the Creative Commons Attribution 4.0 License.
the Creative Commons Attribution 4.0 License.
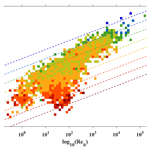
Dissipation ratio and eddy diffusivity of turbulent and salt finger mixing derived from microstructure measurements
Jianing Li
Eddy diffusivity is usually estimated using the Osborn relation assuming a constant dissipation ratio of 0.2. In this study, we examine dissipation ratios and eddy diffusivities of turbulent mixing and salt finger mixing based on microstructure datasets. We find that the dissipation ratio of turbulence, ΓT, is highly variable with a median value clearly greater than 0.2, which shows strong seasonal variation and decreases slightly with depth in the western equatorial Pacific but obviously increases with depth in the midlatitude Atlantic. ΓT is jointly modulated by the Ozmidov scale to the Thorpe scale ratio ROT and the buoyancy Reynolds number Reb, namely ⋅ . The eddy diffusivity based on observed ΓT is larger than that estimated with 0.2 and presents a much stronger bottom enhancement. The eddy diffusivities of heat and salt for a salt finger are calculated using two “analogical” Osborn equations, and their corresponding “effective” dissipation ratios and are examined. scatters over 2 orders of magnitude with a median value of 0.47 and is mostly linearly correlated with as 5 . The density flux ratio for a salt finger decreases sharply with a density ratio Rρ smaller than 2.4 but regrows to a larger value with Rρ exceeding 2.4. The salt-finger-induced eddy diffusivities also increase with depth, with some being comparable to even stronger ones than the mean turbulent ones. This study highlights the influences of variable dissipation ratios and different mixing types on eddy diffusivity estimates and should help the improvement of mixing estimate and parameterization.
- Article
(10662 KB) - Full-text XML
- BibTeX
- EndNote
Microscale turbulence in the ocean is patchy and intermittent. Compared with molecular diffusion, it mixes materials in a larger scale with a higher efficiency, playing a leading role in redistributing heat (Pujiana et al., 2018), dissolved gases (Sabine et al., 2004), pollutants (Kukulka et al., 2016), nutrients, and plankton (Whitt et al., 2017), thus shaping ocean general circulations and influencing biochemical processes in the ocean (Wunsch and Ferrari, 2004). These effects impact the global environment and climate change (Jackson et al., 2008).
Due to these significant effects of microscale mixing, the outputs of ocean general circulation and climate models are deeply affected by the mixing intensity and variation (Jayne, 2009). Since the grid size is too coarse to resolve microscale processes, mixing parameterizations are mostly used as a proxy for turbulence effects in such models (Klymak and Legg, 2010). The proposal, verification, and development of mixing parameterizations heavily rely on our perceptions of mixing intensity and spatiotemporal variation observed in the real ocean. Therefore, accurately estimating eddy diffusivity based on observations has been an unremitting pursuit for researchers. On one hand, many parameterization methods have been developed and are widely used to infer eddy diffusivity (e.g., GHP scaling, Gregg et al., 2003; MG scaling, MacKinnon and Gregg, 2003; the Thorpe scale method, Dillon, 1982), thanks to the abundant accumulation of traditional hydrographic observations. These methods yield a mediocre estimate based on fine-scale profiles of temperature and/or velocity with a resolution significantly larger than microscale and may have applicability problems induced by different mechanisms and hydrologic conditions (Mater et al., 2015). On the other hand, microstructure measurements provide a much more accurate estimate of turbulence behaviors (St. Laurent et al., 2012), although the amount of data is relatively small. With the development of observation technology and the advancement of instruments, microstructure data are experiencing rapid growth. However, neither parameterizations nor microstructure measurements can directly provide eddy diffusivity values; what they infer is the dissipation rate of turbulent kinetic energy (TKE) ε. Assuming mixing is driven by turbulence, the eddy diffusivity of density is then estimated by the conventional Osborn relation, with 0.2 (e.g., St. Laurent et al., 2012), where Rf is the flux Richardson number, N2 is the buoyancy frequency squared, and ΓT is the dissipation ratio of turbulence.
However, there are two inadequacies in the application of the Osborn relation. First, the value of ΓT should be carefully inspected. In the frame of steady, homogeneous turbulence, a balance between TKE production (P), buoyancy flux (B), and dissipation can be reached, 0. And ΓT is the ratio of the buoyancy flux to the dissipation, , which describes the relative proportion of TKE converted to potential energy and irreversibly dissipated to heat. Combining limited measurements with theoretical prediction, Osborn (1980) established a critical value for Rf as Rf≤0.15, resulting in . Consequently, ΓT is usually taken as a constant of 0.2. Eddy diffusivities of heat (Kθ), salt (KS), and density are equal for turbulent mixing, so these diffusivity values can be easily determined by the Osborn relation as long as ΓT is accurately measured. ΓT≈ 0.2 is confirmed to be reasonable by some observations (Gregg et al., 2018); however, besides findings from laboratory experiments and direct numerical simulations (Barry et al., 2001; Jackson and Rehmann, 2003; Shih et al., 2005; Salehipour et al., 2016), there is considerable and accumulating observational evidence indicating that ΓT is significantly variable in both space and time, with a range covering several orders of magnitude, typically from 10−2 to 10 (Moum, 1996; Smyth et al., 2001; Mashayek et al., 2017; Ijichi and Hibiya, 2018; Monismith et al., 2018; Vladoiu et al., 2021; Li et al., 2023).
Observations conducted in different regions showed that the statistical feature of ΓT is significantly distinct from region to region, and repeated measurements at some locations have suggested that ΓT is obviously greater than 0.2 (Ijichi and Hibiya, 2018), indicating that taking ΓT= 0.2 could significantly underestimate eddy diffusivity in these regions. Also, microstructure measurements from both the upper layer and the whole water column suggested that ΓT generally increases with depth by as much as an order of magnitude (Ijichi and Hibiya, 2018; Li et al., 2023). Thus, taking ΓT as a constant also leads to an underestimate of eddy diffusivity in the deep layer. These underestimated eddy diffusivities may be a part of the answer to “the missing mixing” puzzle (Wunsch and Ferrari, 2004). Some studies do show that the magnitude and pattern of ΓT play a key role in regulating global ocean general circulation (Mashayek et al., 2017; Cimoli et al., 2019). Moreover, ΓT is reported to be modulated by turbulence features and is closely correlated with several parameters describing the turbulence state, such as turbulence “age” ROT (the ratio of the Ozmidov scale to the Thorpe scale; Ijichi and Hibiya, 2018) and turbulence “intensity” Reb (buoyancy Reynolds number; Mashayek et al., 2017). However, different correlations between ΓT and these parameters are found in different regions. Taking Reb as an example, different studies concluded that their relation could be negatively correlated (Monismith et al., 2018), nonmonotonically correlated (Mashayek et al., 2017), or uncorrelated (Ijichi and Hibiya, 2018). In a word, taking ΓT as a constant of 0.2 brings a large bias into eddy diffusivity estimate, yet our limited understanding prevents us from assigning a reasonable value for ΓT.
The other inadequacy involves the driving mechanism of mixing. Although turbulent mixing dominates ocean mixing, there are considerable mixing events caused by the release of potential energy due to unstable temperature or salinity stratification (while the density stratification is stable) – that is, double diffusion (Schmitt, 1994). Double diffusion has two manifestations: salt fingers and diffusive convection. The former occurs when warmer, saltier water overlays colder, fresher water, while the latter corresponds to the opposite scenario. Due to their unique requirements of vertical structures for temperature and salinity, diffusive convection is most prominent in the polar and subpolar regions, while salt fingers prevail in tropical and subtropical regions (van der Boog et al., 2021), and the salt finger is our focus in this study. For the importance of salt finger mixing, analysis of a global thermohaline staircase indicated that a salt finger only contributes a small fraction of the required energy to sustain mixing (van der Boog et al., 2021); however, not all salt finger events present staircases (St. Laurent and Schmitt, 1999), and the regional effects of salt finger mixing can be much profound (Fine et al., 2022). Some studies suggested that salt finger mixing is significant when turbulent mixing is weak, while others suggested that a salt finger and turbulence can co-exist and interact with each other (Ashin et al., 2023). Unlike turbulent mixing, salt finger mixing, supplied by the release of potential energy, acts to strengthen the density stratification with a negative value of Kρ. With P being negligible, the balance between B and ε leads to −1, and hence is applied to salt fingers (McDougall, 1988). Therefore, if the mixing mechanism is not identified clearly, the conventional Osborn relation can estimate neither the correct sign nor the accurate magnitude of the eddy diffusivity of density for salt finger mixing. Also, the eddy diffusivities of heat, salt, and density for salt finger mixing are inequivalent, namely Kθ < KS (Schmitt et al., 2005). Therefore, Kθ and KS for salt finger mixing cannot be estimated by the Osborn relation, and they can be calculated by a different manner involving the dissipation ratio ΓF (note that ΓF for a salt finger is equivalent to instead of ); St. Laurent and Schmitt, 1999), density ratio Rρ (describing the relative contributions of temperature and salt to density), and density flux ratio r (the ratio of vertical heat flux to vertical salt flux) (see Sect. 2.3).
To overcome the shortcomings mentioned above, we turn to open microstructure datasets (Sect. 2) to first identify salt finger mixing and turbulent mixing (Sect. 3). Then, we explore the variability of ΓT for turbulent mixing (Sect. 4.1) and examine ΓF and the relation between Rρ and r for salt finger mixing (Sect. 4.2). We also derive diffusivities Kρ, Kθ, and KS and analyze them for both turbulent mixing and salt finger mixing (Sect. 5). A summary is given in Sect. 6.
2.1 Data
We first thank the Climate Process Team for publicly sharing the Microstructure Database (MacKinnon et al., 2017). The data used in this study are selected from the shared microstructure sampling projects covering global oceans. Since the calculation of the dissipation ratio requires the dissipation rate of thermal variance (χθ), and the vertical gradients of temperature θ and salinity S are needed, we chose all five projects that provide χθ in the form of vertical profiles. Besides χθ, θ, and S, we also use ε, which has been standardized to the same vertical grid for each project. The locations, operating period, and other information for the five projects are given in Table 1 and Fig. 1. The MIXET projects are performed in the western equatorial Pacific, while BBTRE and NATRE are conducted in the Atlantic between 40° S and 40° N. A salt finger is always active in the middle to low latitudes of the Atlantic, while its occurrence in the Pacific shows strong temporal variation (Oyabu et al., 2023). These data provide a great opportunity to investigate the spatial–temporal variation of the dissipation ratio and eddy diffusivity induced by turbulent mixing and salt finger mixing.
Table 1Information on the projects used in this study.
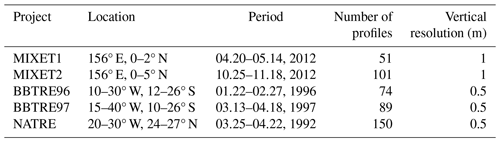
MIXET: MIXing in the Equatorial Thermocline (Waterhouse et al., 2014; Richards et al., 2015). BBTRE: Brazil Basin Tracer Release Experiment (Polzin et al., 1997). NATRE: North Atlantic Tracer Release Experiment (St. Laurent and Schmitt, 1999; Polzin and Ferrari, 2004).
2.2 Identifying turbulent mixing and salt finger mixing
The profiles are divided into half-overlapped patches for further analysis. Following St. Laurent and Schmitt (1999), we choose 10 times the vertical resolution as the patch size – that is, 10 m (5 m) for projects with a vertical resolution of about 1 m (0.5 m). We first examine if and which type of double diffusion is favorable for each patch in a thermodynamical sense by the Turner angle, Tu = tan−1() (Ruddick, 1983). Here, α and β are the thermal expansion and saline contraction coefficients, respectively; θz and Sz are the vertical gradients of the original temperature and salinity profiles, respectively; and atan−1 is the four-quadrant inverse tangent. Tu varies between −180 and 180°, categorizing the water column into four thermodynamical regimes: doubly stable (|Tu| < 45°), salt-finger-favorable (45° < Tu < 90°), diffusive-convection-favorable (−90° < Tu < −45°), and gravitationally unstable (|Tu| > 90°) (Ruddick, 1983). Tu is related to the density ratio Rρ by ). We exclude weak double-diffusion signals (45° < Tu < 60° for salt-finger-favorable and −60° < Tu < −45° for diffusive-convection-favorable) for further identification.
In addition to the specific thermodynamical precondition, distinct statistical features are presented when double-diffusion-induced mixing is dominant. First, Reb is found to be no greater than O(10) for active double diffusion (Inoue et al., 2007) and salt fingers are rare for Reb between 10 and 104 (St. Laurent and Schmitt, 1999). Reb is defined as , where ν is the molecular viscosity coefficient. Moreover, double diffusion generally corresponds to elevated χθ (St. Laurent and Schmitt, 1999; Inoue et al., 2007), and the magnitude of χθ is significantly larger than ε when double diffusion prevails and turbulence is absent (Nagai et al., 2015). Therefore, we use Reb < 25 and 7 as additional criteria for the identification of double diffusion.
For a doubly stable and gravitationally unstable water column, since their thermodynamical condition excludes the existence of double diffusion, we assume the mixing within the column is solely induced by turbulence. The most prominent difference between turbulence patches with |Tu| < 45° and those with |Tu| > 90° is that Reb of the former is significantly smaller than that of the latter. And |Tu| > 90° generally means the presence of overturns. Therefore, the former patches are grouped as “weak turbulence” and the latter represent “energetic turbulence”.
Based on Tu, Reb, and , we classify the dominant mixing mechanisms into four types: weak turbulence (|Tu| < 45° with small Reb), energetic turbulence (| Tu| > 90° with large Reb), salt fingers (60° < Tu < 90°, Reb < 25 and 7), and diffusive convection (−90° < Tu < −60°, Reb < 25 and 7). Diffusive convection prevails mostly in the polar and subpolar regions (van der Boog et al., 2021); thus, it is rarely identified in this study (Sect. 3). As a result, diffusive convection is excluded from further analysis.
2.3 Estimating dissipation ratios and eddy diffusivities for turbulent mixing and salt finger mixing
The dissipation ratio Γ is defined as
for turbulent mixing and salt finger mixing (Oakey, 1985). Based on the production–dissipation balances for TKE and thermal variance (Osborn and Cox, 1972; Osborn, 1980), and introducing Rρ and the density flux ratio , we get
which is applicable to both turbulent mixing and salt finger mixing (please refer to St. Laurent and Schmitt, 1999, for the specific derivation).
For turbulent mixing only, . Then, Eq. (2) leads to
and
where the superscript “T” indicates turbulent mixing.
However, for salt finger mixing only, with (St. Laurent and Schmitt, 1999), Eq. (2) yields
which cannot be used directly to estimate the salt-finger-induced eddy diffusivities. They are estimated separately by introducing Rρ and (St. Laurent and Schmitt, 1999; Schmitt et al., 2005; Inoue et al., 2007):
Note that all these equations are written in forms analogical to the Osborn relation for turbulent mixing. and are two artificial “mixing efficiencies”, which are actually and before for and estimation. is the same as ΓF, while is further derived based on Rρ and rF: . Investigating the statistic features of and can be practically useful when estimating and solely based on ε and N2.
Figure 2 suggests that water properties vary greatly for the five projects, and Table 2 lists the proportions of patches for each mixing type. For the MIXET projects in the western equatorial Pacific, the Tu distribution in spring (MIXET1) shows a shape distinct from the autumn one (MIXET2). In spring, Tu shows double peaks at −30 and 110°, suggesting that mixing is alternately dominated by weak and energetic turbulence, although the contribution of the salt finger accounts for 4.1 % of the total patches and cannot be neglected. However, the autumn distribution is obviously unimodal, peaking at ∼ 45°, and the dominant mixing types are first weak turbulence and second salt finger mixing (∼ 51.5 % and 11.3 %, respectively), with negligible energetic turbulence and diffusive convection. For the BBTRE projects, although they are conducted in different years, the operating seasons are similar: one in late summer and the other early autumn (Southern Hemisphere), so the seasonal variation cannot be studied. Their Tu distributions are similarly bimodal, with a leading peak at 70° and a weak one at −40°, suggesting that the waters are mostly salt-finger-favorable (although only about 5.9 % is confirmed to be a salt finger) and stable (33.3 %), with rare energetic turbulence and neglectable diffusive-convection-favorable contribution. For NATRE, the salt finger overwhelms the others, occupying more than 21 % of the total patches; weak and energetic turbulence together hold 13.3 %, with the diffusive-convection-favorable type still being negligible (1 %). For BBTRE and NATRE, although a large proportion of the patches have 45° < Tu < 90° and are hence salt-finger-favorable, most of them have elevated Reb; thus, we infer these mixing events to be hybrids of a salt finger and turbulence but dominated by turbulence. These patches are excluded from analysis to highlight the difference between turbulent and salt finger mixing. Only a few patches are chosen as effective salt finger events. Therefore, as concluded in the following section (Sect. 5.3), it is turbulent mixing that dominates the observed microstructures, in line with the results based on NATRE (St. Laurent and Schmitt, 1999). For these five projects, only 9.7 % of patches show clear salt finger features. Weak turbulence has a higher percentage (32.0 %), followed by 6.6 % of energetic turbulence. Diffusive convection occurs in less than 0.5 % of the total patches and is therefore negligible. Although dominated by turbulent mixing, the rest of the patches, more than 50 %, are hybrids of different mixing types and are excluded from the analysis.
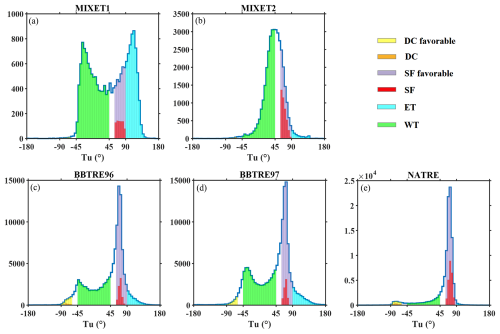
Figure 2Histograms of patch-averaged Tu for different projects. Different Tu ranges of mixing types are marked by different colors: yellow for diffusive-convection-favorable (DC favorable; −90° < Tu < −60°), light purple for salt-finger-favorable (SF favorable; 60° < Tu < 90°), cyan for energetic turbulence (ET), and green for weak turbulence (WT). The red and orange bars denote the actual number of patches of salt fingers (SF) and diffusive convection (DC) selected by two more criteria, Reb < 25 and 5, respectively.
Table 2Proportions of patches with energetic turbulence, weak turbulence, salt finger, diffusive convection, and hybrid mixing types (turbulence and salt finger, or turbulence and diffusive convection) compared to the total number of patches for each project and the sums for all the projects. Patches with hybrid mixing types are excluded from analysis.
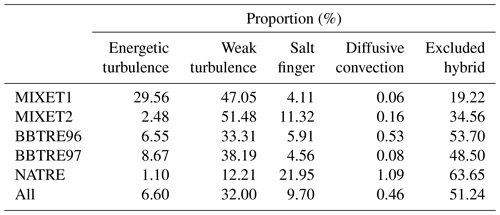
We compare the statistical differences of Reb, ε, N2, and χθ for energetic turbulence, weak turbulence, and salt fingers by analyzing all the patches from the five projects (Fig. 3). The salt finger patches are featured with the weakest turbulence intensity compared with weak and energetic turbulence patches, whose median Reb values are 5.0, 18.2, and 132.7, respectively. The median Reb of energetic turbulence is slightly smaller than that reported in Mashayek et al. (2017) but close to the findings of Ijichi and Hibiya (2018). Since the samples given here are from five different projects, their Reb distributions are actually different: for MIXET projects, the median Reb of energetic turbulence is small, only about 50, while the rest of the projects generally have a median Reb around 200 for energetic turbulence. The variations of ε for different mixing types differ little, mostly ranging from 3 × 10−12 to 3 × 10−8 W kg−1. Although the median ε for energetic turbulence is not obviously different from those for weak turbulence and salt fingers (7.8 × 10−11, 7.9 × 10−11 and 1.1 × 10−10 W kg−1, respectively), it should be noted that most large ε values are induced by energetic turbulence. Distributions of χθ for weak turbulence and energetic turbulence differ little, but χθ of a salt finger is clearly greater in terms of variation ranges (salt finger: 3 × 10−11–10−7 °C2 s−1; weak turbulence and energetic turbulence: 10−13–10−7 °C2 s−1) and median values (salt finger: 1.8 × 10−9 °C2 s−1; energetic turbulence and weak turbulence: 1.5 × 10−11 °C2 s−1). Earlier studies considered the doubly stable regime to involve no mixing or excluded it from analysis (Inoue et al., 2007); however, besides some slight differences of proportion in large χθ and ε, energetic turbulence and weak turbulence share very similar distributions of χθ and ε (Fig. 3b, d), suggesting that the doubly stable regime does not mean an absence of turbulence and should be dominated by weak turbulence. Stratification also presents different features for different mixing types. Energetic turbulence has the weakest stratification with a median of 6.1 × 10−7 s−2, only of that for weak turbulence. And the salt finger presents the strongest stratification (1.9 × 10−5 s−2). Clearly, the identified patches with energetic turbulence, weak turbulence, and salt fingers have distinct turbulent features, verifying the validity of the chosen criteria.
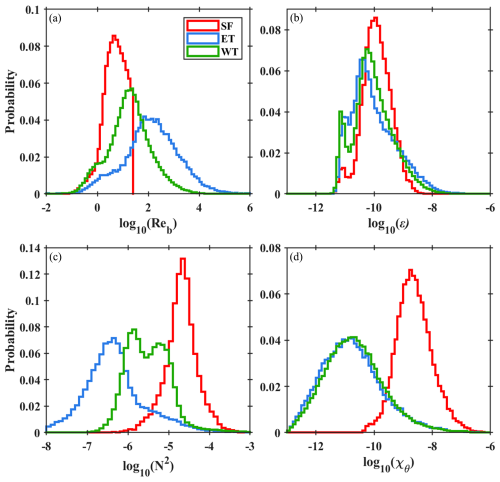
Figure 3Probability-normalized histograms of log10(Reb) (a), log10(ε) (b), log10(N2) (c), and log10(χθ) (d) for different mixing types: SF (salt finger), ET (energetic turbulence), and WT (weak turbulence). Data from the five projects are taken as the whole collection.
A normalized occurrence frequency is calculated to quantify the vertical variation of each mixing type (Fig. 4). Taking energetic turbulence as an example, we first divide the number of energetic turbulence patches in each depth bin by the total number of energetic turbulence patches in the whole project; then, to eliminate the vertical variation of observation frequency, we divide the results by the total number of patches within the same depth bin. This occurrence frequency is eventually normalized between 0 and 1 using its maximum. Consistent with observations in the upper thermocline (Schmitt et al., 2005; van der Boog et al., 2021), salt fingers mostly prevail in the upper 500–1000 m for all projects, with their occurrence frequencies reaching 1. For the MIXET projects and NATRE, the occurrence frequencies of a salt finger gradually become weak and near zero with depth increasing to the seafloor. However, for the BBTRE projects, salt fingers sharply disappear between 1000 and 2000 m and re-occur at greater depth (see Fig. 10). The depth-colored T–S diagrams suggest that the vertical transition of different water masses is responsible for the sudden disappearance of the salt finger (Fig. 5). It is clear to see that both θ and S decrease with depth in most water columns, providing the basic precondition for a salt finger. However, this tendency changes obviously between 1000 and 2000 m. At this depth range, θ changes little, but S increases drastically by at least 0.5; this prevents the occurrence of a salt finger. This depth is just where the fresher Antarctic Intermediate Water transits to the North Atlantic Deep Water. Consequently, the occurrence frequency of a salt finger is severely weakened at this depth. In contrast to salt fingers, energetic turbulence generally becomes more prevalent with increasing depth for most projects. The remarkably weak background stratification may contribute a lot to the prevalence of energetic turbulence at depth, where even a weak perturbation can fully develop.
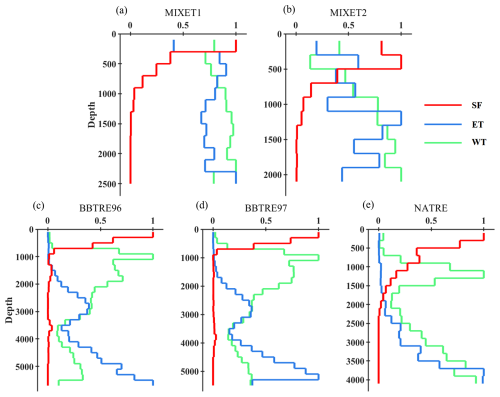
Figure 4Vertical variations of the normalized occurrence frequency of a salt finger (SF), energetic turbulence (ET), and weak turbulence (WT) for the five projects. The depth range is from 100 m to the deepest measurements, with a bin size of 200 m.
4.1 Γ variation of turbulence
4.1.1 Vertical variation
We explore the variation of ΓT first. Figure 6 suggests that ΓT varies in a distinct way for different projects. Results for the MIXET projects suggest that ΓT in the western equatorial Pacific is significantly seasonally variable. In spring (MIXET1), ΓT of energetic turbulence varies between 2.5 × 10−2 and 1.7 (10th–90th percentiles) with a median of 0.23, smaller than that of weak turbulence ranging between 1.4 × 10−1 and 2.8 and peaking at 0.52. ΓT in autumn is significantly elevated (MIXET2), and the medians and variation ranges for energetic turbulence and weak turbulence are 0.41 and from 3.4 × 10−2 to 8.8 as well as 0.58 and from 1.7 × 10−1 to 2.3, respectively. For the BBTRE projects, ΓT of weak turbulence varies little between different years, with most patches varying between 10−2 and 10, although the median value in 1997 (0.35) was greater than that in 1996 (0.20). ΓT of energetic turbulence is larger in 1997 than that in 1996, with median values of 0.48 and 0.20, respectively. Estimates from NATRE also suggest that ΓT largely scatters between 10−2 and 10 for most patches; the median ΓT values are 0.33 and 0.50 for energetic turbulence and weak turbulence, respectively. To summarize, besides the BBTRE and energetic turbulence of the MIXET projects showing a median value close to 0.2, the rest of the estimates are all clearly greater than 0.2. ΓT for the five projects mostly varies within 3 orders of magnitude from 10−2 to 10, in line with other observations (Ijichi and Hibiya, 2018; Vladoiu et al., 2021; Li et al., 2023).
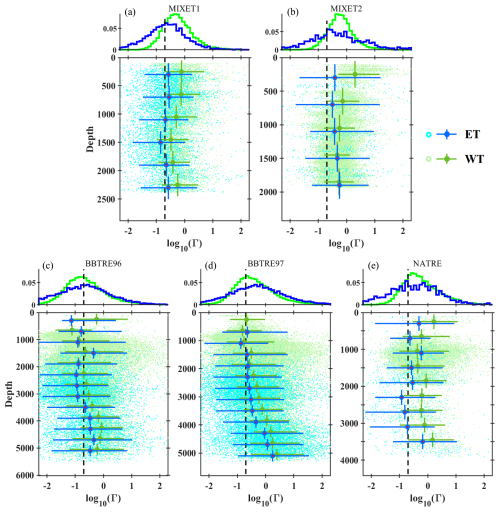
Figure 6Variations of ΓT of energetic turbulence (ET) and weak turbulence (WT). Each panel consists of two sub-panels, with the upper one showing a probability-normalized histogram of ΓT and the lower one being ΓT–depth scatter; the median value of each depth bin is marked by a larger, darker dot overlying a cross marker, with a horizontal bar indicating the 10th to 90th percentile range and vertical bar indicating the depth bin range. The median Reb values are compared between energetic turbulence and weak turbulence at each depth bin, and the median ΓT corresponding to the larger Reb is marked by a red dot. The conventional value of ΓT, namely 0.2, is represented by the dashed black line.
For different projects, ΓT varies with depth in different ways. For MIXET1, ΓT of both energetic turbulence and weak turbulence fluctuates around the statistical median values weakly. For MIXET2, the depth median ΓT of energetic turbulence varies between 0.2 and 0.7, with a slight increase with depth. However, ΓT of weak turbulence shows a clear decrease from 2.5 at 300 m to 0.6 at 1400 m; then, it slightly increases to 0.8 at 1900 m. The ΓT of weak turbulence for BBTRE96 fluctuates around 0.2 in the upper 300 m, and then it increases to ∼ 1 at 4400 m and decreases to ∼ 0.6 at 5200 m. The scenario for energetic turbulence shows a similar picture. ΓT of weak turbulence for BBTRE97 departs little from 0.2 at depths above 1800 m, then monotonically increases to ∼ 2.3 at the deepest depth around 5200 m. ΓT of energetic turbulence varies in a similar way in the vertical direction, except the depth where the trend change is 3000 m. For NATRE, ΓT of energetic turbulence first decreases from 0.6 to 0.1 at 2300 m, then increases to 0.8 at 3500 m. As for weak turbulence, ΓT stays around 0.8 between 600 and 3000 m and then increases beyond unity at 3500 m. In terms of the general trend by linearly fitting ΓT with depth, the five projects show two distinct vertical patterns of ΓT: one is the downward-decreasing pattern represented by the MIXET projects, and the other is the downward-increasing one suggested by the rest of the projects over the midlatitudes of the Atlantic. Downward-increasing ΓT was also reported by Ijichi and Hibiya (2018). Their data collection sites were spread over middle to high latitudes of the Pacific and Southern Ocean. ΓT also presented a clear downward increase in the upper 500 m of the South China Sea north of 10° N (Li et al., 2023). Combining all these observational results, we suggest that ΓT in the equatorial area should be treated differently, since it may decrease with depth, contrary to the downward increase away from the Equator.
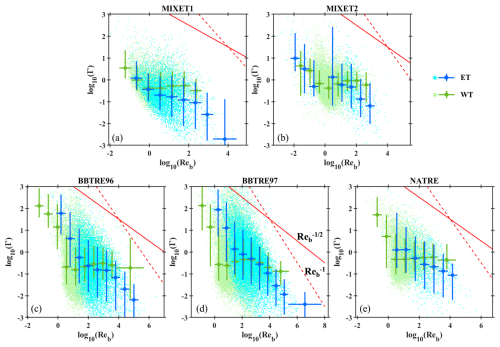
Figure 7Relations between ΓT and Reb for energetic turbulence (ET) and weak turbulence (WT). Overlying the light-colored scatter of individual patches, Reb-binned median values are marked by large darker dots, and the bin size and the 10th–90th percentile range of ΓT are denoted by the horizontal and vertical bars, respectively. The solid and dashed red lines mark and , respectively.
The full-depth statistics of the five projects disagree regarding whether ΓT is larger for energetic turbulence or weak turbulence. However, when comparing ΓT values of energetic turbulence and weak turbulence in the same depth bin, ΓT of energetic turbulence is mostly smaller than that of weak turbulence. Considering that Reb is reported to modulate the variation of ΓT (Mashayek et al., 2017; Monismith et al., 2018) and that energetic turbulence and weak turbulence have clearly different Reb distributions (Fig. 3), we found that energetic turbulence with smaller ΓT generally has larger Reb than weak turbulence, indicating a negative correlation between ΓT and Reb.
4.1.2 Relation between ΓT, Reb, and ROT
We then investigate the relations between ΓT and Reb for energetic turbulence and weak turbulence (Fig. 7). For MIXET1, ΓT of weak turbulence first decreases from 3.5 to 0.5 with Reb increasing from 0.1 to 1, suggesting a relation of , and then it weakly increases to 0.7 with Reb reaching 100; a weak decrease in line with can be observed for Reb > 100. For energetic turbulence, ΓT generally decreases with Reb, indicating ; this relation is consistent with the observations in the western Mediterranean Sea (Vladoiu et al., 2021). The pattern for MIXET2 is similar to that for MIXET1, although ΓT of weak turbulence decreases at a smaller rate when Reb is small and indicates . Excluding the bins with few data points, ΓT of weak turbulence for BBTRE96 shows a weak increase from 0.2 to 0.3 as Reb grows from 10 to 103 and a weak decrease with Reb exceeding 103. ΓT and Reb of weak turbulence for BBTRE97 show similar relationships as those for BBTRE96. The weak turbulence variations for BBTRE are the same as the estimates reported by Ijichi et al. (2020), and the shape is similar to the upper bound of the nonmonotonic ΓT∼ Reb relation proposed by Mashayek et al. (2017). It is notable that the scenario for energetic turbulence is distinct; ΓT generally decreases from 5 to less than 0.1 with Reb between 10 and 2.5 × 104 for BBTRE96, forming a fitting slope steeper than but flatter than −1. ΓT of energetic turbulence for BBTRE97 also shows a similar decrease with Reb. Except for the bins with few samples when Reb < 1 and Reb > 104, ΓT of weak turbulence for NATRE generally increases from 0.5 to 0.7, while ΓT of energetic turbulence monotonically decreases from ∼ 1 at Reb= 102 to ∼ 0.1 at Reb=104, suggesting .
Although ΓT generally decreases with Reb in most cases for the five projects, the decreasing rate varies with projects and Reb ranges. There are several cases showing that ΓT stays constant or even increases with Reb. This suggests that ΓT is not solely modulated by Reb, and there may be other factors that influence ΓT in a comparable or even dominating role relative to Reb. ROT is reported as a parameter that regulates ΓT more strongly than Reb, (Ijichi and Hibiya, 2018). ROT is the ratio of the Ozmidov scale LO to the Thorpe scale LT, with and , where the Thorpe displacement δT is the depth difference of a water parcel between the original and sorted potential temperature profiles of an overturn. Overturns are identified by the cumulative Thorpe displacement ∑δT (Mater et al., 2015; Ijichi and Hibiya, 2018). Because the vertical resolution of temperature profiles is 1 or 0.5 m, overturns with a vertical size of O(1) m or smaller cannot be identified. Additionally, the identified overturns with a size smaller than 10 m or greater than 400 m are excluded from analysis because the former contain too few data points and the latter are possibly the vertical structures of different water masses instead of genuine turbulent overturns. We also estimate the overturn-averaged Tu, ΓT, and Reb. Due to the coarse vertical resolution of temperature profiles used in our study, only a few overturns meet the identification criteria for each project; as a result, the overturns of the five projects are taken as one collection (the total number of overturns is 3862).
Figure 8 shows the overturn-based relation between ΓT and ROT. Since most overturns are identified at depth, with only one-fifth shallower than 1000 m but more than one-third below 2000 m, the overturn-based ΓT is clearly greater than 0.2, with a median value of 0.91. In Fig. 8, although overturns are evenly scattered in the ROT–ΓT space, the probability density shows that they are concentrated around two sites mostly, one with ROT and ΓT of 0.03 and 1.19 and the other with 0.56 and 0.53. These two clusters are distinctly characterized by Reb, with the first location corresponding to Reb < 160 (median value of 25) and the other to Reb > 160 (median value of 835). For both clusters, the contours of probability density tilt at slopes of , confirming is valid for each cluster. However, the general trend between ROT and ΓT for the whole data collection is much flatter, with a slope of only about . Comparing Reb of the two clusters, it is easy to find that Reb grows exponentially with ROT. Therefore, the general variation of ΓT with the growth of ROT is not only influenced by ROT, but also partly affected by Reb. Supposing ΓT is mostly modulated by these two parameters, and considering that the decrease in ΓT with ROT is significantly weakened by Reb, this suggests a positive relation between ΓT and Reb.
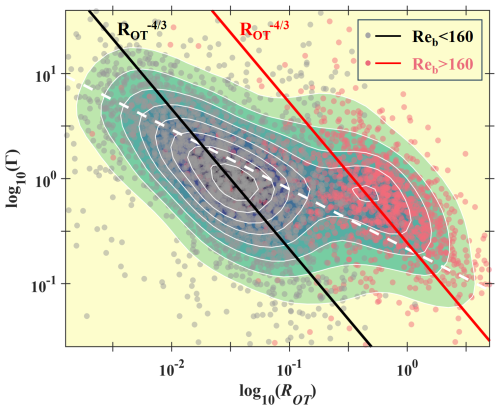
Figure 8Relation between overturn-based ΓT and ROT; overturns from the five projects are considered. The shading describes the distribution of probability density, with yellow indicating minimum probability density and blue representing the maximum one. The overturns are correspondingly divided into two clusters: the gray dots have Reb < 160 and the pink ones Reb > 160. The black and red lines represent , crossing the centers of the two clusters. The dashed white line is the general relation between ΓT and ROT of the whole data collection.
Figure 9 shows the variation of the median value of ΓT jointly binned by Reb and ROT. Note that most parts of the Reb–ROT space are null, with all the data gathered around a band originating from large Reb and ROT to small Reb and ROT. This confirms that Reb and ROT are positively correlated in general. As for the median ΓT, although its value is scattered, its general pattern indicates that ΓT grows fastest along a direction from small Reb and large ROT to large Reb and small ROT, suggesting that ΓT is indeed positively correlated with Reb and negatively correlated with ROT. Assuming , we substitute the median values of ΓT, Reb, and ROT in Fig. 9 into this relation to fit the exponent c. The fitting results suggest c≈ 1/2 and a relation of ΓT≈10. The isolines of this relation are shown in Fig. 9, which can capture the main variation of ΓT well with Reb and ROT. Based on the microstructure measurements collected from the upper layer in the South China Sea, Li et al. (2023) presented a relation of , but a is around 0.02 in that region, which is 1 order of magnitude larger than the value presented here. This is because Reb has a much smaller magnitude in the upper South China Sea, with most Reb values varying between 10−1 and 103. Therefore, compared with the results in Li et al. (2023), the larger Reb in this study leads to a relatively smaller a. On the other hand, the significant variation of a may suggest that some other parameters can influence ΓT besides Reb and ROT.
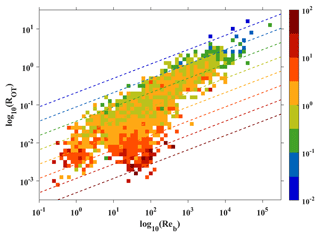
Figure 9Variation of median ΓT binned by ROT and Reb based on overturn estimates for the five projects. The color bar refers to the median ΓT, and the dashed colored lines indicate the isolines of 10.
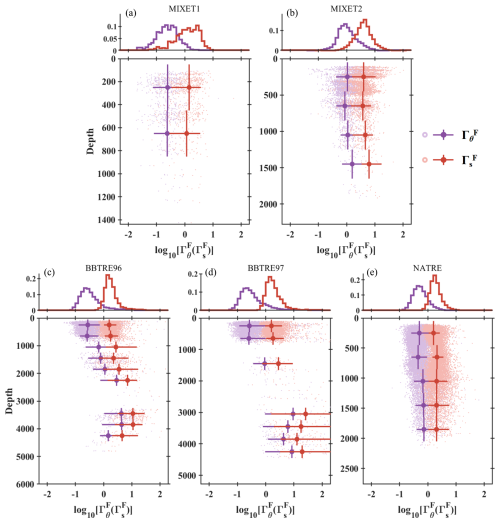
Figure 10Variations of ) of a salt finger for the five projects. Each panel consists of two sub-panels, with the upper one showing the probability-normalized histograms of and and the lower one being their vertical variations. The median value of each depth bin is marked by a larger, darker dot overlying a cross marker, with a horizontal bar indicating the 10th to 90th percentile range and a vertical bar indicating the depth bin range.
4.2 Γ variation of a salt finger
ΓF has been widely used to distinguish salt fingers from turbulence, since its value is reported to be larger than the conventional ΓT value of 0.2 (St. Laurent and Schmitt, 1999). However, the full-depth observations presented in this study and previous ones indicate that 0.2 is an underestimate of ΓT, and the difference in the dissipation ratio between turbulent mixing and salt finger mixing in the deep water needs to be examined. Figure 10 presents the variations of and with depth. Compared with ΓT varying over 3 orders of magnitude, both and are less variable and change by 2 orders of magnitude or as little as 1 order. The median for all samples from the five projects is 0.47, slightly smaller than the ΓF observed in the diurnal thermocline of the Arabian Sea (0.65; Ashin et al., 2023), in the Kuroshio Extension Front (∼ 1; Nagai et al., 2015), and in the thermocline of the western tropical Atlantic (∼ 1.2; Schmitt et al., 2005). The median values for the five projects are distinct: 0.25, 0.29, and 0.28 for MIXET1, BBTRE96, and BBTRE97, similar to the conventional ΓT value of 0.2 as well as 0.52 for NATRE, distinguishable from 0.2 but close to the observed ΓT (Fig. 6), and 0.98 for MIXET2, significantly larger than 0.2 and different from the observed ΓT (Fig. 6). This suggests that the dissipation ratio difference between turbulence and salt fingers is complex.
Vertically, for MIXET1 stays nearly constant at 0.25, while for MIXET2 first decreases from 1 to 0.7 in the upper 700 m and then slightly increases to 2 at 1500 m. presents a similar vertical trend for both BBTRE96 and BBTRE97: is small and stays constant within the upper 800 m, with a median of 0.28; with depth increasing to 3000 m, it significantly increases over orders of magnitude, with the median value reaching ∼ 10. It is weakened at deeper depth. Note that the relatively small median for the BBTRE projects is mainly caused by the dominant patches with small values in the upper 800 m, and at depth is actually very large and significantly greater than 0.2 or the observed ΓT. The scenario for NATRE is similar to that for MIXET2, whose depth median remains nearly consistent around ∼ 0.5, although a very weak positive trend exists.
The “effective” salt dissipation ratio tends to be obviously larger than (Fig. 10). With the overall median of 1.87, the median values of for the five projects are 1.35 (MIXET1), 3.98 (MIXET2), 1.67 (BBTRE96), 1.71 (BBTRE97), and 1.83 (NATRE), floating around the value reported in the thermocline of the western tropical Atlantic of ∼ 2.8 (Schmitt et al., 2005). is strongly positively proportional to , with the median values of for the five projects being 5.1, 3.7, 6.3, 6.9, and 3.8, respectively. Thus, a general relation of 5 can be inferred. Due to this correlation, presents vary similar vertical variation as .
Note that is equivalent to (Eq. 6). Since Rρ is relatively easy to calculate, it is an alternate way to infer the hard-to-measure rF. Rρ and rF are the key parameters to estimate the dissipation ratios of heat and salt for a salt finger (Sect. 2.3). Therefore, many studies have tried to explore the relation of Rρ and rF based on theoretical derivations, laboratory experiments, and numerical simulations (Kelley, 1986; Kunze, 1987; Radko and Smith, 2012). Here, the Rρ–rF diagram colored by probability density for the five projects indicates that the salt finger patches are rather scattered (Fig. 11). However, the median rF binned by Rρ shows a clear nonmonotonic variability. For Rρ increasing from 1 to 2.4, rF decreases from ∼ 0.8 to 0.4; then, it gradually increases to 0.55 with Rρ approaching 3.7. This correlation between Rρ and rF can be well fitted by
Compared with other correlation curves (Kelley, 1986; Kunze, 1987; Radko and Smith, 2012), all of them present an rF decrease for Rρ smaller than 2, although the variation range and rate differ. The most obvious discrepancy between them is that rF tends to regain a larger value with Rρ exceeding 2.4 in our study, while all the other curves decrease little to asymptote to a constant value. The observational result presented here falls in the area outlined by the existing results. For our results, the salt finger patches with Rρ < 2.5 are abundant and mostly concentrated to indicate a negative correlation between Rρ and rF. It needs to be mentioned that patches with Rρ > 2.5 are much more rare and sparsely distributed, making the increase in rF in the larger Rρ range need to be treated carefully.
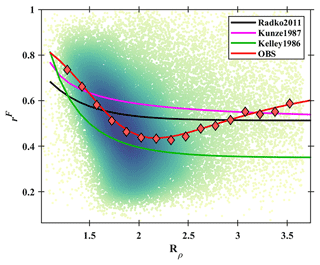
Figure 11Relation between Rρ and rF. Salt finger patches from all five projects are considered. Dots are colored by probability density, with darker color indicating larger probability density. The median rF values binned by Rρ are marked by red diamonds with a black edge, and the red curve is the fitting curve. The black, orange, and purple curves are adopted from Radko and Smith (2012), Kunze (1987), and Kelley (1986), respectively.
We also investigate the relation between observed and Reb (Fig. 12), which differs considerably between different projects. For MIXET1, a nearly linear decrease in (in logarithmic scale) from ∼ 1 to ∼ 0.1 can be easily observed for all patches with Reb between 0.3 and 25, indicating . for MIXET2 with Reb < 2.5 is also well fitted as , but for Reb > 2.5 tends to remain constant at 0.7. For the BBTRE projects, when Reb < 3, decreases at a larger rate than the MIXET projects, , and stays almost unchanged when Reb exceeds 3. for NATRE stays constant at 0.7 with most Reb values ranging from 1 to 25. Due to the strong correlation between and , the dependence of on Reb is similar to that of , although variation rates are different for some projects. Taking all the projects together, and decrease with Reb in general; however, the rate of decrease varies greatly with projects and different Reb bands, indicating that and may also be modulated by variables other than Reb.
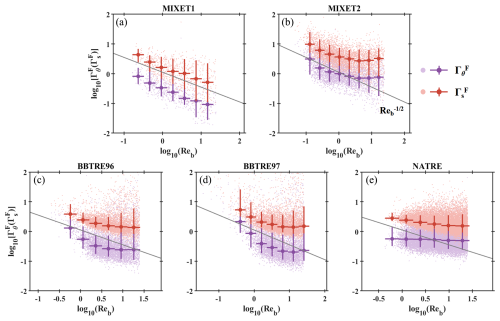
Figure 12Relations between ) and Reb for the five projects. Overlying the light-colored scatter of individual patches, the Reb-binned median values are marked by darker large dots. The bin size and 10th–90th percentile range are denoted by the horizontal and vertical bars, respectively. The gray line in each panel marks .
5.1 Eddy diffusivities induced by turbulence
Since ΓT deviates from the conventionally used constant of 0.2 in the Osborn relation, (also and ) based on ΓT differs from Kc based on 0.2 (Kc= 0.2) to different extents (Fig. 13). For MIXET1, since ΓT is only slightly larger than 0.2 in general, the magnitudes of and Kc differ slightly, with mean Kc= 2.1 × 10−6 m2 s−1 and mean 4.6 × 10−6 m2 s−1. Vertically, both and Kc decrease in the upper 1200 m and increase at deeper depth. Obvious differences between and Kc occur at depth ranges shallower than 1200 m and deeper than 2000 m, where the mean ratios of to Kc are 2.7 and 2.3, respectively. For MIXET2, the magnitude difference between and Kc is larger, with the mean values being 1.3 × 10−6 and 3.9 × 10−6 m2 s−1, respectively. Compared with Kc that stays nearly constant in the upper 1700 m, first decreases in the upper 700 m and then stays around 2 × 10−6 m2 s−1 between 700 and 1700 m. For BBTRE96, except for several depth bins, the difference between mean and mean Kc in the upper 3700 m is small, and they share similar downward increases and similar depth-averaged median values around 2.0 × 10−5 m2 s−1, with being about 2.5 times Kc. Although both increase at depths deeper than 3700 m, is nearly 4.7 times larger than Kc, and the mean values for and Kc are 5.0 × 10−4 and 1.1 × 10−4 m2 s−1, respectively. For BBTRE97, and Kc share the same downward negative trend and magnitude in the upper 1000 m, with mean values close to 2.6 × 10−5 m2 s−1. Beneath 1000 m, although sharing a similar positive trend, becomes larger and larger than Kc with depth. At depth between 1000 and 3700 m, 2.7 with median around 8.3 × 10−5 m2 s−1, while the corresponding values for depths deeper than 3700 m are 8.8 and mean 1.2 × 10−3 m2 s−1. For NATRE, is always larger than Kc at all depth ranges, and the mean values of and Kc are 4.4 × 10−5 and 1.0 × 10−5 m2 s−1, respectively. Vertically, Kc generally fluctuates around its mean value for the whole water column. also shows no clear vertical variation in the upper 2700 m, but it increases significantly from 2.6 × 10−5 m2 s−1 at 2700 m to 1.2 × 10−4 m2 s−1 at 3900 m. As a result, is 13.7 times larger than Kc at 3900 m. For the five projects, taking ΓT as a constant of 0.2 underestimates the actual eddy diffusivity induced by turbulence, and this underestimate may become more severe as ΓT increases with depth.
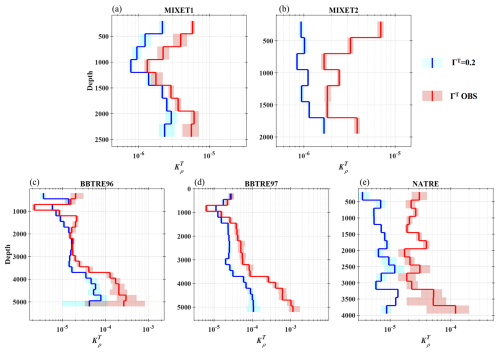
Figure 13Vertical profiles of depth bin mean ) based on energetic turbulence and weak turbulence patches for the five projects. The blue curve shows Kc estimates by using ΓT= 0.2, and the red curve shows based on the measured ΓT. The colored shading corresponds to 95 % bootstrapped confidence intervals. To exclude the influence of extreme values, we only consider patches with ΓT between the upper and lower quartiles for each depth bin. The depth bin size is 250 m.
5.2 Eddy diffusivities induced by salt fingers
For salt-finger-induced eddy diffusivities, some studies estimated their values by taking a constant rF of around 0.7 (0.75 in Schmitt et al., 2005; 0.6 in St. Laurent and Schmitt, 1999). Here, derived from the observed rF is compared with the rF= 0.7 estimate, (Fig. 14). Depending on the deviation of the observed rF from 0.7, the five projects are distinct in terms of the difference between and . For MIXET1, and both vary little with depth. But the magnitude of is significantly greater than that of , with mean values being 2.2 × 10−6 and 4.6 × 10−7 m2 s−1, respectively. This is in line with the fact that the mean value of the measured rF for MIXET1 is only 0.37, about one-half of 0.7. For MIXET2, with the median rF elevated to 0.63, is only slightly larger than . And they both increase with depth form O(10−6) m2 s−1 at 100 m to O(10−5) m2 s−1 at 1850 m. The median values of and are 4.4 × 10−6 and 3.2 × 10−6 m2 s−1, respectively. The difference between MIXET1 and MIXET2 indicates a strong seasonal variation of salt fingers in the tropical Pacific. For both BBTRE96 and BBTRE97, is significantly larger than in the upper layer with magnitudes around O(10−5) and O(10−6) m2 s−1, respectively, and this difference turns small as they both increase to 2 × 10−5 m2 s−1 with depth increasing to 2000 m. At deeper depths, although the salt finger disappears at some depth ranges, varies little around 2.5 × 10−5 m2 s−1. is generally larger than between 2400 and 3400 m with varying between 3 and 10, and this ratio drops to less than 2 for depths deeper than 3400 m. For NATRE, both and present clear downward increases, and is dominantly greater than . The difference between and is reduced with increasing depth due to the fact that increases much faster with depth from about 2 × 10−6 m2 s−1 in the upper 500 m to 1.5 × 10−5 m2 s−1 at 2400 m. For all the projects, is generally smaller than since rF is mostly smaller than 0.7, and this phenomenon is most obvious in the upper layer (upper 1000 m for BBTRE96, BBTRE97, and NATRE). At deeper depths, > can be observed in projects like BBTRE96 and BBTRE97. All of this indicates that rF is highly variable regionally and vertically. We also explore the vertical variation of , which is very similar to that of but with a larger magnitude (Fig. 15), as a result of being larger than and strongly proportional to .
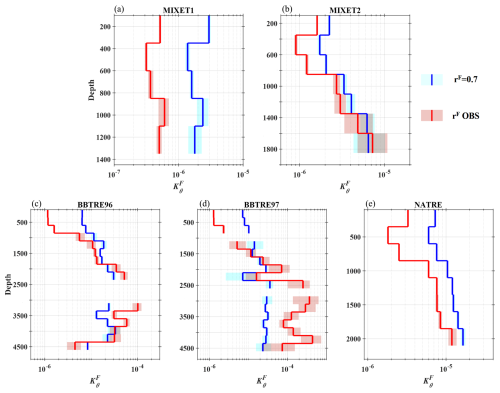
Figure 14Vertical profiles of depth bin mean ) based on salt finger patches for the five projects. The blue curves are estimated with rF= 0.7, and the red ones are based on the measured rF. The colored shading corresponds to 95 % bootstrapped confidence intervals. To exclude the influence of extreme values, we only consider patches with between the upper and lower quartiles for each depth bin. The depth bin size is 250 m, and depth bins with fewer than 10 patches are excluded.
Next, we examine the vertical variation of the ratio of to for the five projects (Fig. 16). For MIXET1, generally decreases from 5.3 in the upper 400 m to 4 at 1400 m, with an averaged value of 4.5. The averaged drops to 3.9 for MIXET2, and it varies between 3.7 and 4.5 except the small values shallower than 400 m and beneath 1600 m. The BBTRE projects share a similar vertical structure of : it has a maximum value of 6 in the upper 800 m, then sharply decreases to 2.5 at 1350 m and stays at this value until reaching 4600 m. for NATRE first increases from 3.0 to 4.7 in the upper 800 m and then sharply decreases to 3 at 1100 m and remains unchanged. From the five projects, generally increases with depth in the upper 1000 m with an average value about 5; then, it sharply drops to around 3 and stays at this value at deeper depths. This ratio is reported to be 2.3 in the western tropical Atlantic (Schmitt et al., 2005), slightly smaller than the result presented here. Van de Boog et al. (2021) presented a global map of and based on Argo data and an empirical method, and their results indicate that varies between 1.3 and 7.8 for Rρ ranging from 1 to 4. These earlier works do not show the vertical variation of due to indirect methods used.
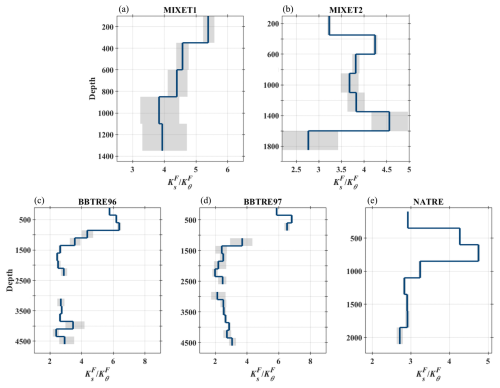
Figure 16Vertical profiles of depth bin mean based on salt finger patches for the five projects. The dark blue curves are the mean , and the gray shading represents 95 % bootstrapped confidence intervals. To exclude the influence of extreme values, we only consider patches with between the upper and lower quartiles for each depth bin. The depth bin size is 250 m, and depth bins with fewer than 10 patches are excluded.
5.3 “Total” eddy diffusivities under superposed salt fingers and turbulence
We examine the “total” eddy diffusivities contributed by both salt fingers and turbulence by combining the patches with weak turbulence, energetic turbulence, and salt fingers. Two different methods are used to estimate the total eddy diffusivities. The first is from McDougall and Ruddick (1992) (hereinafter MR92). MR92 does not need to differentiate salt finger and turbulent patches; it estimates the total eddy diffusivities by (i) evaluating the departure of observed Γ (Eq. 1) to a preset reasonable turbulent ΓT (e.g., ΓT= 0.265) and (ii) introducing a “salt flux enhancement factor”, M0, scaled by Rρ and r (more details are given in McDougall and Ruddick, 1992). Here, r is treated specifically depending on the mixing type – that is, rT=Rρ for turbulence and for salt fingers (St. Laurent and Schmitt, 1999). The second is from St. Laurent and Schmitt (1999) (hereinafter LS99), which differentiates turbulence and salt fingers first, then estimates their eddy diffusivities separately, and finally obtains the total ones as Kθ= and , where PT and PF are the number proportions of turbulence and salt finger patches to their sum, respectively. Figure 17 shows the total Kθ estimated by these two methods. Compared with BBTRE and NATRE, the results based on MR92 and LS99 present larger differences for MIXET, which may be due to fewer patches and more scattered ΓT and ΓF. Nonetheless, it is obvious that both estimates have a similar magnitude and vertical trend for all five projects. Comparing the total Kθ with and (Figs. 13, 14), we can see that Kθ, especially for the LS99 result, is obviously closer to for all five projects, confirming that turbulence dominates the observed microstructures. Note that Kθ values in the upper 500 m for BBTRE and NATRE are significantly lower than , seemingly indicating a strong weakening of Kθ due to the prevalence of salt fingers. However, the effect of a salt finger is actually overestimated, since the dominant hybrid mixing patches at this depth range are all excluded, which should be dominated by turbulence, as indicated by the elevated Reb. The total KS is not shown since it is very similar to the situation of Kθ, and the only notable difference is that KS is not significantly weakened by a salt finger in the upper 500 m for BBTRE and NATRE owing to being clearly greater than and much closer to (Fig. 15).
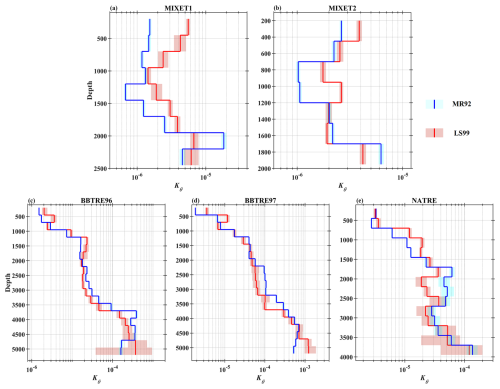
Figure 17Vertical profiles of depth-bin-averaged total Kθ based on turbulence and salt finger patches for the five projects. The blue curves are results based on MR92, and the red ones are based on LS99. The shading corresponds to 95 % bootstrapped confidence intervals. The depth bin size is 250 m, and depth bins with fewer than 10 patches are excluded.
The Osborn relation is widely used to estimate vertical eddy diffusivity in practice, assuming a constant dissipation ratio of ΓT= 0.2 without identifying the underlying mixing mechanisms. The dissipation ratios of heat, salinity, and density are equal for turbulent mixing; however, they differ for salt-finger-induced mixing. As a result, the eddy diffusivities derived from a constant dissipation ratio would inevitably depart from the actual values. In this study, we differentiated between turbulent mixing and salt finger mixing, quantified their dissipation ratios and eddy diffusivities, and examined their relations based on the datasets from the Microstructure Database.
We evaluated the variation of ΓT and its relations to Reb and ROT. The observed ΓT is scattered over orders of magnitude, typically ranging from 10−2 to 10. The significant difference between the five projects suggests that ΓT is highly variable with space and time. ΓT in the western equatorial Pacific presents a weak decrease downwards, while it obviously increases in the midlatitudes in the Atlantic. Although a negative relation between ΓT and Reb was supported by most of the projects, further investigation of the relations of ΓT to Reb and ROT suggested . This indicates that ΓT is modulated by more than one variable and explains why different relations between ΓT and Reb have been reported (e.g., Mashayek et al., 2017; Ijichi and Hibiya, 2018).
We compared estimated using observed ΓT with Kc estimated using ΓT= 0.2. is clearly larger than Kc. For the MIXET projects with downward weak decreasing ΓT, shares a similar vertical structure of Kc, with a magnitude elevated by about 2 or 3 times. For the rest of the projects where ΓT increases significantly with depth, generally presents a much more obvious increase than Kc, and can exceed Kc by an order of magnitude. This suggests that the intensity of bottom-enhanced mixing may be underestimated when assuming ΓT= 0.2.
For salt fingers, two effective dissipation ratios for heat () and salt () are derived, and two artificial Osborn relations are used to calculate the corresponding eddy diffusivities. spans about 2 orders of magnitude. Both the magnitude and vertical structure of are distinct for the five projects. is strongly related to , and they exhibit similar vertical structures, 5 . Data from some projects indicate a negative relation between () and Reb, while others suggest no clear relation. Unlike the existing results indicating that rF decreases and then asymptotes to a constant value with increasing Rρ, our results suggest that rF decreases sharply with Rρ when it is smaller than 2.4 and grows to a larger value with Rρ when it exceeds 2.4.
We examined salt-finger-induced and . Although a salt finger becomes rarer with depth, and increase clearly with depth, and is greater than . In the upper 1000 m, is significantly greater than by about 5 times for most projects, but below 1000 m, generally remains around 3. and estimated using the observed rF are generally smaller than those using rF= 0.7 due to most observed rF values being smaller than 0.7 but varying more sharply with depth.
Compared with eddy diffusivity induced by turbulence, is smaller than in the upper 1000 m, but they become increasingly comparable with depth. is close to or even larger than at all depths for all the projects. In general, although salt finger events are much rarer than turbulence at depth (so they are incapable of significantly altering the background mixing intensity shaped by turbulence), they can play a crucial role in local, short-period mixing events, which is worth investigating and properly parameterizing in numerical models.
The microstructure datasets used in this study are available at http://microstructure.ucsd.edu (Climate Process Team, 2016). The ETOPO 2022 bathymetry data used in Fig. 1 are available from NOAA at https://doi.org/10.25921/fd45-gt74 (NOAA, 2022).
The study was conceived and designed by all co-authors. Data preparation, material collection, and analysis were performed by JL. JL prepared the manuscript with contributions from all co-authors.
The contact author has declared that none of the authors has any competing interests.
Publisher’s note: Copernicus Publications remains neutral with regard to jurisdictional claims made in the text, published maps, institutional affiliations, or any other geographical representation in this paper. While Copernicus Publications makes every effort to include appropriate place names, the final responsibility lies with the authors.
We thank the Climate Process Team for publicly sharing the Microstructure Database, and we thank the two reviewers for their valuable feedback.
This research has been supported by the National Natural Science Foundation of China (grant nos. 42376012 and 42076012), the Postdoctoral Fellowship Program of CPSF (grant no. GZC20241610), the China Postdoctoral Science Foundation (grant no. 2024M753046), and the Fundamental Research Funds for the Central Universities (grant no. 202413031).
This paper was edited by Bernadette Sloyan and reviewed by two anonymous referees.
Ashin, K., Girishkumar, M. S., D'Asaro, E., Jofia, J., Sherin, V. R., Sureshkumar, N., and Rao, E. P. R.: Observational evidence of salt finger in the diurnal thermocline, Sci. Rep., 13, 3627, https://doi.org/10.1038/s41598-023-30564-5, 2023.
Barry, M. E., Ivey, G. N., Winters, K. B., and Imberger, J.: Measurements of diapycnal diffusivities in stratified fluids, J. Fluid Mech., 442, 267–291, https://doi.org/10.1017/S0022112001005080, 2001.
Cimoli, L., Caulfield, C. cille P., Johnson, H. L., Marshall, D. P., Mashayek, A., Naveira Garabato, A. C., and Vic, C.: Sensitivity of Deep Ocean Mixing to Local Internal Tide Breaking and Mixing Efficiency, Geophys. Res. Lett., 46, 14622–14633, https://doi.org/10.1029/2019GL085056, 2019.
Climate Process Team: Microstructure Database, Scripps Institution of Oceanography at University of California San Diego [data set], http://microstructure.ucsd.edu (last access: 11 April 2025), 2016.
Dillon, T. M.: Vertical overturns: A comparison of Thorpe and Ozmidov length scales, J. Geophys. Res., 87, 9601–9613, https://doi.org/10.1029/jc087ic12p09601, 1982.
Fine, E. C., MacKinnon, J. A., Alford, M. H., Middleton, L., Taylor, J., Mickett, J. B., Cole, S. T., Couto, N., Boyer, A. L., and Peacock, T.: Double Diffusion, Shear Instabilities, and Heat Impacts of a Pacific Summer Water Intrusion in the Beaufort Sea, J. Phys. Oceanogr., 52, 189–203, https://doi.org/10.1175/JPO-D-21-0074.1, 2022.
Gregg, M. C., Sanford, T. B., and Winkel, D. P.: Reduced mixing from the breaking of internal waves in equatorial waters, Nature, 422, 513–515, https://doi.org/10.1038/nature01507, 2003.
Gregg, M. C., D'Asaro, E. A., Riley, J. J., and Kunze, E.: Mixing efficiency in the ocean, Annu. Rev. Mar. Sci., 10, 443–473, https://doi.org/10.1146/annurev-marine-121916-063643, 2018.
Ijichi, T. and Hibiya, T.: Observed variations in turbulent mixing efficiency in the deep ocean, J. Phys. Oceanogr., 48, 1815–1830, https://doi.org/10.1175/JPO-D-17-0275.1, 2018.
Ijichi, T., St. Laurent, L., Polzin, K. L., and Toole, J. M.: How Variable Is Mixing Efficiency in the Abyss?, Geophys. Res. Lett., 47, e2019GL086813, https://doi.org/10.1029/2019GL086813, 2020.
Inoue, R., Yamazaki, H., Wolk, F., Kono, T., and Yoshida, J.: An Estimation of Buoyancy Flux for a Mixture of Turbulence and Double Diffusion, J. Phys. Oceanogr., 37, 611–624, https://doi.org/10.1175/JPO2996.1, 2007.
Jackson, L., Hallberg, R., and Legg, S.: A Parameterization of Shear-Driven Turbulence for Ocean Climate Models, J. Phys. Oceanogr., 38, 1033–1053, https://doi.org/10.1175/2007JPO3779.1, 2008.
Jackson, P. R. and Rehmann, C. R.: Laboratory Measurements of Differential Diffusion in a Diffusively Stable, Turbulent Flow, J. Phys. Oceanogr., 33, 1592–1603, https://doi.org/10.1175/2405.1, 2003.
Jayne, S. R.: The Impact of Abyssal Mixing Parameterizations in an Ocean General Circulation Model, J. Phys. Oceanogr., 39, 1756–1775, https://doi.org/10.1175/2009JPO4085.1, 2009.
Kelley, D.: Oceanic thermocline staircase, PhD thesis, Dalhousie University, Canada, 1986.
Klymak, J. M. and Legg, S. M.: A simple mixing scheme for models that resolve breaking internal waves, Ocean Model., 33, 224–234, https://doi.org/10.1016/j.ocemod.2010.02.005, 2010.
Kukulka, T., Law, K. L., and Proskurowski, G.: Evidence for the Influence of Surface Heat Fluxes on Turbulent Mixing of Microplastic Marine Debris, J. Phys. Oceanogr., 46, 809–815, https://doi.org/10.1175/JPO-D-15-0242.1, 2016.
Kunze, E.: Limits on growing, finite length salt fingers: a Richardson number constraint, J. Mar. Res., 45, 533–556, 1987.
Li, J., Yang, Q., Sun, H., Zhang, S., Xie, L., Wang, Q., Zhao, W., and Tian, J.: On the Variation of Dissipation Flux Coefficient in the Upper South China Sea, J. Phys. Oceanogr., 53, 551–571, https://doi.org/10.1175/JPO-D-22-0127.1, 2023.
MacKinnon, J. A. and Gregg, M. C.: Mixing on the Late-Summer New England Shelf – Solibores, Shear, and Stratification, J. Phys. Oceanogr., 33, 1476–1492, https://doi.org/10.1175/1520-0485(2003)033<1476:MOTLNE>2.0.CO;2, 2003.
MacKinnon, J. A., Zhao, Z., Whalen, C. B., Waterhouse, A. F., Trossman, D. S., Sun, O. M., Laurent, L. C. S., Simmons, H. L., Polzin, K., Pinkel, R., Pickering, A., Norton, N. J., Nash, J. D., Musgrave, R., Merchant, L. M., Melet, A. V., Mater, B., Legg, S., Large, W. G., Kunze, E., Klymak, J. M., Jochum, M., Jayne, S. R., Hallberg, R. W., Griffies, S. M., Diggs, S., Danabasoglu, G., Chassignet, E. P., Buijsman, M. C., Bryan, F. O., Briegleb, B. P., Barna, A., Arbic, B. K., Ansong, J. K., and Alford, M. H.: Climate Process Team on Internal Wave–Driven Ocean Mixing, B. Am. Meteorol. Soc., 98, 2429–2454, https://doi.org/10.1175/BAMS-D-16-0030.1, 2017.
Mashayek, A., Salehipour, H., Bouffard, D., Caulfield, C. P., Ferrari, R., Nikurashin, M., Peltier, W. R., and Smyth, W. D.: Efficiency of turbulent mixing in the abyssal ocean circulation, Geophys. Res. Lett., 44, 6296–6306, https://doi.org/10.1002/2016GL072452, 2017.
Mater, B. D., Venayagamoorthy, S. K., Laurent, L. S., and Moum, J. N.: Biases in thorpe-scale estimates of turbulence dissipation. Part I: Assessments from large-scale overturns in oceanographic data, J. Phys. Oceanogr., 45, 2497–2521, https://doi.org/10.1175/JPO-D-14-0128.1, 2015.
McDougall, T. J.: Some Implications of Ocean Mixing for Ocean Modell., in: Elsevier Oceanography Series, vol. 46, edited by: Nihoul, J. C. J. and Jamart, B. M., Elsevier, 21–35, https://doi.org/10.1016/S0422-9894(08)70535-X, 1988.
McDougall, T. J. and Ruddick, B. R.: The use of ocean microstructure to quantify both turbulent mixing and salt-fingering, Deep-Sea Res., 39, 1931–1952, https://doi.org/10.1016/0198-0149(92)90006-F, 1992.
Monismith, S. G., Koseff, J. R., and White, B. L.: Mixing Efficiency in the Presence of Stratification: When Is It Constant?, Geophys. Res. Lett., 45, 5627–5634, https://doi.org/10.1029/2018GL077229, 2018.
Moum, J. N.: Efficiency of mixing in the main thermocline, J. Geophys. Res., 101, 12057–12069, https://doi.org/10.1029/96JC00508, 1996.
Nagai, T., Inoue, R., Tandon, A., and Yamazaki, H.: Evidence of enhanced double-diffusive convection below the main stream of the Kuroshio Extension, J. Geophys. Res., 120, 8402–8421, https://doi.org/10.1002/2015JC011288, 2015.
NOAA: National Centers for Environmental Information: ETOPO 2022 15 Arc-Second Global Relief Model, NOAA National Centers for Environmental Information [data set], https://doi.org/10.25921/fd45-gt74, 2022.
Oakey, N. S.: Statistics of Mixing Parameters in the Upper Ocean During JASIN Phase 2, J. Phys. Oceanogr., 15, 1662–1675, https://doi.org/10.1175/1520-0485(1985)015<1662:SOMPIT>2.0.CO;2, 1985.
Osborn, T. R.: Estimates of the Local Rate of Vertical Diffusion from Dissipation Measurements, J. Phys. Oceanogr., 10, 83–89, https://doi.org/10.1175/1520-0485(1980)010<0083:EOTLRO>2.0.CO;2, 1980.
Osborn, T. R. and Cox, C. S.: Oceanic fine structure, Geophys. Fluid Dyn., 3, 321–345, https://doi.org/10.1080/03091927208236085, 1972.
Oyabu, R., Yasuda, I., and Sasaki, Y.: Large-Scale Distribution and Variations of Active Salt-Finger Double-Diffusion in the Western North Pacific, J. Phys. Oceanogr., 53, 2013–2027, https://doi.org/10.1175/JPO-D-22-0244.1, 2023.
Polzin, K. and Ferrari, R.: Isopycnal Dispersion in NATRE, J. Phys. Oceanogr., 34, 247–257, https://doi.org/10.1175/1520-0485(2004)034<0247:IDIN>2.0.CO;2, 2004.
Polzin, K. L., Toole, J. M., Ledwell, J. R., and Schmitt, R. W.: Spatial Variability of Turbulent Mixing in the Abyssal Ocean, Science, 276, 93–96, https://doi.org/10.1126/science.276.5309.93, 1997.
Pujiana, K., Moum, J. N., and Smyth, W. D.: The Role of Turbulence in Redistributing Upper-Ocean Heat, Freshwater, and Momentum in Response to the MJO in the Equatorial Indian Ocean, J. Phys. Oceanogr., 48, 197–220, https://doi.org/10.1175/JPO-D-17-0146.1, 2018.
Radko, T. and Smith, D. P.: Equilibrium transport in double-diffusive convection, J. Fluid Mech., 692, 5–27, https://doi.org/10.1017/jfm.2011.343, 2012.
Richards, K. J., Natarov, A., Firing, E., Kashino, Y., Soares, S. M., Ishizu, M., Carter, G. S., Lee, J. H., and Chang, K. I.: Shear-generated turbulence in the equatorial Pacific produced by small vertical scale flow features, J. Geophys. Res., 120, 3777–3791, https://doi.org/10.1002/2014JC010673, 2015.
Ruddick, B.: A practical indicator of the stability of the water column to double-diffusive activity, Deep-Sea Res., 30, 1105–1107, https://doi.org/10.1016/0198-0149(83)90063-8, 1983.
Sabine, C. L., Feely, R. A., Gruber, N., Key, R. M., Lee, K., Bullister, J. L., Wanninkhof, R., Wong, C. S., Wallace, D. W. R., Tilbrook, B., Millero, F. J., Peng, T.-H., Kozyr, A., Ono, T., and Rios, A. F.: The Oceanic Sink for Anthropogenic CO2, Science, 305, 367–371, https://doi.org/10.1126/science.1097403, 2004.
Salehipour, H., Caulfield, C. P., and Peltier, W. R.: Turbulent mixing due to the Holmboe wave instability at high Reynolds number, J. Fluid Mech., 803, 591–621, https://doi.org/10.1017/jfm.2016.488, 2016.
Schmitt, R. W.: Double Diffusion in Oceanography, Annu. Rev. Fluid Mech., 26, 255–285, https://doi.org/10.1146/annurev.fl.26.010194.001351, 1994.
Schmitt, R. W., Ledwell, J. R., Montgomery, E. T., Polzin, K. L., and Toole, J. M.: Enhanced Diapycnal Mixing by Salt Fingers in the Thermocline of the Tropical Atlantic, Science, 308, 685–688, https://doi.org/10.1126/science.1108678, 2005.
Shih, L. H., Koseff, J. R., Ivey, G. N., and Ferziger, J. H.: Parameterization of turbulent fluxes and scales using homogeneous sheared stably stratified turbulence simulations, J. Fluid Mech., 525, 193–214, https://doi.org/10.1017/S0022112004002587, 2005.
Smyth, W. D., Moum, J. N., and Caldwell, D. R.: The efficiency of mixing in turbulent patches: Inferences from direct simulations and microstructure observations, J. Phys. Oceanogr., 31, 1969–1992, https://doi.org/10.1175/1520-0485(2001)031<1969:teomit>2.0.co;2, 2001.
St. Laurent, L. and Schmitt, R. W.: The contribution of salt fingers to vertical mixing in the North Atlantic Tracer Release Experiment, J. Phys. Oceanogr., 29, 1404–1424, https://doi.org/10.1175/1520-0485(1999)029<1404:tcosft>2.0.co;2, 1999.
St. Laurent, L., Garabato, A. C. N., Ledwell, J. R., Thurnherr, A. M., Toole, J. M., and Watson, A. J.: Turbulence and Diapycnal Mixing in Drake Passage, J. Phys. Oceanogr., 42, 2143–2152, https://doi.org/10.1175/JPO-D-12-027.1, 2012.
van der Boog, C. G., Dijkstra, H. A., Pietrzak, J. D., and Katsman, C. A.: Double-diffusive mixing makes a small contribution to the global ocean circulation, Commun. Earth Environ., 2, 1–9, https://doi.org/10.1038/s43247-021-00113-x, 2021.
Vladoiu, A., Bouruet-Aubertot, P., Cuypers, Y., Ferron, B., Schroeder, K., Borghini, M., and Leizour, S.: Contrasted mixing efficiency in energetic versus quiescent regions: Insights from microstructure measurements in the Western Mediterranean Sea, Prog. Oceanogr., 195, 102594, https://doi.org/10.1016/j.pocean.2021.102594, 2021.
Waterhouse, A. F., MacKinnon, J. A., Nash, J. D., Alford, M. H., Kunze, E., Simmons, H. L., Polzin, K. L., Laurent, L. C. S., Sun, O. M., Pinkel, R., Talley, L. D., Whalen, C. B., Huussen, T. N., Carter, G. S., Fer, I., Waterman, S., Garabato, A. C. N., Sanford, T. B., and Lee, C. M.: Global Patterns of Diapycnal Mixing from Measurements of the Turbulent Dissipation Rate, J. Phys. Oceanogr., 44, 1854–1872, https://doi.org/10.1175/JPO-D-13-0104.1, 2014.
Whitt, D. B., Lévy, M., and Taylor, J. R.: Low-frequency and high-frequency oscillatory winds synergistically enhance nutrient entrainment and phytoplankton at fronts, J. Geophys. Res., 122, 1016–1041, https://doi.org/10.1002/2016JC012400, 2017.
Wunsch, C. and Ferrari, R.: Vertical mixing, energy, and the general circulation of the oceans, Annu. Rev. Fluid Mech., 36, 281–314, https://doi.org/10.1146/annurev.fluid.36.050802.122121, 2004.
- Abstract
- Introduction
- Data and methods
- Statical features of turbulent mixing and salt finger mixing
- Γ variation of turbulence and salt fingers
- Eddy diffusivities induced by turbulence and salt fingers
- Summary
- Data availability
- Author contributions
- Competing interests
- Disclaimer
- Acknowledgements
- Financial support
- Review statement
- References
- Abstract
- Introduction
- Data and methods
- Statical features of turbulent mixing and salt finger mixing
- Γ variation of turbulence and salt fingers
- Eddy diffusivities induced by turbulence and salt fingers
- Summary
- Data availability
- Author contributions
- Competing interests
- Disclaimer
- Acknowledgements
- Financial support
- Review statement
- References