the Creative Commons Attribution 4.0 License.
the Creative Commons Attribution 4.0 License.
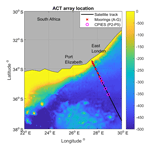
Investigating the relationship between volume transport and sea surface height in a numerical ocean model
Björn Backeberg
Juliet Hermes
Shane Elipot
The Agulhas Current Time-series Experiment mooring array (ACT) measured transport of the Agulhas Current at 34∘ S for a period of 3 years. Using along-track satellite altimetry data directly above the array, a proxy of Agulhas Current transport was developed based on the relationship between cross-current sea surface height (SSH) gradients and the measured transports. In this study, the robustness of the proxy is tested within a numerical modelling framework using a 34-year-long regional hindcast simulation from the Hybrid Coordinate Ocean Model (HYCOM). The model specifically tested the sensitivity of the transport proxy to (1) changes in the vertical structure of the current and to (2) different sampling periods used to calculate the proxy. Two reference proxies were created using HYCOM data from 2010 to 2013 by extracting model data at the mooring positions and along the satellite altimeter track for the box (net) transport and the jet (southwestward) transport. Sensitivity tests were performed where the proxy was recalculated from HYCOM for (1) a period where the modelled vertical stratification was different compared to the reference proxy and (2) different lengths of time periods: 1, 3, 6, 12, 18, and 34 years. Compared to the simulated (native) transports, it was found that the HYCOM proxy was more capable of estimating the box transport of the Agulhas Current compared to the jet transport. This was because the model is unable to resolve the dynamics associated with meander events, for which the jet transport algorithm was developed. The HYCOM configuration in this study contained exaggerated levels of offshore variability in the form of frequently impinging baroclinic anticyclonic eddies. These eddies consequently broke down the linear relationship between SSH slope and vertically integrated transport. Lastly, results showed that calculating the proxy over shorter or longer time periods in the model did not significantly impact the skill of the Agulhas transport proxy. Modelling studies of this kind provide useful information towards advancing our understanding of the sensitivities and limitations of transport proxies that are needed to improve long-term ocean monitoring approaches.
- Article
(2903 KB) - Full-text XML
- BibTeX
- EndNote
The Agulhas Current system is the strongest western boundary current in the Southern Hemisphere and transports warm tropical water southward along the east coast of South Africa (Lutjeharms, 2006). The Agulhas Current, in the northern region, is known for its narrow, fast flow conditions following the steep continental slope (de Ruijter et al., 1999). As the current continues southwestward it becomes increasingly unstable over the widening continental shelf until it eventually retroflects, forming an anticyclonic loop south of Africa and returning to the Indian Ocean as the eastward Agulhas return current (Beal et al., 2011; Biastoch and Krauss, 1999; Dijkstra and de Ruijter, 2001; Hermes et al., 2007; Lutjeharms, 2006; Loveday et al., 2014). The anticyclonic loop, known as the Agulhas retroflection, contains some of the highest levels of mesoscale variability in the global ocean (Gordon, 2003) in the form of Agulhas rings, eddies and filaments. These contribute heat, salt and energy into the Benguela upwelling system, the Atlantic Ocean and the global overturning circulation system (Gordon et al., 1987; Beal et al., 2011; Durgadoo et al., 2013), impacting the Atlantic Meridional Overturning Circulation (AMOC) (Biastoch and Krauss, 1999; Beal et al., 2011; Durgadoo et al., 2013; Loveday et al., 2014). In the regional context, the Agulhas Current has a major influence on the local weather systems, due to large latent and sensible heat fluxes, which contributes to rainfall and storm events over the adjacent land (Reason, 2001; Rouault et al., 2002; Rouault and Lutjeharms, 2003). The unique circulation of the Agulhas Current system, in the context of regional and global climate variability, makes it an important field of research.
To understand the complicated dynamics of the Agulhas Current requires an integrated approach using numerical ocean models, satellite remote sensing measurements, and in situ observations. Previous studies have suggested that measuring the dynamics of the Agulhas Current in the northern region is easier due to its stable trajectory and its confinement to the continental slope (van Sebille et al., 2010). However, the close proximity of the current to the coast has made it difficult to monitor using satellite altimetry (Rouault et al., 2010). Newer altimetry products dedicated to coastal areas are promising but are yet to be validated within the Agulhas Current region (Birol et al., 2017). In addition, the frequent disturbances of the current in the form of solitary meanders, also known as the Natal Pulse, and its interactions with mesoscale features originating upstream and from the east (Elipot and Beal, 2015) remain poorly resolved in many numerical ocean models (Tsugawa and Hasumi, 2010; Braby et al., 2016), highlighting the challenges involved in monitoring and modelling the dynamics in this region.
There is a trade-off between spatial and temporal sampling. In situ mooring observations provide high temporal observations of the Agulhas Current throughout the water column but are spatially coarse. In contrast, satellite observations can provide high spatial resolution data of the surface ocean but lacks detailed information below the surface. Hence, numerical models are needed to provide a temporally coherent, high-resolution representation of the ocean throughout the water column. Numerous studies aiming to monitor long-term changes in global current systems have adopted methods to combine various sampling tools (Maul et al., 1990; Imawaki et al., 2001; Andres et al., 2008; Zhu et al., 2004; Yan and Sun, 2015), including the recent development of the Agulhas transport proxy established to monitor the interannual variability and long-term trends in Agulhas Current transport (Beal and Elipot, 2016).
Beal and Elipot (2016) have shown that a strong relationship exists between surface geostrophic velocity and full-depth transport such that sea level anomalies can be used to study the variability and dynamics of the Agulhas Current system as has been demonstrated before (Fu et al., 2010; Rouault et al., 2010; Rouault and Penven, 2011; etc.). The 22-year transport proxy created by Beal and Elipot (2016) assumed a fixed linear relationship between in situ transport and sea surface slope based on in situ measurements over the 3-year sampling period of the Agulhas Current Time-series Experiment (ACT) (Beal et al., 2015). Analyses of the Agulhas Current transport proxy time series concluded that the Agulhas Current has not intensified over the last 2 decades in response to intensified global winds under anthropogenic climate change (Cai, 2006; Yang et al., 2016), but instead has broadened as a result of increased eddy activity (Beal and Elipot, 2016) in agreement with Backeberg et al. (2012). This could essentially decrease poleward heat transport and increase mixing over the continental shelf, thereby increasing cross-frontal exchange of nutrients and pollutants between the coastal ocean and the deep ocean (Backeberg et al., 2012; Beal and Elipot, 2016).
This modelling study recreates the Agulhas transport proxy developed by Beal and Elipot (2016), within a regional HYCOM simulation of the greater Agulhas Current system, aiming to test the sensitivity of using 3 years of in situ mooring data to develop a transport proxy as well as the sensitivity of the proxy to changes in the vertical structure of the Agulhas Current. The paper is structured as follows. Section 2 describes the data and methods; it should be noted that this section forms a key part of the paper as the methods of recreating the proxy are an integral component of the study. Section 3 presents the results from the HYCOM transport proxy and lastly Sect. 4 presents the summary and conclusions.
2.1 The Hybrid Coordinate Ocean Model
The Hybrid Coordinate Ocean Model (HYCOM) is a primitive equation ocean model that was developed from the Miami Isopycnic Coordinate Ocean Model (MICOM) (Smith et al., 1990). HYCOM combines the optimal features of isopycnic coordinates and fixed-grid ocean circulation models into one framework (Bleck, 2002) and uses the hybrid layers to change the vertical coordinates depending on the stratification of the water column. The model makes a dynamically smooth transition between the vertical coordinate types via the continuity equation using the hybrid coordinate generator (Chassignet et al., 2007). Well-mixed surface layers use z-level coordinates, ρ coordinates are utilized between the surface and bottom layers in a well-stratified ocean, and the bottom layers apply σ coordinates following bottom topography. Adjusting the vertical spacing between the hybrid coordinate layers in HYCOM simplifies the numerical implementation of several physical processes without affecting the efficient vertical resolution, and thus combines the advantages of the different coordinate types in optimally simulating coastal and open-ocean circulation features (Chassignet et al., 2007).
This study used output from a one-way nested 1∕10∘ model of the greater Agulhas Current system (AGULHAS) (Backeberg et al., 2008, 2009, 2014). The regional nested model, AGULHAS, received boundary conditions from the basin-scale model of the Indian Ocean and Southern Ocean (INDIA) (George et al., 2010) every 6 h. The boundary conditions were relaxed towards the outer model over a 20 grid cell sponge layer. The nested model covered the region from the Mozambique Channel to the Agulhas retroflection region and the Agulhas return current, geographically extending from approximately 0 to 60∘ E and from 10 to 50∘ S, with a horizontal resolution of ∼10 km that adequately resolved mesoscale dynamics to the order of the first baroclinic Rossby radius estimated to be about 30 km (Chelton et al., 1998). AGULHAS has 30 hybrid layers and targeted densities ranging from 23.6 to 27.6 kg m−3.
AGULHAS was initialized from a balanced field of the parent model interpolated to the high-resolution grid and ran from 1980 to 2014 using interannual forcing from ERA40 (Uppala et al., 2005) and ERA-Interim (Dee et al., 2011). Version 2.2 of the HYCOM source code has been used in this model and, together with the second-order advection scheme, provides an adequate representation of the Agulhas Current (Backeberg et al., 2014). However, limitations of the free-running model include high levels of sea surface height (SSH) variability south of Madagascar and offshore of the Agulhas Current, suggesting that eddy trajectories may be too regular in the model (Backeberg et al., 2014). The data available for this study were a weekly output of the regional HYCOM simulation of the Agulhas region from 1980 to 2014.
2.2 The Agulhas Current Time-series Experiment
The ACT was established to obtain a multi-decadal proxy of Agulhas Current transport using satellite altimeter data. The first phase of the experiment was the in situ phase where the ACT mooring array was deployed in the Agulhas Current, near 34∘ S, for a period of 3 years from 2010 to 2013 (Beal et al., 2015) (Fig. 1). From the data collected, Beal et al. (2015) provided two volume transport estimates: (1) a box or boundary layer transport (Tbox) and (2) a western boundary jet transport (Tjet). Tbox is the net transport within a fixed distance from the coast, while Tjet is a stream-dependent transport that is calculated by changing the boundaries of integration at each time step depending on the strength and cross-sectional area of the southwestward jet. The western boundary jet transport algorithm was developed to specifically exclude the northeastward transport during meander events, occurring inshore of the meander (Beal et al., 2015). During the second phase of the ACT, Beal and Elipot (2016) built a 22-year transport proxy by regressing the 3 years of in situ transport measurements (obtained from phase 1 against along-track satellite altimeter data spanning the years 1993–2015.
2.3 Development of the Agulhas transport proxy
Previous analyses have shown that the vertical structure of the Agulhas Current is barotropic (Elipot and Beal, 2015), implying that the relationship between surface geostrophic velocity and full depth transport should be strong, despite the presence of the Agulhas Undercurrent (Beal and Elipot, 2016) (Fig. 2). Access to the data from the ACT allowed us to validate the velocity cross section in HYCOM (Fig. 2). Beal et al. (2015) defined the Agulhas Current to be 219 km wide and 3000 m deep on average, as is reflected in the vertical section of the in situ ACT observations (Fig. 2a). In HYCOM the current is wider, weaker, and further offshore than the observed current, on average the current is 254 km wide and extends deeper down to ∼3500 m, particularly inshore with a less pronounced undercurrent (Fig. 2b).
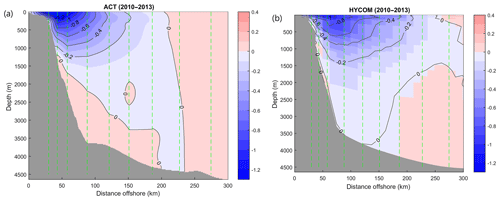
Figure 2Time mean cross section of the velocity structure of the Agulhas Current across the ACT array (m s−1) during the in situ ACT period (2010–2013) from (a) the ACT observations and (b) the HYCOM. Blue shading represents the negative, southwest current flow and pink shading represents the positive, northeast current flow. Contours are every 0.2 m s−1. Dashed green vertical lines represent the nine locations of the mooring and CPIES pairs, the first line representing mooring A and CPIES pair P4P5 furthest offshore.
The transport proxy created by Beal and Elipot (2016) was initially developed by finding a linear relationship between transport and sea surface slope across the entire length of the ACT array, a common method used in previous studies (Imawaki et al., 2001; van Sebille et al., 2010; Sprintall and Revelard, 2014; Yan and Sun, 2015). However, this method led to uncertainty in the linear regression due to the strong, co-varying sea surface height across the current. The preferred method was therefore to build nine individual linear regression models, one for each mooring position and CPIES pairs along the ACT array, which locally related transport to sea surface slope (Beal and Elipot, 2016). It is important to note that the regression models assumed a constant, linear relationship between sea surface slope and transport over the 3-year in situ period. The transport variable in the regression models was defined as transport per unit distance, i.e. the vertically integrated velocity with units in square metre per second (m2 s−1), where Tx represents the net component of the current flow and Txsw the southwestward component of the flow. The total transports, Tbox and Tjet in cubic metre per second (m3 s−1), were calculated by integrating the Tx and Txsw estimates, predicted from the regression models, to the respective current boundaries.
2.4 Recreating the Agulhas transport proxy in HYCOM
In order to recreate the Agulhas Current proxy in HYCOM, data corresponding to the measurements collected from the ACT mooring array were extracted from the model. To build the regression models, the transport per unit distance and sea surface slope for each of the nine mooring locations were calculated using the model data (hereafter CPIES pairs P3–P4 and P4–P5 were included as mooring positions 8 and 9).
2.4.1 Model transport
The barotropic velocity – equivalent to an integral of the velocity with depth – from each mooring location (A–G) and CPIES pairs P3–P4 and P4–P5 (Beal et al., 2015) was extracted for the 34-year model period. Extracting the barotropic velocity component from each mooring avoided interpolation errors that may have occurred if the model velocity was interpolated onto the locations of each current-meter instrument on each mooring (e.g. van Sebille et al., 2010). Transport per unit distance (Tx) for each mooring was calculated by multiplying the cross-track barotropic velocity by the respective depth at each mooring location. The same method was employed to calculate the southwestward transport component (Txsw) excluding the northeast cross-track barotropic velocity values in the calculation.
2.4.2 Model SSH
In order to reproduce the “along-track” SSH altimeter data needed to create the proxy as in Beal and Elipot (2016), 34 years of HYCOM SSH data was linearly interpolated onto the coordinates of the TOPEX/Jason satellite track number 96 overlapping the model ACT array. The coordinates of the along-track altimeter data were obtained from the filtered 12 km Jason-2 Aviso satellite product. To obtain the sea surface slope for each regression model, an optimal pair of SSH data points was chosen such that the horizontal length scale between them allowed for a maximum correlation between sea surface slope and Tx. The length scales of the slopes ranged from 24 km at mooring A to 12 km at mooring G, and 48 km for the offshore CPIES pairs, indicating an increase in the spatial scale of offshore flow, possibly due to increased offshore variability. Results from the in situ proxy experiment by Beal and Elipot (2016) also showed an increasing length scale with increasing distance offshore; however, the results varied in magnitude: 27 km at mooring B to 102 km at mooring G. In this study the SSH slope was calculated such that a negative SSH slope corresponds to a negative surface velocity (southwestward) according to geostrophy, whereas a positive slope would indicate positive northeastward flow.
2.4.3 Building the regression models
Nine linear regression models were developed to estimate the transport per unit distance (Tx and Txsw) from the HYCOM sea surface slope during the same 3-year period over which the ACT proxy was developed (April 2010–February 2013). The 3-year time period is hitherto referred to as the reference period.
To calculate the total transport across the ACT array required continuous Tx estimates across the current. This was achieved as in Beal and Elipot (2016) by fitting a piecewise cubic Hermite interpolating polynomial function to obtain transport estimates at 1 km intervals from the coast to the end of the array (Fig. 3). Fitting the transport function to the coast and equating it to zero would be equivalent to implementing a no-slip boundary condition in the model. Before calculating the total transport the current boundaries needed to be defined. The box transport (Tbox) was calculated by integrating Tx horizontally to 230 km offshore, the 3-year mean width of the current in HYCOM. The jet transport (Tjet) was calculated using the algorithm developed by Beal et al. (2015) by integrating Txsw, the southwestward transport component, to the first maximum of Tx beyond the half-width of the current (115 km in HYCOM) at each time step (Fig. 3).
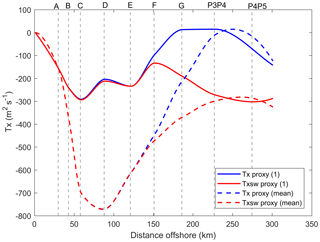
Figure 3HYCOM transport per unit distance proxy (m2 s−1) for Tx (blue) and Txsw (red) at 1 km intervals at the first model time step (solid lines) and for the ACT reference period (2010–2013, dashed lines). The dashed grey lines represent the positions of moorings and offshore CPIES pairs.
Assuming that the 3-year linear relationship between SSH slope and transport per unit distance (Tx and Txsw) from 2010–2013 remains constant, the regression models were applied to the entire 34-year SSH model data. Thereafter, the 34-year transports were calculated by applying the same methods that were used to calculate the 3-year transport time series; firstly, obtaining Tx and Txsw estimates at 1 km intervals along the array and secondly integrating horizontally to obtain Tbox and Tjet.
2.5 Comparison of the transport proxy to actual model transports
The simulated model transports were calculated using the full-depth velocity fields across the array. If the relationship between SSH slope and transport is strong, there would be good agreement between the proxy and the actual model transports. To quantify this, correlations and transport statistics for the model and proxy were calculated from the two time series (Table 2). These provided insight into which processes the proxy may have failed to capture, which were then further investigated in HYCOM. Statistics are deemed significant at the 95 % significance level.
Eddy kinetic energy (EKE) was calculated to show the surface variability of the current coincident with averaged SSH contours used to represent the mean surface structure (Fig. 6). EKE was calculated over the 3-year mean reference period, and over the highest and lowest correlated years. In order to evaluate the subsurface current structure along the ACT array, vertical velocity profiles were analysed for each mooring and CPIES pair over the 3-year mean reference period as well as over the highest and lowest correlated years.
Transport variability in HYCOM was analysed by investigating the current structure during the residual transport events in the least- and best-performing regression models. Residual transport events were identified as the outlying residual transport values above and below 2 standard deviations of the estimated transport.
where e is the estimated residuals, Txi is the HYCOM transport per unit distance value and is the estimated transport per unit distance value according to the linear regression models (i.e. the transport proxy).
To investigate the current structure during these residual events, composite averages of the cross-track velocity structure were analysed. The cross-track velocity at each depth layer in HYCOM was extracted at 12 km intervals from 0 to 400 km offshore for the 34-year model period. Although the ACT array only reached 300 km offshore, analysis of the current structure in HYCOM was extended further offshore. Previous analyses have shown increased levels of offshore variability in this HYCOM simulation (Backeberg et al., 2009, 2014), which therefore made it interesting to study the subsurface structure during the offshore current meanders and the influence these could have on the transport proxy. To further investigate the effect of the residual transport values on the transport proxy, all corresponding transport events exceeding plus or minus 2 standard deviations were removed from each linear regression model during development of the proxy (Fig. 4).
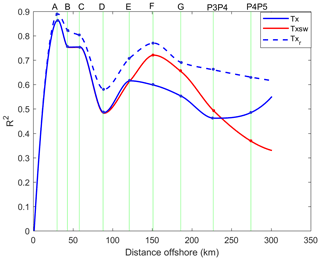
Figure 4R2 statistics from the linear regression models showing the relationship between HYCOM SSH slope and HYCOM transport per unit distance for each mooring (A–G) and CPIES pair (P3P4 and P4P5) over the 3-year reference period (2010–2013). Tx is represented by the solid blue line and Txsw by the solid red line. The dashed blue line represents the results of Tx after the removal of the residual transport events (see Sect. 3.4). Sites A to CPIES pair P4P5 are shown by the faint green lines.
2.6 Sensitivity tests
Sensitivity experiments were performed in HYCOM to test how many years of mooring data is needed to create an accurate proxy of Agulhas Current transport. With 34 years of model data the linear relationship could be tested over much longer or shorter periods.
Using the method described in Sect. 2.4.3, the proxy regression models were built using 1, 6, 12, 18, and 34 years of HYCOM data. In addition, the proxies were calculated over two arbitrary 3-year periods to test the sensitivity of the proxy to current dynamics over different years. Lastly, the regression models were calculated over the maximum and minimum annual transport years in HYCOM, as well as during the years the HYCOM transport standard deviation was the largest and the smallest. Table 1 shows the time range over which the sensitivity experiments were performed.
3.1 HYCOM linear regression models
The coefficients of determination (R2) from the regression models highlight how well the linear relationship predicts the transport in HYCOM (Fig. 4). R2 ranged from 0.86 at mooring A (30 km offshore) to 0.49 at the last CPIES pair P4P5 (275 km offshore) for Tx and 0.86 at mooring A to 0.37 at P4P5 for Txsw (P values ). Results from Beal and Elipot (2016) showed an increase in the R2 statistics in the regression models ranging from 0.51 at mooring A and 0.81 for CPIES pair P4P5 for Tx, indicating that the in situ observation-based regression models had poorer skill inshore, whereas in HYCOM the regression models have poorer skill offshore. The results from the Txsw regression models in HYCOM showed similar results to Beal and Elipot (2016) for the inshore mooring locations (A, B, C, E) with slightly higher correlations for offshore moorings F, G, and CPIES pair P3P4 but a lower correlation for D and the furthest CPIES pair P4P5.
3.2 Proxy validation
Two transport types, the box transport (Tbox) and the jet transport (Tjet), were extracted from HYCOM in order to validate the relative proxies. The Tbox (Tjet) proxy explained 57 % (14 %) of transport variance during the 3-year reference period (2010–2013) (Table 2b). Using 34 years of model data (1980–2014), assuming the fixed 3-year relationship between SSH slope and transport, Tbox (Tjet) explained 52 % (26 %) of the transport variance (Table 2b). Results from Beal and Elipot (2016) also showed that Tbox explained a higher percentage of variance (61 %) during the ACT period than the jet transport proxy (Tjet: 55 %).
Table 2(a) Summary of the transport statistics of the ACT observations over the 3-year in situ period and the HYCOM model transports and HYCOM proxy transports over the 3-year and extended 34-year time periods. Negative values denote transport in the southwest direction. 1 Sv =106 m3 s−1 (sverdrup). (b) Correlations between the HYCOM model transport and HYCOM proxy transport for the box transport and jet transport with the percentage of variance shown in brackets. All correlations were significant.
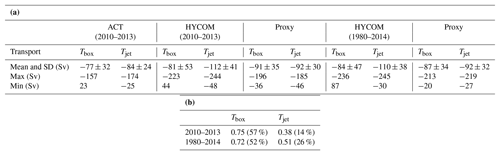
The 34-year mean transport and standard deviation from HYCOM for Tbox and Tjet were Sv and Sv, respectively (Table 2a). The proxy Tbox and Tjet were Sv and Sv, respectively (Table 2a). According to the ACT observations, the mean transport and standard deviation were Sv for Tbox and Sv for Tjet. A higher jet transport was expected considering it excludes northeast counterflows that decrease the box transport (Beal et al., 2015). The differences between the standard deviations of HYCOM and the proxy indicate that transport in HYCOM experiences more variability compared to the proxy. The proxies only capture a portion of the transport estimate from HYCOM, suggesting it also only captures a portion of the model variability. The positive minimum transport values for Tbox during both time periods also appear to be peculiar, suggesting a current reversal during those events (Table 2a).
The Tjet annual correlation varies greatly from year to year with a significant maximum correlation of 0.82 (2014) and a minimum correlation of 0.00 (2003) (Fig. 5). In contrast, the correlations for Tbox vary much less and are always significant with a maximum correlation of 0.88 (1988) and minimum correlation of 0.50 (1994) (Fig. 5). The box transport has higher correlations for most of the 34-year time period except during two single years where the jet transport has a higher correlation, 0.78 versus 0.70 during 1991 and 0.54 versus 0.50 during 1994. These results indicate that the proxy is generally better suited in HYCOM to estimate the box transport rather than the jet transport.
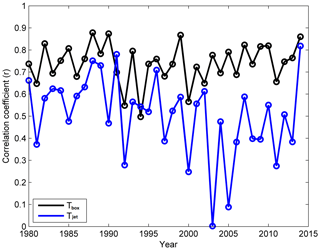
Figure 5This 34-year annual correlations between the box (black) and jet (blue) transport proxies against the box and jet transports extracted from HYCOM.
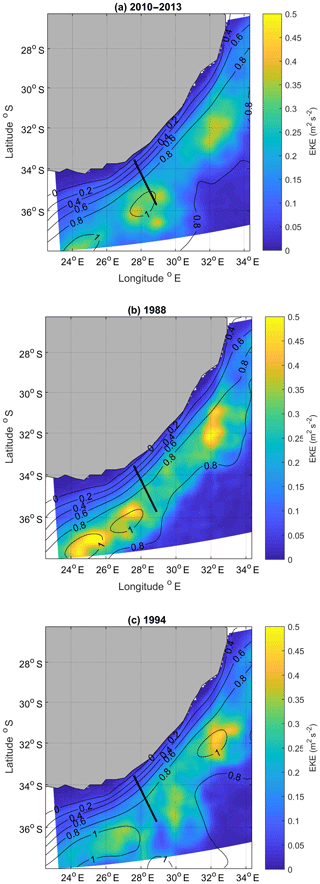
Figure 6Eddy kinetic energy (EKE in m2 s−2) and sea surface height (SSH in metres) contours during (a) the reference period (2010–2013) (b) the highest (1988), and (c) lowest (1994) correlated years. The black line representing the ACT array.
The jet transport proxy by Beal and Elipot (2016) was developed to estimate the transport of the Agulhas Current during mesoscale meander events, which generally causes the current to manifest as a full-depth, surface intensified, cyclonic circulation out to 150 km from the coast with anticyclonic circulation farther offshore (Elipot and Beal, 2015). The Agulhas meanders in the HYCOM simulation occur in association with large anticyclonic eddies predominantly located at the offshore edge of the current, with a narrow, southwest stream close to the coast (Backeberg et al., 2009). In some instances anticyclonic eddies span the length of the entire array. Therefore, considering that the model is unable to resolve the dynamics associated with meander events, for which the jet transport algorithm was specifically developed, further analysis only focuses on the box transport proxy.
3.3 Evaluating the box transport proxy
The strengths and weaknesses of the box proxy are further investigated by selecting the highest and lowest correlated years from the 34-year annual correlations (Fig. 5), and evaluated by plotting the current structure in the model over the respective years (Figs. 6 and 7).
During the year with maximum correlation (1988) the current is stable and inshore, whereas during the lowest correlated year (1994) and during the proxy reference period (2010–2013) the current is meandering and it appears that a large portion of the energy of the current has been shifted offshore (Fig. 6). The narrow spacing of the SSH contours for all three periods indicates a strong gradient inshore and hence a strong mean geostrophic current; however, the wide spacing between the SSH contours offshore suggests that the variability in the model is confined to the offshore side of the current. It is assumed that high levels of mesoscale variability in the model could bias the current position and hence the transport estimate. However, based on the analysis there were approximately five anticyclonic eddies during the highest correlated year (1988) and approximately seven anticyclonic eddies during the lowest correlated year (1994) which does not explain the difference in the accuracy of the proxy for those years.
The model cross-track velocity changes direction with depth, specifically for offshore mooring G and CPIES pairs P3P4 and P4P5 at the depth of ∼2000 m (Fig. 7), thereby defining the depth of the Agulhas jet. During the 3-year reference period the velocity changes direction at moorings B and G (∼1200 and ∼2000 m, respectively) and at sites P3P4 (∼2000 m) and P4P5 (∼300, ∼2000 m). During 1988, sites F–P4P5 experience a change in direction (≳2000 m). Lastly, during 1994 mooring G and sites P3P4 and P4P5 exhibited a change in direction (≳2000 m). An explanation for the offshore subsurface countercurrents may be due to the impinging baroclinic eddies continuously propagating downstream (Backeberg et al., 2009), affecting the entire water column by changing the direction of flow at certain depths. This directly impacts the accuracy of the proxy and explains why the transport proxy fails to capture current reversals (Table 2), because the SSH slope does not capture the subsurface countercurrents associated with the impinging baroclinic eddies.
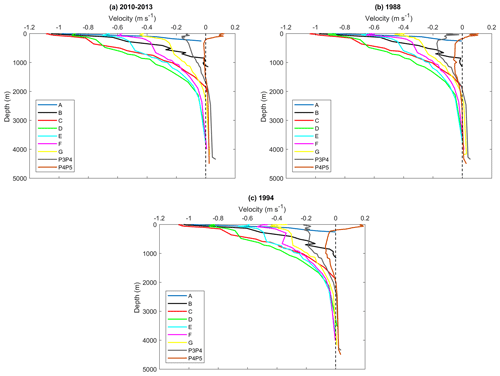
Figure 7Mean cross-track velocity profiles (m s−1) during (a) the 3-year reference period (2010–2013), (b) during the highest correlated year (1988), and (c) the lowest correlated year (1994). Each colour represents the different moorings (A–G) and CPIES pairs (P3P4 and P4P5). Negative values indicate southwestward flow.
3.4 Investigating the transport variability
As shown previously, the performance of the linear regression models weakened moving offshore (Fig. 4). Regression model RM8 (CPIES pair P3P4, Fig. 8a) captured the least transport variance at 46 % and RM 1 (mooring A, Fig. 8b) explained the most transport variance at 86 %. According to our methods, a negative SSH slope in HYCOM corresponds to a negative (southwestward) surface velocity; and if the current structure were barotropic, a negative (southwestward) transport and vice versa.
As shown in RM 1 (Fig. 8b), all the data points are clustered such that the negative SSH slope relates to a negative Tx value, in the absence of northeast counterflows. Careful analyses of RM 8 indicates that eight of the nine residual transport events violate the proportional relationship between SSH slope and Tx (Fig. 8a). Some of which have a negative SSH slope relating to a positive Tx value where others show a positive SSH slope with negative Tx value. Therefore the SSH slope does not always reflect the direction of flow at depth, and thus the correct sign for Tx.
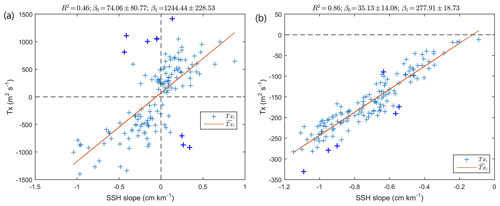
Figure 8Linear regression models showing the relationship between HYCOM SSH and transport per unit distance (Tx) for (a) RM 8, capturing the least transport variance (46 %), and (b) RM 1, capturing the most transport variance (86 %). Txi (blue crosses) represent the Tx values from HYCOM and (red line) represents the Tx estimates from the linear regression model. The bold crosses highlight the residual transport events with transport values greater or less than 2 standard deviations of the transport estimate. The coefficient of determination (R2) quantifies the amount of variance explained by the regression model; β0 is the slope coefficient and β1 the intercept with 95 % confidence intervals. Note the different scaling on the x and y axes.
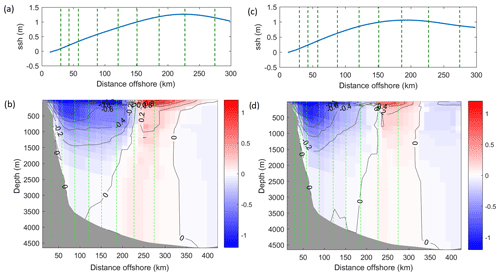
Figure 9Mean SSH (metres, m) and composite cross-track velocity structure (metres per second, m s−1) of the residual transport events from RM 8 (a, b) and RM 1 (c, d). Blue shading represents the negative, southwest current flow and red represents the positive, northeast current flow. Contours are every 0.2 m s−1. Dashed vertical lines represents the nine locations of the mooring and CPIES pairs, the first line representing mooring A and CPIES pair P4P5 furthest offshore.
It was expected that removing the outlying transport events (outliers larger than ±2 standard deviations) would increase the statistical performance of the linear regression models (Fig. 4). However, it is noteworthy that the improvement was remarkably better for the offshore regression models since the baroclinic eddies responsible for breaking down the linear relationship between SSH slope and transport frequently effected the offshore edge of the current.
Examination of the composite cross-track velocity structure of the residual transport events (Fig. 9) shows that there is a change in the direction of velocity in the bottom layers at the location of RM 8 (CPIES pair P3P4). The cross-track flow in the surface layers (∼0–700 m) of the current is southwestward, whereas below ∼700 m the flow is northeastward. Therefore, the vertically integrated flow (Tx) is positive (northeastward) and in the opposite direction implied by the SSH slope. In contrast, at mooring A (RM 1), the composite velocity field is always southwestward, consistent with the SSH slope.
3.5 Sensitivity tests
The 34-year Agulhas transport proxy under analysis thus far was based on regression models built using only 3 years of HYCOM model data. The statistics in Table 3 show the results obtained from building the linear regression models and deriving the transport proxy using 1, 3, 6, 12, 18, and 34 years of model data. We find that the correlation between proxy box transport and model box transport is not improved by using more years of model data to build the proxy. Using data from 2010 to 2013 the correlation of 0.72 changes by no more than 0.01 when extending the number of years of model data (Table 3). Similarly, building the proxy with 1 year of model data decreases the correlation by only 0.01 (Table 3). The only difference was the decrease in standard deviation.
The sensitivity of the box transport proxy was also tested using two arbitrary 3-year periods. In comparison to the correlation obtained during 2010–2013 the correlation decreased by 0.02 during 1980–1982 and remained the same during 2000–2002. The results obtained from calculating the Tbox proxy during the maximum (minimum) transport and standard deviation years in HYCOM showed no improvement or decrease in the skill of the proxy either.
The Agulhas Current transport proxies, developed by Beal and Elipot (2016), were based on nine linear regression models, each assuming a constant linear relationship from 3 years of observations between in situ transport and satellite along-track sea surface gradients. Applying constant linear models and assuming a constant vertical current structure, the transport proxies were extended using 22 years of along-track satellite data to produce two 22-year time series of Agulhas Current transports (Beal and Elipot, 2016). The Agulhas Current transport proxies in this study replicates the methods used by Beal and Elipot (2016) but applies these using a regional HYCOM simulation of the Agulhas Current (Backeberg et al., 2009, 2014).
The HYCOM transport proxies were developed using nine 3-year linear regression models between model transport and model SSH slope, and extended using 34 years of the model SSH data from 1980 to 2014. The HYCOM model provided the means to investigate the validity of the assumptions used to create the proxies, such as the constant vertical structure of the current, hence a constant relationship between SSH slope and transport per unit distance during the 3-year reference period and secondly the temporal scale of observations needed to obtain a strong linear relationship between transport and SSH slope.
Overall, results showed that the proxy was more capable of estimating the box transport (net transport) over the 34-year model period, explaining 52 % of the transport variance in comparison to 26 % of the jet transport (southwest transport) variance. A limitation of this study is that HYCOM was unable to resolve all of the observed dynamics in the Agulhas Current, specifically the mesoscale meander events. The model demonstrated much higher levels of mesoscale variability than observed (Backeberg et al., 2008, 2009). On average, 1.6 mesoscale meanders pass through the ACT array at 34∘ S per year (Rouault and Penven, 2011; Elipot and Beal, 2015). In HYCOM, an average of 5 anticyclonic eddies passed over the array per year. The poorer performance of the Tjet proxy in HYCOM (26 %) compared to the in situ Tjet proxy (55 %) of Beal and Elipot (2016) is due to various model discrepancies including the consistent merging of the anticyclonic eddies with the Agulhas Current in the northern region (Backeberg et al., 2014), which is due to poorly resolved eddy interactions and dissipation processes (Braby et al., 2016), a limitation of many numerical ocean models in this region (Tsugawa and Hasumi, 2010; Penven et al., 2011; Durgadoo et al., 2013; Backeberg et al., 2014; Loveday et al., 2014).
Furthermore, although the resolution of HYCOM is able to capture the mesoscale dynamics of eddies (Holton et al., 2017), it fails to resolve the near-coastal features, such as the inshore surface-intensified cyclonic motion in this simulation. This would require a finer resolution at the coast in order to reveal smaller offshore displacements, ∼50 km, associated with these meander events (Elipot and Beal, 2015). The poorer performance of the Tjet proxy in HYCOM and possibly in the in situ study, may also be because it only represents the southwestward component of the flow, whereas the input sea surface slope reflects the net flow along the array. Therefore, based on these findings further analysis focused on the Tbox proxy only.
The frequently impinging eddies have been found to make it difficult to effectively estimate the accurate box transport of the Agulhas Current in the model since the advection of these eddies is responsible for large transport fluctuations (Backeberg et al., 2009). The transport proxy only included the transport of the portion of the eddy that was reflected in the SSH signal across the array, whether it was the southwestward or northeastward portion of the eddy or both. Although the transport proxy may capture the SSH signal of the eddies along the array, the correlation of the regression models decreases offshore. Therefore transport estimates inshore would be more accurate than the transport estimates offshore when the current is in a meandering state.
It was shown that removing the residual transport events, violating the proportional relationship between SSH slope and transport as a result of impinging baroclinic eddies, improved the proxy performance, i.e. increased the percentage of transport variance explained. Several studies have suggested methods to decrease the levels of EKE in numerical simulations. Backeberg et al. (2009) improved the representation of the southern Agulhas Current by applying a higher-order momentum advection scheme, resulting in a well-defined meandering current rather than a continuous stream of eddies. Anderson et al. (2011) found that the use of relative wind forcing significantly decreased eddy intensities and a study by Renault et al. (2017) focused on the current stress feedback between the ocean and atmosphere and demonstrated a reduction of mesoscale variability by coupling the ocean model with an atmospheric model. Improving the mesoscale variability in HYCOM could therefore yield better results for the transport proxy, specifically for the offshore regression models, in the future. In order to effectively mirror the performance of the in situ transport proxy (Beal and Elipot, 2016), a numerical model that accurately simulates Agulhas meanders and the vertical variability, including an accurate representation of the Agulhas Undercurrent is required and this has not yet been achieved in existing regional configurations.
The development of the ACT transport proxy was initially tested using a regional Nucleus for European Modelling of the Ocean (NEMO) configuration in order to evaluate the potential of the altimeter proxy to monitor the multi-decadal transport of the Agulhas Current (van Sebille et al., 2010). Using the numerical model, it was concluded that the correlation between the Agulhas Current transport and gradient in sea surface height was greater than r=0.78 for any 3-year measuring period, and is therefore an adequate timescale to build an accurate transport proxy (van Sebille et al., 2010).
The HYCOM output in this study was used to test the sensitivity of the relationship between transport and SSH slope over a range of time periods. It was hypothesized that building the linear relationship over longer time periods, >3 years, would increase the skill of the transport proxy, since the linear relationship would include more current variability over longer periods of time. The results showed that calculating the transport proxy over longer or shorter time periods did not necessarily improve the performance of the proxy, thereby suggesting that the current dynamics for any 3-year period in the model could be very similar, in agreement with the results obtained in van Sebille et al. (2010), suggesting that the results were consistent despite the model biases. This suggests that 3 years is an appropriate time-period to develop the transport proxy of the Agulhas Current in HYCOM.
Lastly, the study showed that the transport proxy is sensitive to subsurface variability in the model, hence caution should be taken regarding the implicit assumption of a fixed vertical current structure. The accuracy of the transport proxy remains sensitive to model bias. Hence the sensitivity of the proxy should be tested in other model simulations. Sensitivity studies of this kind, using numerical ocean models, provide useful information advancing our understanding of the sensitivities and limitations of transport proxies, contributing to the improvement of long-term ocean monitoring approaches and assisting in the development and planning of future measurement programmes.
Data sets are available upon request by contacting the corresponding author.
EV conducted the data analyses and wrote up the final paper. BB provided the HYCOM model data, supervised the project, and provided financial support. JH supervised the project and provided financial support and SE assisted with the methodology of the transport proxy. All authors conceptualized ideas and contributed to writing the paper.
The authors declare that they have no conflict of interest.
This work has been funded by the National Research Foundation of South Africa and by the bilateral South Africa–Norway SANCOOP SCAMPI project. We would like to thank the Nansen-Tutu Centre in South Africa and SAEON (South African Environmental Observation Network for providing opportunities to present the project locally and internationally. We thank the Nansen Environmental and Remote Sensing Center (NERSC) in Bergen, Norway, for hosting us for a duration of the project and we wish to thank Knut-Arild Lisæter for his guidance while working at NERSC. This work also received a grant for computer time from the Norwegian Program for supercomputing (NOTUR project number nn2993k). We gratefully acknowledge Lisa Beal, Shane Elipot, and the rest of the ASCA (Agulhas System Climate Array) team from the Rosenstiel School of Marine and Atmospheric Science (RSMAS), University of Miami, for granting us permission to replicate the Agulhas transport proxy methods. Shane Elipot was supported by the U.S. National Science Foundation through the ASCA project, Award OCE-1459543.
This paper was edited by Matthew Hecht and reviewed by three anonymous referees.
Anderson, L. A., McGillicuddy, D. J., Maltrud, M. E., Lima, I. D., and Doney, S. C.: Impact of eddy-wind interaction on eddy demographics and phytoplankton community structure in a model of the North Atlantic Ocean, Dynam. Atmos. Oceans, 52, 80–94, https://doi.org/10.1016/j.dynatmoce.2011.01.003, 2011. a
Andres, M., Park, J.-H., Wimbush, M., Zhu, X.-H., Chang, K., and Ichikawa, H.: Study of the Kuroshio/Ryukyu Current System Based on Satellite-Altimeter and in situ Measurements, J. Oceanogr., 64, 937–950, 2008. a
Backeberg, B. C., Johannessen, J. A., Bertino, L., and Reason, C. J.: The greater Agulhas Current system: An integrated study of its mesoscale variability, J. Phys. Oceanogr., 1, 29–44, 2008. a, b
Backeberg, B. C., Bertino, L., and Johannessen, J. A.: Evaluating two numerical advection schemes in HYCOM for eddy-resolving modelling of the Agulhas Current, Ocean Sci., 5, 173–190, https://doi.org/10.5194/os-5-173-2009, 2009. a, b, c, d, e, f, g, h
Backeberg, B. C., Penven, P., and Rouault, M.: Impact of intensified Indian Ocean winds on mesoscale variability in the Agulhas system, Nat. Clim. Change, 2, 608–612, https://doi.org/10.1038/nclimate1587, 2012. a, b
Backeberg, B. C., Counillon, F., Johannessen, J. A., and Pujol, M. I.: Assimilating along-track SLA data using the EnOI in an eddy resolving model of the Agulhas system, Ocean Dynam., 64, 1121–1136, https://doi.org/10.1007/s10236-014-0717-6, 2014. a, b, c, d, e, f, g
Beal, L. M. and Elipot, S.: Broadening not strengthening of the Agulhas Current since the early 1990s, Nature Publishing Group, 540, 570–573, https://doi.org/10.1038/nature19853, 2016. a, b, c, d, e, f, g, h, i, j, k, l, m, n, o, p, q, r, s, t, u, v
Beal, L. M., De Ruijter, W. P. M., Biastoch, A., and Zahn, R.: On the role of the Agulhas system in ocean circulation and climate, Nature, 472, 429–36, https://doi.org/10.1038/nature09983, 2011. a, b, c
Beal, L. M., Elipot, S., Houk, A., and Leber, G. M.: Capturing the Transport Variability of a Western Boundary Jet: Results from the Agulhas Current Time-Series Experiment (ACT)*, J. Phys. Oceanogr., 45, 1302–1324, https://doi.org/10.1175/JPO-D-14-0119.1, 2015. a, b, c, d, e, f, g, h
Biastoch, A. and Krauss, W.: The Role of Mesoscale Eddies in the Source Regions of the Agulhas Current, J. Phys. Oceanogr., 29, 2303–2317, 1999. a, b
Birol, F., Fuller, N., Lyard, F., Cancet, M., Nino, F., Delebecque, C., Fleury, S., Toublanc, F., Melet, A., Saraceno, M., and Léger, F.: Coastal applications from nadir altimetry: Example of the X-TRACK regional products, Adv. Space Res., 59, 936–953, 2017. a
Bleck, R.: An oceanic general circulation model framed in hybrid isopycnic-Cartesian coordinates, Ocean Model., 37, 55–88, 2002. a
Braby, L., Backeberg, B. C., Ansorge, I., Roberts, M. J., Krug, M., and Reason, C. J. C.: Observed eddy dissipation in the Agulhas Current, Geophys. Res. Lett., 43, 8143–8150, https://doi.org/10.1002/2016GL069480, 2016. a, b
Cai, W.: Antarctic ozone depletion causes an intensification of the Southern Ocean super-gyre circulation, Geophys. Res. Lett., 33, 1–4, https://doi.org/10.1029/2005GL024911, 2006. a
Chassignet, E. P., Hurlburt, H. E., Martin, O., Halliwell, G. R., Hogan, P. J., Wallcraft, A. J., Baraille, R., and Bleck, R.: The HYCOM (HYbrid Coordinate Ocean Model) data assimilative system, J. Marine Syst., 65, 60–83, https://doi.org/10.1016/j.jmarsys.2005.09.016, 2007. a, b
Chelton, D. B., DeSzoeke, R. A., Schlax, M. G., El Naggar, K., and Siwertz, N.: Geographical Variability of the First Baroclinic Rossby Radius of Deformation, J. Phys. Oceanogr., 28, 433–460, https://doi.org/10.1175/1520-0485(1998)028<0433:GVOTFB>2.0.CO;2, 1998. a
Dee, D. P., Uppala, S. M., Simmons, A. J., Berrisford, P., Poli, P., Kobayashi, S., Andrae, U., Balmaseda, M. A., Balsamo, G., Bauer, D. P., and Bechtold, P.: The ERA-Interim reanalysis: Configuration and performance of the data assimilation system, Q. J. Roy. Meteor. Soc., 137, 553–597, 2011. a
de Ruijter, W. P. M., van Leeuwen, P. J., and Lutjeharms, J. R. E.: Generation and Evolution of Natal Pulses: Solitary Meanders in the Agulhas Current, J. Phys. Oceanogr., 29, 3043–3055, https://doi.org/10.1175/1520-0485(1999)029<3043:GAEONP>2.0.CO;2, 1999. a
Dijkstra, H. A. and de Ruijter, W.: On the Physics of the Agulhas Current: Steady Retroflection Regimes, J. Phys. Oceanogr., 31, 2971–2985, 2001. a
Durgadoo, J., Loveday, B., Reason, C., Penven, P., and Biastoch, A.: Agulhas Leakage Predominantly Responds to the Southern Hemisphere Westerlies, J. Phys. Oceanogr., 43, 2113–2131, https://doi.org/10.1175/JPO-D-13-047.1, 2013. a, b, c
Elipot, S. and Beal, L.: Characteristics, Energetics, and Origins of Agulhas Current Meanders and their Limited Influence on Ring Shedding, J. Phys. Oceanogr., 45, 2294–2314, 2015. a, b, c, d, e
Fu, L.-L., Chelton, D., Le Traon, P.-Y., and Morrow, R.: Eddy Dynamics From Satellite Altimetry, Oceanography, 23, 14–25, https://doi.org/10.5670/oceanog.2010.02, 2010. a
George, M. S., Bertino, L., Johannessen, O. M., and Samuelsen, A.: Validation of a hybrid coordinate ocean model for the Indian Ocean, J. Oper. Oceanogr., 3, 25–38, https://doi.org/10.1080/1755876X.2010.11020115, 2010. a
Gordon, A. L.: Oceanography: The brawniest retroflection, Nature, 421, 904–905, https://doi.org/10.1038/421904a, 2003. a
Gordon, A. L., Lutjeharms, J. R., and Gründlingh, M. L.: Stratification and circulation at the Agulhas Retroflection, Deep-Sea Res., 34, 565–599, https://doi.org/10.1016/0198-0149(87)90006-9, 1987. a
Hermes, J. C., Reason, C., and Lutjeharms, J.: Modeling the Variability of the Greater Agulhas Current System, J. Climate, 20, 3131–3146, https://doi.org/10.1175/JCLI4154.1, 2007. a
Holton, L., Deshayes, J., Backeberg, B., Loveday, B., Hermes, J., and Reason, C.: Spatio-temporal characteristics of Agulhas leakage: a model inter-comparison study, Clim. Dynam., 48, 2107–2121, 2017. a
Imawaki, S., Uchida, H., Ichikawa, H., and Fukasawa, M.: Satellite altimeter monitoring the Kuroshio transport south of Japan, Geophys. Res. Lett., 28, 17–20, 2001. a, b
Loveday, B. R., Durgadoo, J. V., Reason, C. J., Biastoch, A., and Penven, P.: Decoupling of the Agulhas leakage from the Agulhas Current, J. Phys. Oceanogr., 44, 1776–1797, https://doi.org/10.1175/JPO-D-13-093.1, 2014. a, b, c
Lutjeharms, J. R.: The agulhas current, vol. 5, Springer, Berlin, 2006. a, b
Maul, G. A., Mayer, D. A., and Bushnell, M.: Statistical relationships between local sea level and weather with Florida-Bahamas cable and Pegasus measurements of Florida Current volume transport, J. Geophys. Res., 95, 3287–3296, 1990. a
Penven, P., Herbette, S., and Rouault, M.: Ocean Modelling in the Agulhas Current System, in: Nansen-Tutu Conference Proceedings, 17–21, https://doi.org/10.1017/CBO9781107415324.004, July 2011, Cape Town, South Africa, 2011. a
Reason, C. J. C.: Subtropical Indian Ocean SST dipole events and southern African rainfall, Geophys. Res. Lett., 28, 2225–2227, 2001. a
Renault, L., McWilliams, J. C., Penven, P., Renault, L., McWilliams, J. C., and Penven, P.: Modulation of the Agulhas Current Retroflection and Leakage by Oceanic Current Interaction with the Atmosphere in Coupled Simulations, J. Phys. Oceanogr., 47, 2077–2100, https://doi.org/10.1175/JPO-D-16-0168.1, 2017. a
Rouault, M. and Lutjeharms, J.: Estimation of sea-surface temperature around southern Africa from satellite-derived microwave observations., S. Afr. J. Sci., 99, 489–493, 2003. a
Rouault, M., White, S. A., Reason, C. J. C., Lutjeharms, J. R. E., and Jobard, I.: Ocean Atmospheric Interaction in the Agulhas Current Region and a South African Extreme Weather Event, Weather Forecast., 17, 655–669, https://doi.org/10.1175/1520-0434(2002)017<0655:OAIITA>2.0.CO;2, 2002. a
Rouault, M. J. and Penven, P.: New perspectives on Natal Pulses from satellite observations, J. Geophys. Res.-Oceans, 116, 1–14, https://doi.org/10.1029/2010JC006866, 2011. a, b
Rouault, M. J., Mouche, A., Collard, F., Johannessen, J. A., and Chapron, B.: Mapping the Agulhas Current from space: An assessment of ASAR surface current velocities, J. Geophys. Res., 115, 1–14, https://doi.org/10.1029/2009JC006050, 2010. a, b
Smith, L., Boudra, D., and Bleck, R.: A Wind-Driven Isopycnic Coordinate Model of the North and Equatorial Atlantic Ocean 2. The Atlantic Basin Experiments, J. Geophys. Res., 95, 105–128, 1990. a
Sprintall, J. and Revelard, A.: The Indonesian Throughflow response to Indo-Pacific climate varaibility, J. Geophys. Res.-Oceans, 119, 1161–1175, https://doi.org/10.1002/2013JC009533, 2014. a
Tsugawa, M. and Hasumi, H.: Generation and Growth Mechanism of the Natal Pulse, J. Phys. Oceanogr., 40, 1597–1612, https://doi.org/10.1175/2010JPO4347.1, 2010. a, b
Uppala, S. M., Kallberg, P. W., Simmons, A. J., Andrae, U., Bechtold, V. D. C., Fiorino, M., Gibson, J. K., Haseler, J., Hernandez, A., Kelly, G. A., Li, X., Onogi, K., Saarinen, S., Sokka, N., Allan, R. P., Andersson, E., Arpe, K., Balmaseda, M. A., Beljaars, A. C. M., Berg, L. V. D., Bidlot, J., Bormann, N., Caires, S., Chevallier, F., Dethof, A., Dragosavac, M., Fisher, M., Fuentes, M., Hagemann, S., Holm, E., Hoskins, B. J., Isaksen, L., Janssen, P. A. E. M., Jenne, R., Mcnally, A. P., Mahfouf, J., Morcrette, J., Rayner, N. A., Saunders, R. W., Simon, P., Sterl, A., Trenberth, K. E., Untch, A., Vasiljevic, D., Viterbo, P., and Woollen, J.: The ERA-40 re-analysis, Q. J. Roy. Meteor. Soc., 131, 2961–3012, https://doi.org/10.1256/qj.04.176, 2005. a
van Sebille, E., Beal, L. M., and Biastoch, A.: Sea surface slope as a proxy for Agulhas Current strength, Geophys. Res. Lett., 37, 2–5, https://doi.org/10.1029/2010GL042847, 2010. a, b, c, d, e, f
Yan, X. M. and Sun, C.: An altimetric transport index for Kuroshio inflow northeast of Taiwan Island, Sci. China Earth Sci., 58, 697–706, https://doi.org/10.1007/s11430-014-5024-z, 2015. a, b
Yang, H., Lohmann, G., Wei, W., Dima, M., Ionita, M., and Liu, J.: Intensification and poleward shift of subtropical western boundary currents in a warming climate, J. Geophys. Res.-Oceans, 121, 4928–4945, https://doi.org/10.1002/2015JC010796, 2016. a
Zhu, X. H., Ichikawa, H., Ichikawa, K., and Takeuchi, K.: Volume transport variability southeast of Okinawa Island estimated from satellite altimeter data, J. Oceanogr., 60, 953–962, https://doi.org/10.1007/s10872-005-0004-8, 2004. a