figure 7Upper-ocean changes in mixed layer temperature (MLT, a), mixed layer salinity (MLS, b), and mixed layer potential density (MLPD, c) during hurricane-strength tropical cyclones, based on Argo observations. Changes are shown in regions where pre-TC salinity increases with depth. In all panels, a point-wise α=0.05 hypothesis test is performed and used to indicate (with dots) where the null hypothesis is rejected.
Finally, Argo-based changes in mixed layer properties with the TC passage largely reflect what was described earlier for changes at different vertical levels and in time. Looking at these changes as a function of the cross-track angle (e.g., Fig. 7 for the increasing case) shows the asymmetric signature of wind-driven vertical mixing with the TC passage; a stronger signal is observed on the right-hand side of the storms in the Northern Hemisphere and on the left-hand side of the storms in the Southern Hemisphere, e.g., documented for salinity in Sun et al. (2021). We note that, while panels in Fig. 7 include events in both the Northern Hemisphere and Southern Hemisphere, we flip the sign of the cross-track angle for TCs in the Southern Hemisphere when estimating changes in ocean properties, in order to display the asymmetry in a consistent manner across hemispheres.
In this study, we characterize observed upper-ocean changes during hurricane-strength tropical cyclones. We focus on (1) the statistical significance of the changes associated with different pre-event vertical structures of salinity, i.e.increasing versus decreasing with depth, and (2) the contribution of salinity changes to upper-ocean density changes in the increasing versus decreasing case. Upper-ocean salinity increases with the TC passage in increasing locations, i.e., the majority of the areas with cyclonic activity; vice versa, it decreases in decreasing locations. This observed difference is consistent with wind-induced vertical mixing of the upper ocean with the TC passage; e.g., for the increasing case, fresher near-surface waters mix with saltier waters below. The difference in salinity changes for the increasing versus decreasing case results in differences in how upper-ocean density changes: while near-surface density increases in both the increasing case and the decreasing case, consistent with cooling due to air–sea exchanges of heat and vertical mixing, it increases more for the increasing case, due to the contribution from the salinity increase, with potential changes in stratification; i.e., as density increases with depth in a stable water column, a larger increase in near-surface density is consistent with a larger decrease in stratification. Results from Argo observations are discussed in the more general context of upper-ocean changes during hurricane-strength wind events (not just tropical cyclones) using the HYCOM reanalysis. Results from Argo and HYCOM are overall consistent with one another and complement each other, as while HYCOM provides high-resolution fields in space and time, it may be limited in representing all relevant processes and in capturing the details of the pre-event vertical structure of upper-ocean properties in the real ocean.
Our focus on the association between the pre-event vertical structure of salinity, increasing versus decreasing with depth, and upper-ocean salinity and density changes with the TC passage adds to the existing literature on air–sea interactions during TCs. Changes in the vertical structure of upper-ocean density may have implications for air–sea interactions during the weather event and after; hence, a better understanding of relevant processes has the potential to improve the prediction of TC intensification and, more in general, the model representation of air–sea interactions during the season when hurricane-strength wind events occur. As an example, insights from our work could inform process model experiments to study air–sea interactions in increasing versus decreasing regions during and after hurricane-strength wind events, along the lines of, e.g., Balaguru et al. (2012), Hoffman et al. (2022), and Iyer and Drushka (2021) (not all focusing on TCs).
Several opportunities also exist to extend our work in other directions, including for other weather events of interest or using satellite observations. As one example, changes in the ocean biogeochemistry during hurricane-strength TCs or other weather events could be investigated using our framework, as the fleet of biogeochemical Argo floats grows in time, e.g., changes in chlorophyll a, oxygen, nitrate, and pH (Bittig et al., 2019). Finally, our framework can be leveraged to compare how different reanalysis products represent air–sea exchanges during weather events in the context of the insights from sparse observations.
Argo data were collected and made freely available by the International Argo Program and the national programs that contribute to it (https://argo.ucsd.edu, https://www.ocean-ops.org, last access: 21 June 2024; https://doi.org/10.17882/42182, Argo, 2000). The International Argo Program is part of the Global Ocean Observing System. Tropical cyclone track data are made available by NOAA (https://www.nhc.noaa.gov/data/#hurdat, Landsea and Franklin, 2013) and the Joint Typhoon Warning Center (https://www.metoc.navy.mil/jtwc/jtwc.html?best-tracks, Chu et al., 2002). HYCOM data are available at https://www.hycom.org/data/glbv0pt08/expt-53ptx (HYCOM, 2024). Argo profiles and tropical cyclone track data were also accessed via Argovis (https://argovis.colorado.edu, Tucker et al., 2020; https://github.com/argovis/demo_notebooks, Giglio and Mill, 2024).
The supplement related to this article is available online at: https://doi.org/10.5194/os-20-1441-2024-supplement.
All authors contributed to the conceptualization and design of this study. JS conducted the analysis and wrote the manuscript, with contributions from DG, AH, and MK. The final manuscript underwent a thorough review and editing process, led by JS, DG, AH, MK, and KMW, ensuring its quality and accuracy.
The contact author has declared that none of the authors has any competing interests.
Publisher's note: Copernicus Publications remains neutral with regard to jurisdictional claims made in the text, published maps, institutional affiliations, or any other geographical representation in this paper. While Copernicus Publications makes every effort to include appropriate place names, the final responsibility lies with the authors.
We would like to acknowledge high-performance computing support from the supercomputer Cheyenne provided by the National Center for Atmospheric Research's (NCAR) Computational and Information Systems Laboratory, sponsored by the National Science Foundation (NSF). Argo data were collected and made freely available by the International Argo Program and the national programs that contribute to it (https://argo.ucsd.edu, https://www.ocean-ops.org). The International Argo Program is part of the Global Ocean Observing System. Funding for the development of HYCOM has been provided by the National Oceanographic Partnership Program and the Office of Naval Research. Data assimilative products using HYCOM are funded by the United States Navy. Computer time was made available by the Department of Defense (DoD) High Performance Computing Modernization Program. The output is publicly available at https://hycom.org (last access: 14 June 2024).
Jacopo Sala and Donata Giglio were supported by NSF award 1928305, NASA award 80NSSC19K0059, and NOAA award NA21OAR4310261. Addison J. Hu was supported by the NSF GRFP (award DGE175016) and NSF DMS (award 1520786). Mikael Kuusela was supported by NOAA award NA21OAR4310258.
This paper was edited by Mario Hoppema and reviewed by two anonymous referees.
Anthes, R. A. and Chang, S. W.: Response of the hurricane boundary layer to changes of sea surface temperature in a numerical model, J. Atmos. Sci., 35, 1240–1255, https://doi.org/10.1175/1520-0469(1978)035<1240:ROTHBL>2.0.CO;2, 1978. a
Argo: Argo float data and metadata from global data assembly centre (Argo GDAC), Seanoe [data set], https://doi.org/10.17882/42182, 2000. a, b, c
Balaguru, K., Chang, P., Saravanan, R., Leung, L. R., Xu, Z., Li, M., and Hsieh, J.-S.: Ocean barrier layers' effect on tropical cyclone intensification, P. Natl. Acad. Sci. USA, 109, 14343–14347, 2012. a, b, c, d, e
Balaguru, K., Foltz, G. R., Leung, L. R., and Emanuel, K. A.: Global warming-induced upper-ocean freshening and the intensification of super typhoons, Nat. Commun., 7, 1–8, 2016. a
Balaguru, K., Foltz, G. R., and Leung, L. R.: Increasing magnitude of hurricane rapid intensification in the central and eastern tropical Atlantic, Geophys. Res. Lett., 45, 4238–4247, 2018. a
Balaguru, K., Foltz, G. R., Leung, L. R., Kaplan, J., Xu, W., Reul, N., and Chapron, B.: Pronounced impact of salinity on rapidly intensifying tropical cyclones, B. Am. Meteorol. Soc., 101, E1497–E1511, 2020. a
Bender, M. A. and Ginis, I.: Real-case simulations of hurricane–ocean interaction using a high-resolution coupled model: Effects on hurricane intensity, Mon. Weather Rev., 128, 917–946, 2000. a
Bhatia, K. T., Vecchi, G. A., Knutson, T. R., Murakami, H., Kossin, J., Dixon, K. W., and Whitlock, C. E.: Recent increases in tropical cyclone intensification rates, Nat. Commun., 10, 635, https://doi.org/10.1038/s41467-019-08471-z, 2019. a
Bittig, H. C., Maurer, T. L., Plant, J. N., Schmechtig, C., Wong, A. P. S., Claustre, H., Trull, T. W., Udaya Bhaskar, T. V. S., Boss, E., Dall’Olmo, G., Organelli, E., Poteau, A., Johnson, K. S., Hanstein, C., Leymarie, E., Le Reste, S., Riser, S. C., Rupan, A. R., Taillandier, V., Thierry, V., and Xing, X.: A BGC-Argo guide: Planning, deployment, data handling and usage, Frontiers in Marine Science, 6, 502, https://doi.org/10.3389/fmars.2019.00502, 2019. a
Black, W. J. and Dickey, T. D.: Observations and analyses of upper ocean responses to tropical storms and hurricanes in the vicinity of Bermuda, J. Geophys. Res.-Oceans, 113, C08009, https://doi.org/10.1029/2007JC004358, 2008. a
Bleck, R.: An oceanic general circulation model framed in hybrid isopycnic-Cartesian coordinates, Ocean Model., 4, 55–88, 2002. a
Bond, N. A., Cronin, M. F., Sabine, C., Kawai, Y., Ichikawa, H., Freitag, P., and Ronnholm, K.: Upper ocean response to Typhoon Choi-Wan as measured by the Kuroshio Extension Observatory mooring, J. Geophys. Res.-Oceans, 116, C02031, https://doi.org/10.1029/2010JC006548, 2011. a, b
Bulusu, S., Murty, V. S. N., Sharp, R. J., and O'Brien, J. J.: Air-sea coupling during the tropical cyclones in the Indian Ocean: A case study using satellite observations, Pure Appl. Geophys., 162, 1643–1672, 2005. a
Cardona, Y. and Bracco, A.: Enhanced vertical mixing within mesoscale eddies due to high frequency winds in the South China Sea, Ocean Model., 42, 1–15, 2012. a
Chan, J. C.: The physics of tropical cyclone motion, Annu. Rev. Fluid Mech., 37, 99–128, 2005. a
Chassignet, E. P., Hurlburt, H. E., Smedstad, O. M., Halliwell, G. R., Hogan, P. J., Wallcraft, A. J., Baraille, R., and Bleck, R.: The HYCOM (hybrid coordinate ocean model) data assimilative system, J. Marine Syst., 65, 60–83, 2007. a
Chaudhuri, D., Sengupta, D., D'Asaro, E., Venkatesan, R., and Ravichandran, M.: Response of the salinity-stratified Bay of Bengal to cyclone Phailin, J. Phys. Oceanogr., 49, 1121–1140, 2019. a, b
Cheng, L., Zhu, J., and Sriver, R. L.: Global representation of tropical cyclone-induced short-term ocean thermal changes using Argo data, Ocean Sci., 11, 719–741, https://doi.org/10.5194/os-11-719-2015, 2015. a, b, c
Chu, J.-H., Sampson, C. R., Levine, A. S., and Fukada, E.: The joint typhoon warning center tropical cyclone best-tracks, 1945–2000, Ref. NRL/MR/7540-02-16, https://www.metoc.navy.mil/jtwc/jtwc.html?best-tracks (last access: 27 April 2024), 2002. a, b
Cione, J. J. and Uhlhorn, E. W.: Sea surface temperature variability in hurricanes: Implications with respect to intensity change, Mon. Weather Rev., 131, 1783–1796, 2003. a
Cione, J. J., Kalina, E. A., Zhang, J. A., and Uhlhorn, E. W.: Observations of air–sea interaction and intensity change in hurricanes, Mon. Weather Rev., 141, 2368–2382, 2013. a
Cronin, M. F. and McPhaden, M. J.: Barrier layer formation during westerly wind bursts, J. Geophys. Res.-Oceans, 107, SRF 21-1–SRF 21-12, https://doi.org/10.1029/2001JC001171, 2002. a
Cui, H., Tang, D., Mei, W., Liu, H., Sui, Y., and Gu, X.: Predicting Tropical Cyclone-Induced Sea Surface Temperature Responses Using Machine Learning, Geophys. Res. Lett., 50, e2023GL104171, https://doi.org/10.1029/2023GL104171, 2023. a
Domingues, R., Goni, G., Bringas, F., Lee, S.-K., Kim, H.-S., Halliwell, G., Dong, J., Morell, J., and Pomales, L.: Upper ocean response to Hurricane Gonzalo (2014): Salinity effects revealed by targeted and sustained underwater glider observations, Geophys. Res. Lett., 42, 7131–7138, 2015. a, b
Duchon, J.: Splines minimizing rotation-invariant semi-norms in Sobolev spaces, in: Constructive Theory of Functions of Several Variables: Proceedings of a Conference, Oberwolfach, 25 April–1 May 1976, Springer, 85–100, https://doi.org/10.1007/BFb0086566, 1977. a
Elsberry, R. L., Fraim, T. S., and Trapnell Jr., R. N.: A mixed layer model of the oceanic thermal response to hurricanes, J. Geophys. Res., 81, 1153–1162, 1976. a
Emanuel, K. A.: Thermodynamic control of hurricane intensity, Nature, 401, 665–669, 1999. a
Emanuel, K. A.: Tropical cyclones, Annu. Rev. Earth Pl. Sc., 31, 75–104, 2003. a
Emanuel, K. A. and Nolan, D. S.: Tropical cyclone activity and the global climate system, Vol. 10, in: Preprints, 240–241, 26th Conf. on Hurricanes and Tropical Meteorology, Miami, FL, Am. Meteor. Soc. A, https://ams.confex.com/ams/pdfpapers/75463.pdf (last access: 18 October 2024), 2004. a
Fisher, E. L.: Hurricanes and the sea-surface temperature field, J. Atmos. Sci., 15, 328–333, 1958. a
Gierach, M. M., Subrahmanyam, B., and Thoppil, P. G.: Physical and biological responses to Hurricane Katrina (2005) in a nested Gulf of Mexico HYCOM, J. Marine Syst., 78, 168–179, 2009. a
Giglio, D. and Mills, B. K.-A.: Argovis Demo Notebooks, GitHub [data set], https://github.com/argovis/demo_notebooks, last access: 18 October 2024. a
Godfrey, J. S. and Lindstrom, E. J.: The heat budget of the equatorial western Pacific surface mixed layer, J. Geophys. Res.-Oceans, 94, 8007–8017, https://doi.org/10.1029/JC094iC06p08007, 1989. a
Green, P. J. and Silverman, B. W.: Nonparametric regression and generalized linear models: a roughness penalty approach, CRC Press, https://doi.org/10.1201/b15710, 1993. a
Greening, H., Doering, P., and Corbett, C.: Hurricane Impacts on Coastal Ecosystems, Estuar. Coast., 29, 877–879, 2006. a
Grodsky, S. A., Reul, N., Lagerloef, G., Reverdin, G., Carton, J. A., Chapron, B., Quilfen, Y., Kudryavtsev, V. N., and Kao, H.-Y.: Haline hurricane wake in the Amazon/Orinoco plume: AQUARIUS/SACD and SMOS observations, Geophys. Res. Lett., 39, 20, https://doi.org/10.1029/2012GL053335, 2012. a, b
Han, C.: Tropical Cyclone Induced Upper Ocean Response in the South Pacific observed by Argo floats, PhD thesis, ResearchSpace@ Auckland, https://hdl.handle.net/2292/64867 (last access: 18 October 2024), 2023. a
Han, C., Bowen, M., and Sutton, P.: The response of the upper ocean to tropical cyclones in the South Pacific, J. Geophys. Res.-Oceans, 129, e2023JC020627, https://doi.org/10.1029/2023JC020627, 2024. a, b
Hoffman, L., Mazloff, M. R., Gille, S. T., Giglio, D., and Varadarajan, A.: Ocean Surface Salinity Response to Atmospheric River Precipitation in the California Current System, J. Phys. Oceanogr., 52, 1867–1885, https://doi.org/10.1175/JPO-D-21-0272.1, 2022. a
Holte, J. and Talley, L.: A new algorithm for finding mixed layer depths with applications to Argo data and Subantarctic Mode Water formation, J. Atmos. Ocean. Tech., 26, 1920–1939, 2009. a, b, c
Holyer, J. Y., Jones, T., Priestley, M., and Williams, N.: The effect of vertical temperature and salinity gradients on double-diffusive interleaving, Deep-Sea Res., 34, 517–530, 1987. a
Hu, A. J., Kuusela, M., Lee, A. B., Giglio, D., and Wood, K. M.: Spatiotemporal methods for estimating subsurface ocean thermal response to tropical cyclones, Adv. Stat. Clim. Meteorol. Oceanogr., 10, 69–93, https://doi.org/10.5194/ascmo-10-69-2024, 2024. a, b, c, d, e, f, g, h, i, j, k, l, m, n
HYCOM: GOFS 3.1: 41-layer HYCOM + NCODA Global 1/12° Reanalysis, HYCOM [data set], https://www.hycom.org/data/glbv0pt08/expt-53ptx, last access: 17 March 2024. a
HYCOM Consortium: Global Ocean Forecasting System (GOFS) 3.1 output on the GLBv0.08 grid, https://www.hycom.org/dataserver/gofs-3pt1/reanalysis (last access: 17 March 2024), 2019. a
IPCC: Climate Change 2022: Impacts, Adaptation and Vulnerability. Contribution of Working Group II to the Sixth Assessment Report of the Intergovernmental Panel on Climate Change, edited by: Pörtner, H.-O., Roberts, D. C., Tignor, M., Poloczanska, E. S., Mintenbeck, K. Alegría, A., Craig, M., Langsdorf, S., Löschke, S., Möller, V., Okem, A., and Rama, B., Cambridge University Press, Cambridge University Press, Cambridge, UK and New York, NY, USA, 3056 pp., https://doi.org/10.1017/9781009325844, 2022. a
Iyer, S. and Drushka, K.: The influence of preexisting stratification and tropical rain modes on the mixed layer salinity response to rainfall, J. Geophys. Res.-Oceans, 126, e2021JC017574, https://doi.org/10.1029/2021JC017574, 2021. a
Jourdain, N. C., Lengaigne, M., Vialard, J., Madec, G., Menkès, C. E., Vincent, E. M., Jullien, S., and Barnier, B.: Observation-based estimates of surface cooling inhibition by heavy rainfall under tropical cyclones, J. Phys. Oceanogr., 43, 205–221, 2013. a
Karnauskas, K. B., Zhang, L., and Emanuel, K. A.: The feedback of cold wakes on tropical cyclones, Geophys. Res. Lett., 48, e2020GL091676, https://doi.org/10.1029/2020GL091676, 2021. a, b
Kishtawal, C., Jaiswal, N., Singh, R., and Niyogi, D.: Tropical cyclone intensification trends during satellite era (1986–2010), Geophys. Res. Lett., 39, 10, https://doi.org/10.1029/2012GL051700, 2012. a
Klotzbach, P. J., Bowen, S. G., Pielke, R., and Bell, M.: Continental US hurricane landfall frequency and associated damage: Observations and future risks, B. Am. Meteorol. Soc., 99, 1359–1376, 2018. a
Klotzbach, P. J., Wood, K. M., Schreck III, C. J., Bowen, S. G., Patricola, C. M., and Bell, M. M.: Trends in global tropical cyclone activity: 1990–2021, Geophys. Res. Lett., 49, e2021GL095774, https://doi.org/10.1029/2021GL095774, 2022. a
Korty, R. L., Emanuel, K. A., and Scott, J. R.: Tropical cyclone–induced upper-ocean mixing and climate: Application to equable climates, J. Climate, 21, 638–654, 2008. a
Kuang, C., Lee, J. H., Harrison, P. J., and Yin, K.: Effect of wind speed and direction on summer tidal circulation and vertical mixing in Hong Kong waters, J. Coastal Res., 27, 74–86, 2011. a
Kuusela, M. and Stein, M. L.: Locally stationary spatio-temporal interpolation of Argo profiling float data, P. Roy. Soc. A-Math. Phy., 474, 20180400, https://doi.org/10.1098/rspa.2018.0400, 2018. a
Landsea, C. W. and Franklin, J. L.: Atlantic hurricane database uncertainty and presentation of a new database format, Mon. Weather Rev., 141, 3576–3592, 2013 (data available at: https://www.nhc.noaa.gov/data/#hurdat, last access: 17 May 2024). a, b
Large, W. G., McWilliams, J. C., and Doney, S. C.: Oceanic vertical mixing: A review and a model with a nonlocal boundary layer parameterization, Rev. Geophys., 32, 363–403, 1994. a
Leipper, D. F.: Observed ocean conditions and Hurricane Hilda, 1964, J. Atmos. Sci., 24, 182–186, 1967. a
Lin, I.-I., Wu, C.-C., Emanuel, K. A., Lee, I.-H., Wu, C.-R., and Pun, I.-F.: The interaction of Supertyphoon Maemi (2003) with a warm ocean eddy, Mon. Weather Rev., 133, 2635–2649, 2005. a
Lin, S., Zhang, W., Shang, S., and Hong, H.: Ocean response to typhoons in the western North Pacific: Composite results from Argo data, Deep-Sea Res. Pt. I, 123, 62–74, 2017. a, b, c
Liu, F., Zhang, H., Ming, J., Zheng, J., Tian, D., and Chen, D.: Importance of precipitation on the upper ocean salinity response to typhoon kalmaegi (2014), Water, 12, 614, https://doi.org/10.3390/w12020614, 2020. a, b
Liu, Z., Xu, J., Zhu, B., Sun, C., and Zhang, L.: The upper ocean response to tropical cyclones in the northwestern Pacific analyzed with Argo data, Chin. J. Oceanol. Limn., 25, 123–131, 2007. a, b
Lloyd, I. D. and Vecchi, G. A.: Observational evidence for oceanic controls on hurricane intensity, J. Climate, 24, 1138–1153, 2011. a
McDougall, T. J. and Barker, P. M.: Getting started with TEOS-10 and the Gibbs Seawater (GSW) oceanographic toolbox, Scor/Iapso WG, 127, 1–28, ISBN 978-0-64655621-5, 2011. a, b
McPhaden, M. J., Foltz, G. R., Lee, T., Murty, V., Ravichandran, M., Vecchi, G. A., Vialard, J., Wiggert, J. D., and Yu, L.: Ocean-atmosphere interactions during cyclone nargis, EOS T. Am. Geophys. Un., 90, 53–54, 2009. a
Mendelsohn, R., Emanuel, K. A., Chonabayashi, S., and Bakkensen, L.: The impact of climate change on global tropical cyclone damage, Nat. Clim. Change, 2, 205–209, 2012. a
Meng, Q., Li, P., Zhai, F., and Gu, Y.: The vertical mixing induced by winds and tides over the Yellow Sea in summer: a numerical study in 2012, Ocean Dynam., 70, 847–861, 2020. a
Neetu, S., Lengaigne, M., Vincent, E. M., Vialard, J., Madec, G., Samson, G., Ramesh Kumar, M., and Durand, F.: Influence of upper-ocean stratification on tropical cyclone-induced surface cooling in the Bay of Bengal, J. Geophys. Res.-Oceans, 117, C12020, https://doi.org/10.1029/2012JC008433, 2012. a
Nychka, D. W.: Spatial-process estimates as smoothers, in: Smoothing and regression: approaches, computation, and application, edited by: Schimek, M. G., Wiley, https://doi.org/10.1002/9781118150658.ch13, 2000. a
Orr, D. W. and Ogden, J. C.: The impact of Hurricane Andrew on the ecosystems of South Florida, Conserv. Biol., 6, 488–490, 1992. a
Prasad, T. and Hogan, P. J.: Upper-ocean response to Hurricane Ivan in a 1/25 nested Gulf of Mexico HYCOM, J. Geophys. Res.-Oceans, 112, C04013, https://doi.org/10.1029/2006JC003695, 2007. a, b, c
Price, J. F.: Upper ocean response to a hurricane, J. Phys. Oceanogr., 11, 153–175, 1981. a, b, c
Reul, N., Fournier, S., Boutin, J., Hernandez, O., Maes, C., Chapron, B., Alory, G., Quilfen, Y., Tenerelli, J., Morisset, S., Kerr, Y., Mecklenburg, S., and Delwart, S.: Sea surface salinity observations from space with the SMOS satellite: A new means to monitor the marine branch of the water cycle, Surv. Geophys., 35, 681–722, 2014a. a
Reul, N., Quilfen, Y., Chapron, B., Fournier, S., Kudryavtsev, V., and Sabia, R.: Multisensor observations of the Amazon-Orinoco river plume interactions with hurricanes, J. Geophys. Res.-Oceans, 119, 8271–8295, 2014b. a, b, c
Reul, N., Chapron, B., Grodsky, S. A., Guimbard, S., Kudryavtsev, V., Foltz, G. R., and Balaguru, K.: Satellite observations of the sea surface salinity response to tropical cyclones, Geophys. Res. Lett., 48, e2020GL091478, https://doi.org/10.1029/2020GL091478, 2021. a, b, c, d, e
Roemmich, D. H. and Gilson, J.: The 2004–2008 mean and annual cycle of temperature, salinity, and steric height in the global ocean from the Argo Program, Prog. Oceanogr., 82, 81–100, 2009. a
Roemmich, D. H., Davis, R. E., Riser, S. C., Owens, W. B., Molinari, R. L., Garzoli, S. L., and Johnson, G. C.: The Argo project. Global ocean observations for understanding and prediction of climate variability, Tech. rep., Scripps Institution Of Oceanography La Jolla Ca, https://apps.dtic.mil/sti/pdfs/ADA496094.pdf (last access: 18 October 2024), 2003. a, b
Roemmich, D. H., Johnson, G. C., Riser, S., Davis, R., Gilson, J., Owens, W. B., Garzoli, S. L., Schmid, C., and Ignaszewski, M.: The Argo Program: Observing the global ocean with profiling floats, Oceanography, 22, 34–43, 2009. a, b, c
Saha, S., Moorthi, S., Wu, X., Wang, J., Nadiga, S., Tripp, P., Behringer, D., Hou, Y.-T., Chuang, H.-y., Iredell, M., Ek, M., Meng, J., Yang, R., Peña Mendez, M., van den Dool, H., Zhang, Q., Wang, W., Chen, M., and Becker, E.: The NCEP climate forecast system version 2, J. Climate, 27, 2185–2208, 2014. a
Samson, G., Masson, S., Lengaigne, M., Keerthi, M. G., Vialard, J., Pous, S., Madec, G., Jourdain, N. C., Jullien, S., Menkes, C., and Marchesiello, P.: The NOW regional coupled model: Application to the tropical Indian Ocean climate and tropical cyclone activity, J. Adv. Model. Earth Sy., 6, 700–722, 2014. a
Sanford, T. B., Black, P. G., Haustein, J. R., Feeney, J. W., Forristall, G. Z., and Price, J. F.: Ocean response to a hurricane. Part I: Observations, J. Phys. Oceanogr., 17, 2065–2083, 1987. a, b
Schade, L. R. and Emanuel, K. A.: The ocean's effect on the intensity of tropical cyclones: Results from a simple coupled atmosphere–ocean model, J. Atmos. Sci., 56, 642–651, 1999. a
Shay, L. K., Goni, G. J., and Black, P. G.: Effects of a warm oceanic feature on Hurricane Opal, Mon. Weather Rev., 128, 1366–1383, 2000. a
Shen, W. and Ginis, I.: Effects of surface heat flux-induced sea surface temperature changes on tropical cyclone intensity, Geophys. Res. Lett., 30, 1933, https://doi.org/10.1029/2003GL017878, 2003. a
Steffen, J. and Bourassa, M.: Barrier layer development local to tropical cyclones based on Argo float observations, J. Phys. Oceanogr., 48, 1951–1968, 2018. a, b, c
Sun, J., Vecchi, G., and Soden, B.: Sea surface salinity response to tropical cyclones based on satellite observations, Remote Sens.-Basel, 13, 420, https://doi.org/10.3390/rs13030420, 2021. a, b
Tucker, T., Giglio, D., Scanderbeg, M., and Shen, S. S. P.: Argovis: A Web Application for Fast Delivery, Visualization, and Analysis of Argo Data, J. Atmos. Ocean. Tech., 37, 401–416, https://doi.org/10.1175/JTECH-D-19-0041.1, 2020 (data available at: https://argovis.colorado.edu, last access: 23 August 2024). a
Venkatesan, R., Mathew, S., Vimala, J., Latha, G., Muthiah, M. A., Ramasundaram, S., Sundar, R., Lavanya, R., and Atmanand, M. A.: Signatures of very severe cyclonic storm Phailin in met–ocean parameters observed by moored buoy network in the Bay of Bengal, Curr. Sci. India, 107, 589–595, 2014. a, b
Vinayachandran, P. N. and Mathew, S.: Phytoplankton bloom in the Bay of Bengal during the northeast monsoon and its intensification by cyclones, Geophys. Res. Lett., 30, 1572, https://doi.org/10.1029/2002GL016717, 2003. a, b
Vincent, E. M., Emanuel, K. A., Lengaigne, M., Vialard, J., and Madec, G.: Influence of upper ocean stratification interannual variability on tropical cyclones, J. Adv. Model. Earth Sy., 6, 680–699, 2014. a
Wahba, G.: Spline bases, regularization, and generalized cross validation for solving approximation problems with large quantities of noisy data, University of Wisconsin, https://pages.stat.wisc.edu/~wahba/TRarchive/TRsEARLY2/tr597_1.pdf (last access: 18 October 2024), 1980. a
Wahba, G.: Spline models for observational data, SIAM, https://epubs.siam.org/doi/10.1137/1.9781611970128 (last access: 18 October 2024), 1990. a
Wang, G., Wu, L., Johnson, N. C., and Ling, Z.: Observed three-dimensional structure of ocean cooling induced by Pacific tropical cyclones, Geophys. Res. Lett., 43, 7632–7638, 2016. a
Wang, X., Han, G., Qi, Y., and Li, W.: Impact of barrier layer on typhoon-induced sea surface cooling, Dynam. Atmos. Oceans, 52, 367–385, https://doi.org/10.1016/j.dynatmoce.2011.05.002, 2011. a, b
Webb, D. and Suginohara, N.: Vertical mixing in the ocean, Nature, 409, 37–37, 2001. a
Wood, S. N.: Generalized additive models: an introduction with R, CRC Press, https://doi.org/10.1201/9781315370279, 2017. a
Zamudio, L. and Hogan, P. J.: Nesting the Gulf of Mexico in Atlantic HYCOM: oceanographic processes generated by Hurricane Ivan, Ocean Model., 21, 106–125, 2008. a
Zhang, H., Chen, D., Zhou, L., Liu, X., Ding, T., and Zhou, B.: Upper ocean response to typhoon Kalmaegi (2014), J. Geophys. Res.-Oceans, 121, 6520–6535, 2016. a, b
Zhang, H., He, H., Zhang, W.-Z., and Tian, D.: Upper ocean response to tropical cyclones: A review, Geoscience Letters, 8, 1–12, 2021. a, b
Zhang, Z., Wu, H., Yin, X., and Qiao, F.: Dynamical response of Changjiang River plume to a severe typhoon with the surface wave-induced mixing, J. Geophys. Res.-Oceans, 123, 9369–9388, 2018. a, b
Zhang, Z., Ma, Z., Fei, J., Zheng, Y., and Huang, J.: The effects of tropical cyclones on characteristics of barrier layer thickness, Front. Earth Sci., 10, 1287, https://doi.org/10.3389/feart.2022.962232, 2022. a
Zhu, T. and Zhang, D.-L.: The impact of the storm-induced SST cooling on hurricane intensity, Adv. Atmos. Sci., 23, 14–22, 2006. a